An End-to-end Heterogeneous Restraint Network for RGB-D Cross-modal Person Re-identification
ACM TRANSACTIONS ON MULTIMEDIA COMPUTING COMMUNICATIONS AND APPLICATIONS(2022)
摘要
The RGB-D cross-modal person re-identification (re-id) task aims to identify the person of interest across the RGB and depth image modes. The tremendous discrepancy between these two modalities makes this task difficult to tackle. Few researchers pay attention to this task, and the deep networks of existing methods still cannot be trained in an end-to-end manner. Therefore, this article proposes an end-to-end module for RGBD cross-modal person re-id. This network introduces a cross-modal relational branch to narrow the gaps between two heterogeneous images. It models the abundant correlations between any cross-modal sample pairs, which are constrained by heterogeneous interactive learning. The proposed network also exploits a dual-modal local branch, which aims to capture the common spatial contexts in two modalities. This branch adopts shared attentive pooling and mutual contextual graph networks to extract the spatial attention within each local region and the spatial relations between distinct local parts, respectively. Experimental results on two public benchmark datasets, that is, the BIWI and RobotPKU datasets, demonstrate that our method is superior to the state-of-the-art. In addition, we perform thorough experiments to prove the effectiveness of each component in the proposed method.
更多查看译文
关键词
RGB-D cross-modal person re-identification,end-to-end deep network,heterogeneous interactive learning,cross-modal relational branch,mutual contextual graph networks
AI 理解论文
溯源树
样例
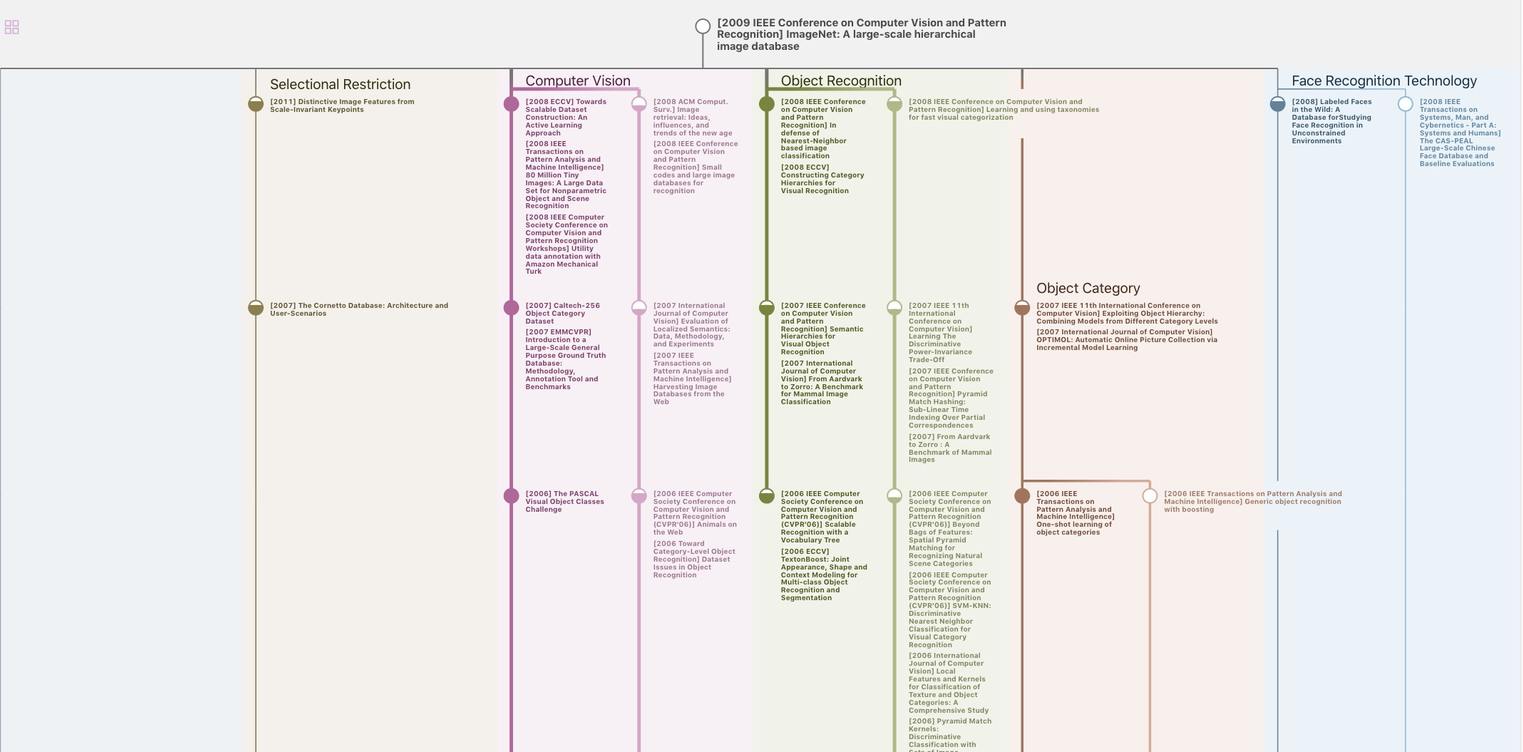
生成溯源树,研究论文发展脉络
Chat Paper
正在生成论文摘要