Machine Learning-based Wearable Bio-processor for Real-Time Blood Pressure Estimation
ICEIC(2022)
摘要
Recently, as continuous monitoring of blood pressure (BP) becomes important for the treatment and management of hypertension, which is a representative chronic disease, many studies on non-invasive cuff-less BP have been conducted. Due to accuracy degradation of the simple linear algorithms, complex nonlinear algorithms are preferred, but its latency and energy efficiency are suffered from the limited CPU computational capability on wearable devices. In this paper, we present a wearable bio-processor that is capable of real-time BP estimation based on Electrocardiogram (ECG) and Photoplethysmography (PPG), which can provide high BP estimation accuracy with a nonlinear regression machine learning model. The proposed bio-processor including Arm Cortex-M0 and dedicated hardware accelerators runs at 50MHz and is prototyped with a Xilinx Artix-7 FPGA. It shows the root mean square error (RMSE) of 6.04 mmHg and 5.88 mmHg for Systolic BP and Diastolic BP, respectively.
更多查看译文
关键词
Blood Pressure, BP Estimation, Bio-processor, Machine Learning, SoC (System-on-Chip)
AI 理解论文
溯源树
样例
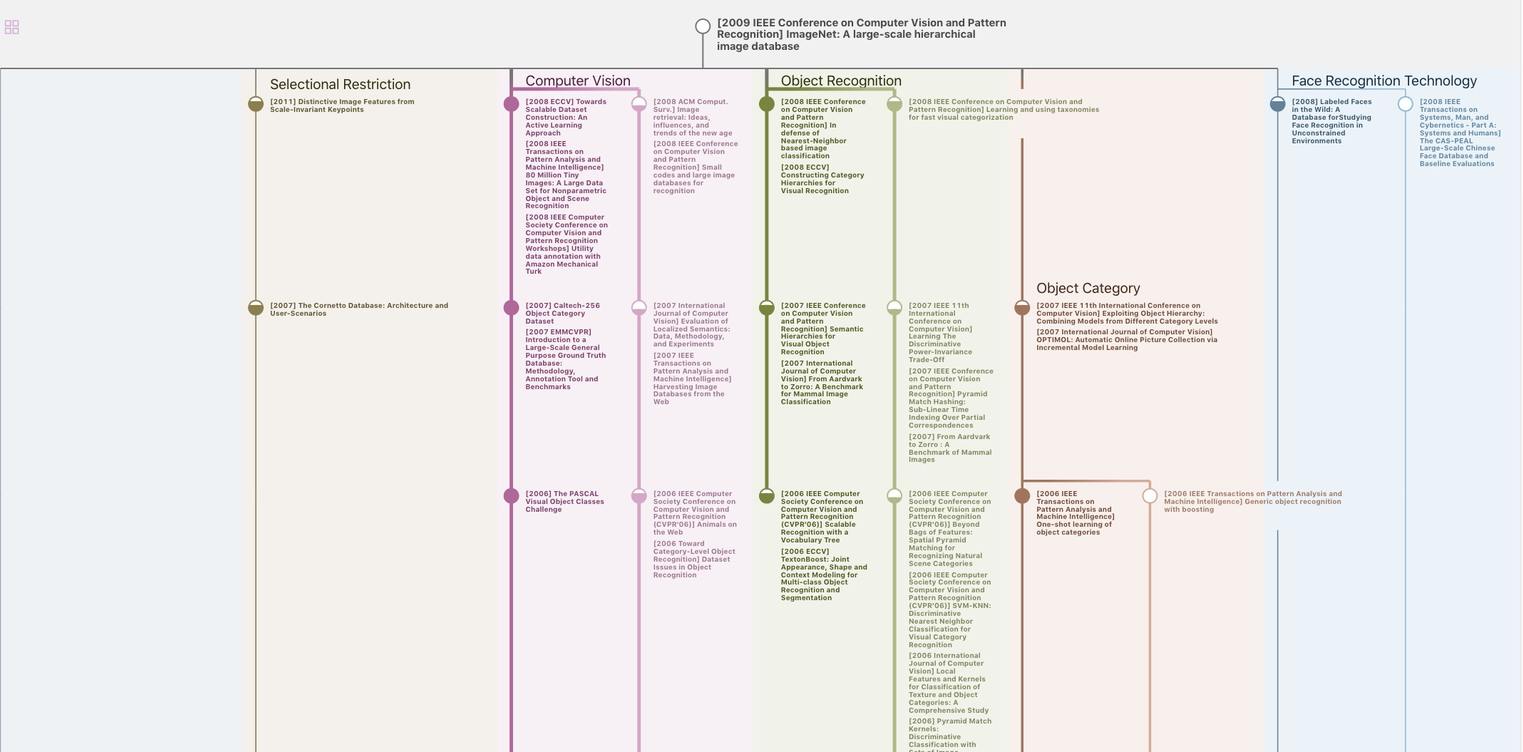
生成溯源树,研究论文发展脉络
Chat Paper
正在生成论文摘要