Regional People Counting Approach Based on Multi-Motion States Models Using MIMO Radar
IEEE GEOSCIENCE AND REMOTE SENSING LETTERS(2022)
摘要
The existing radar-based regional people counting methods usually perform a single prediction model to classify the number of people. However, signals of different motion states of the target vary greatly, which results in some features aliasing among different numbers of people. It is difficult to find an optimal single prediction model to adapt the different motion states of the target, especially in the case of limited training data. To address this problem, we propose a regional people counting approach based on multi-motion states models using multi-input multioutput (MIMO) radar. We first use the state classification model with the 2-D spatial attribute features to classify the motion state of the target and then cascade the corresponding motion state model to classify the number of people. In particular, we incorporate the mixed state into the proposed approach to deal with the situations of mixed motion states of targets. The experimental results show that the proposed approach can be implemented in an embedded operating system, and its average classification accuracy of zero person, one person, and multiple persons is above 97.45% and much better than that of the existing regional people counting methods in the metal ramp with strong static clutter and strong multipath, which is a small room and too small for many people.
更多查看译文
关键词
Predictive models,Clutter,Radar,Indexes,Feature extraction,Chirp,MIMO radar,Metal ramp,multi-input multioutput (MIMO) radar,multi-motion states models (MMSMs),regional people counting,spatial attribute features
AI 理解论文
溯源树
样例
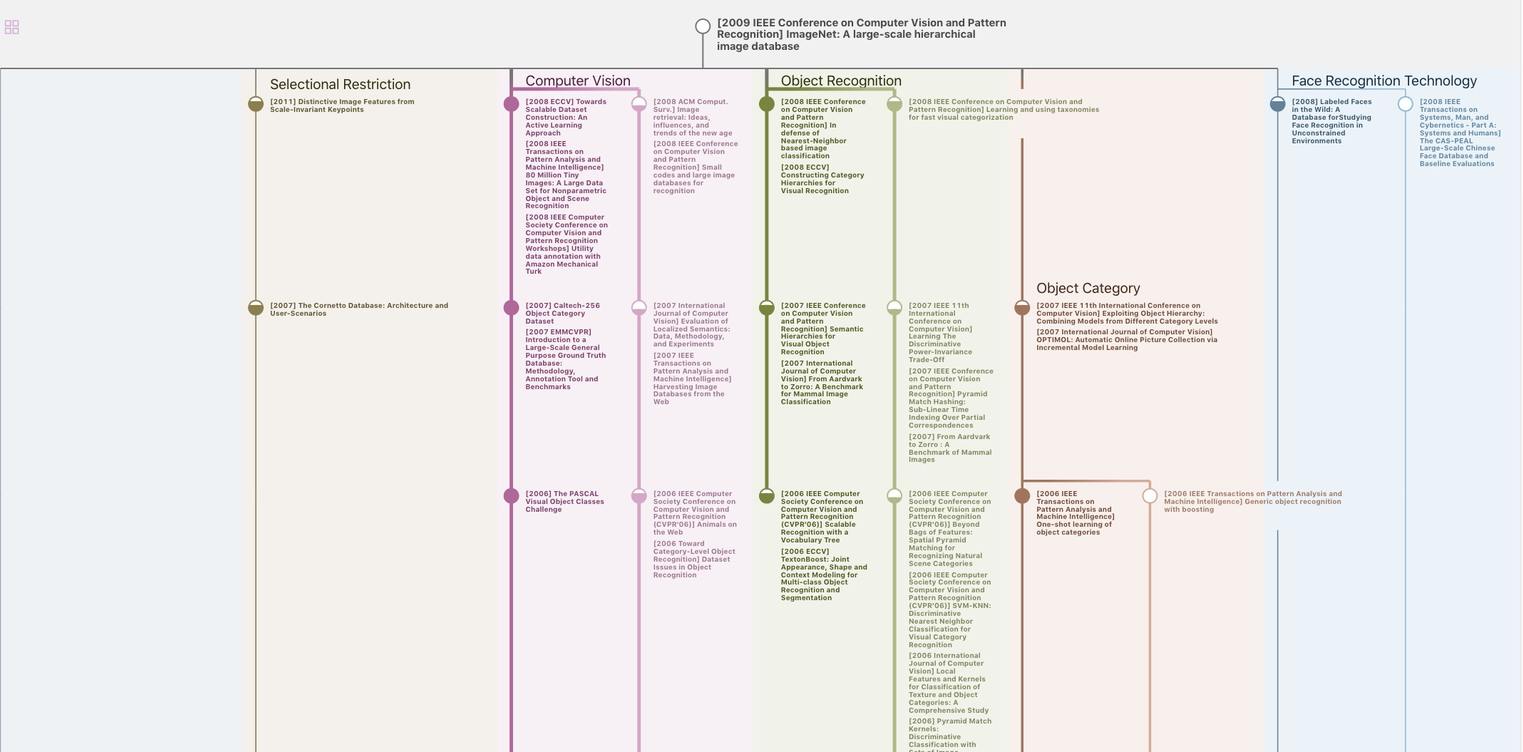
生成溯源树,研究论文发展脉络
Chat Paper
正在生成论文摘要