Evading behavioral classifiers: a comprehensive analysis on evading ransomware detection techniques
Neural Computing and Applications(2022)
摘要
Recent progress in machine learning has led to promising results in behavioral malware detection. Behavioral modeling identifies malicious processes via features derived by their runtime behavior. Behavioral features hold great promise as they are intrinsically related to the functioning of each malware, and are therefore considered difficult to evade. Indeed, while a significant amount of results exists on evasion of static malware features, evasion of dynamic features has seen limited work. This paper examines the robustness of behavioral ransomware detectors to evasion and proposes multiple novel techniques to evade them. Ransomware behavior differs significantly from that of benign processes, making it an ideal best case for behavioral detectors, and a difficult candidate for evasion. We identify and propose a set of novel attacks that distribute the overall malware workload across a small set of independent, cooperating processes in order to avoid the generation of significant behavioral features. Our most effective attack decreases the accuracy of a state-of-the-art classifier from 98.6 to 0% using only 18 cooperating processes. Furthermore, we show our attacks to be effective against commercial ransomware detectors in a black-box setting. Finally, we evaluate a detector designed to identify our most effective attack, as well as discuss potential directions to mitigate our most advanced attack.
更多查看译文
关键词
Ransomware, Machine learning, Behavioral detection, Evasion
AI 理解论文
溯源树
样例
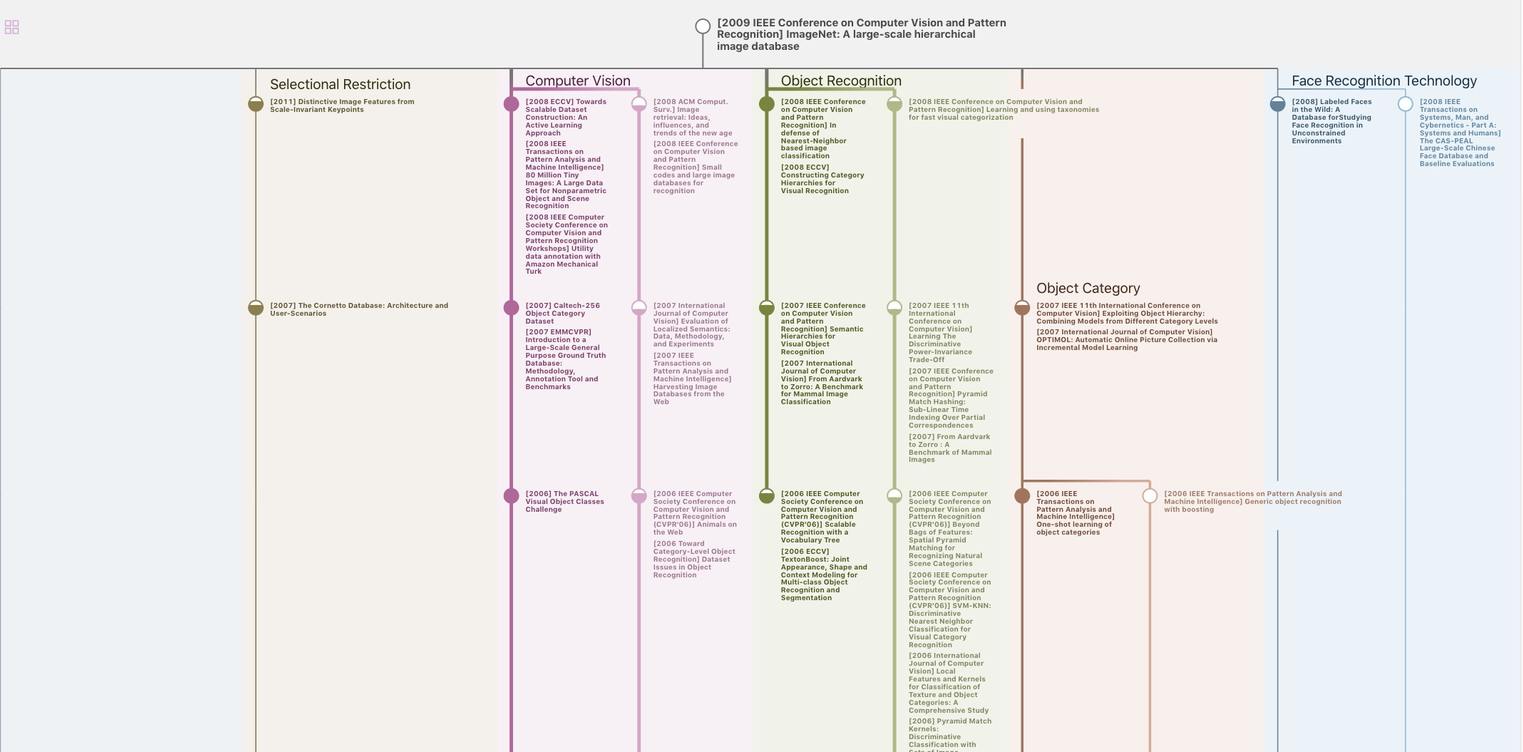
生成溯源树,研究论文发展脉络
Chat Paper
正在生成论文摘要