Multi-viewport based 3D convolutional neural network for 360-degree video quality assessment
Multimedia Tools and Applications(2022)
摘要
360-degree videos, also known as omnidirectional or panoramic videos, provide the user an immersive experience that 2D videos cannot provide. It is crucial to access the perceived quality of the 360-degree video. 2D video quality assessment (VQA) methods are unsuitable for 360-degree videos. There are few 360-degree video quality assessment (360VQA) methods. This paper proposes a multi-viewport based 3D convolutional neural network for 360VQA (3D-360VQA). First, it is easy to divide the 2D planar video into rectangular blocks as video patches in order to adapt to a deep neural network. The way to form the video patch in a 2D planar video is unsuitable for a 360-degree video. Thus, a multiple viewports based video patch forming method is proposed. Second, although the deep neural networks have achieved great success in image quality assessment (IQA), there are few deep neural networks for 360VQA. A 3D convolution based deep neural network is proposed to predict the perceived quality of 360-degree videos. The publicly available 360-degree videos datasets are used to evaluate the proposed method. The experimental results show that the proposed method is suitable for the 360-degree video and outperforms other existing methods, which verifies the effectiveness of our network architecture.
更多查看译文
关键词
Deep learning, Video quality assessment (VQA), 360-degree video, Convolutional neural network (CNN), Virtual reality (VR)
AI 理解论文
溯源树
样例
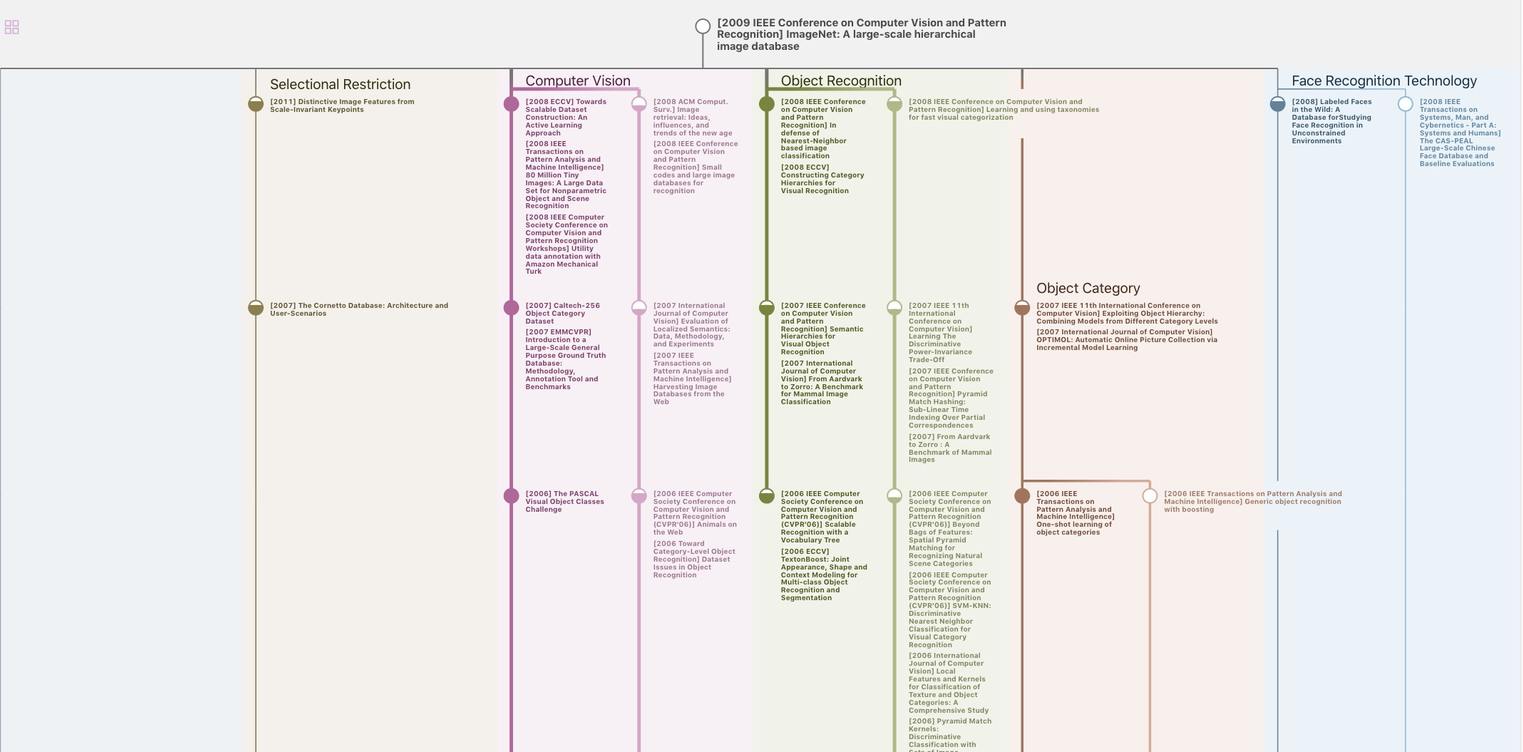
生成溯源树,研究论文发展脉络
Chat Paper
正在生成论文摘要