Perceived individual fairness with a molecular representation for medicine recommendations
Knowledge-Based Systems(2022)
摘要
Medicine recommendations, as important research fields of artificial intelligence, could provide patients with treatments. However, existing medicine recommendation algorithms aim to maximize the overall accuracy while ignoring individual fairness. It is of great importance to ensure that medicine recommendation algorithms fairly treat every patient. To ensure individual fairness, we propose individual fairness-based medicine recommendation systems (IFMR). In fairness scenario, similar patients can get similar performance of recommendation. Specifically, we first adopt dual-pipeline gate recurrent units to capture the health condition with electrical health records. IFMR then utilizes the molecular graph encoder and molecular substructure encoder to capture medicine features at the molecular level. Finally, IFMR maintains the spatial distance of similar patients by utilizing multi-similarity individual fairness loss. Extensive experiments on benchmark datasets demonstrate that IFMR is more effective and fairer than all strong baselines.
更多查看译文
关键词
Fair recommendation,Individual fairness,Recommendation systems,Medicine recommendations
AI 理解论文
溯源树
样例
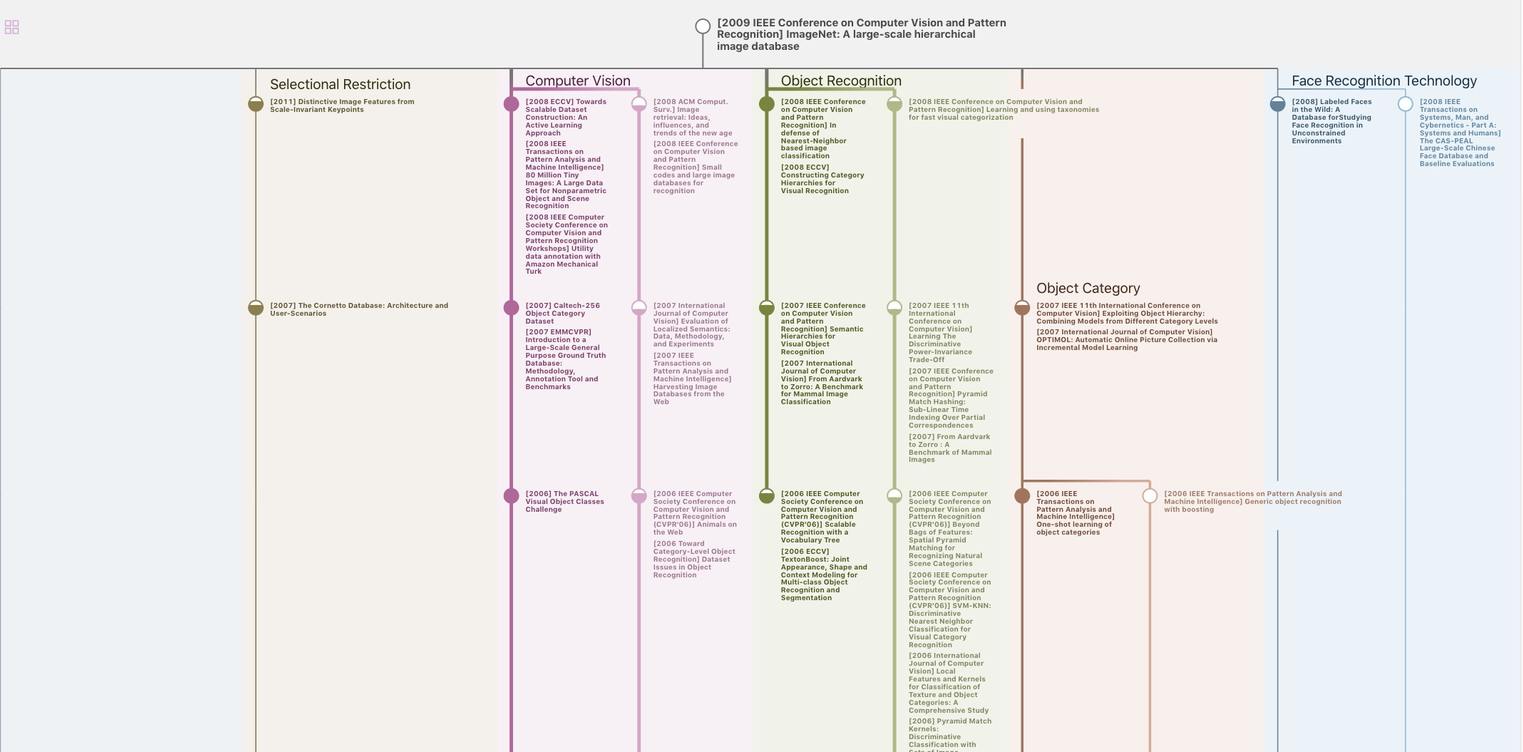
生成溯源树,研究论文发展脉络
Chat Paper
正在生成论文摘要