A hierarchical kriging approach for multi-fidelity optimization of automotive crashworthiness problems
Structural and Multidisciplinary Optimization(2022)
摘要
Multi-fidelity optimization schemes enriching expensive high-fidelity functions with cheap-to-evaluate low-fidelity functions have gained popularity in recent years. In the present work, an optimization scheme based on a hierarchical kriging is proposed for large-scale and highly non-linear crashworthiness problems. After comparison to other multi-fidelity techniques an infill criterion called variable-fidelity expected improvement is applied and evaluated. This is complemented by two innovative techniques, a new approach regarding initial sampling and a novel way to generate the low-fidelity model for crash problems are suggested. For the former, a modified Latin hypercube sampling, pushing samples more towards design space boundaries, increases the quality of sampling selection. For the latter, a projection-based non-intrusive model order reduction technique accelerates and simplifies the low-fidelity model evaluation. The proposed techniques are investigated with two application problems from the field of automotive crashworthiness—a size optimization problem for lateral impact and a shape optimization problem for frontal impact. The use of a multi-fidelity scheme compared to baseline single-fidelity optimization saves computational effort while keeping an acceptable level of accuracy. Both suggested modifications, independently and especially combined, increase computational performance and result quality in the presented examples.
更多查看译文
关键词
Kriging,Efficient global optimization,Multi-fidelity optimization,Crashworthiness,Model order reduction,Isovolumetric Latin hypercube
AI 理解论文
溯源树
样例
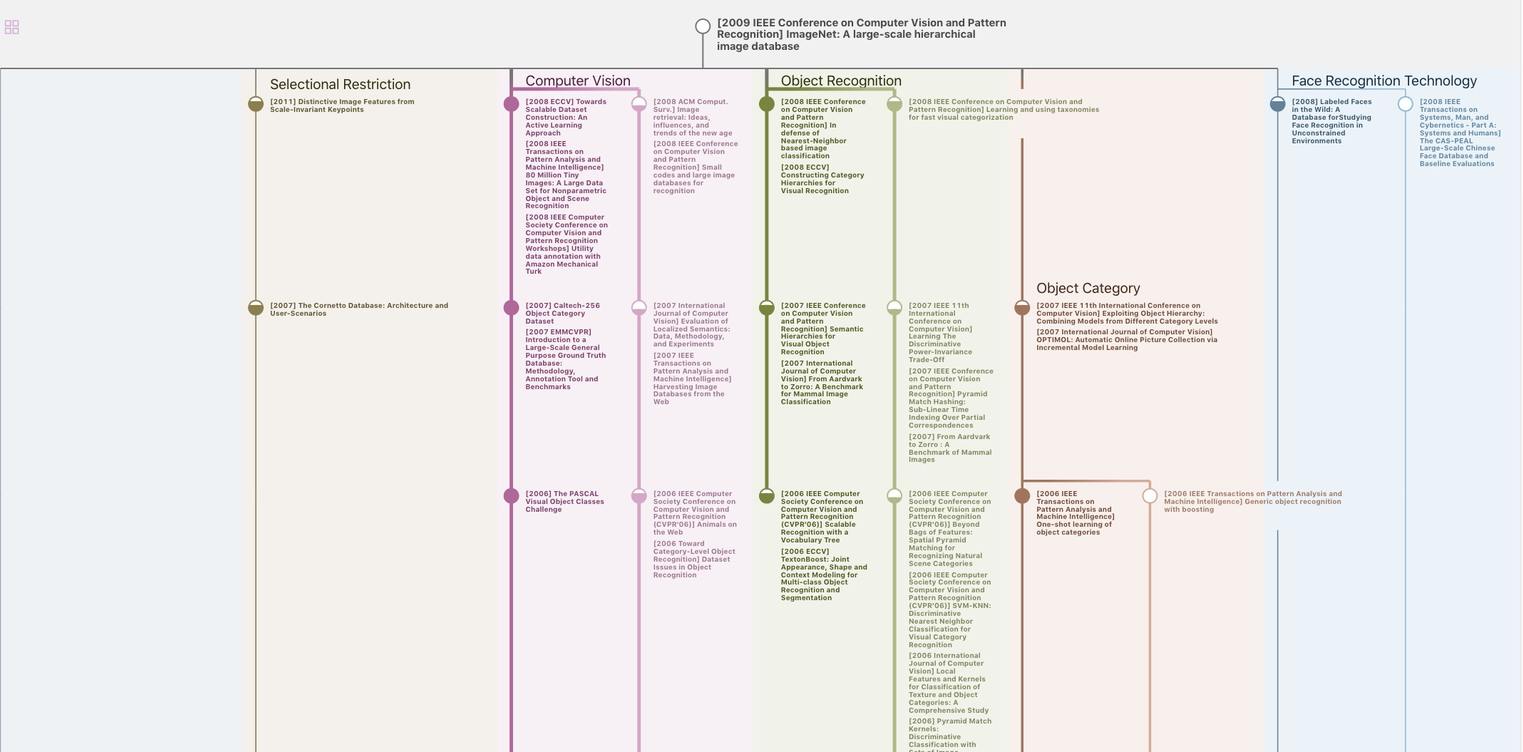
生成溯源树,研究论文发展脉络
Chat Paper
正在生成论文摘要