A feature selection method with feature ranking using genetic programming
CONNECTION SCIENCE(2022)
摘要
Feature selection is a data processing method which aims to select effective feature subsets from original features. Feature selection based on evolutionary computation (EC) algorithms can often achieve better classification performance because of their global search ability. However, feature selection methods using EC cannot get rid of invalid features effectively. A small number of invalid features still exist till the termination of the algorithms. In this paper, a feature selection method using genetic programming (GP) combined with feature ranking (FRFS) is proposed. It is assumed that the more the original features appear in the GP individuals' terminal nodes, the more valuable these features are. To further decrease the number of selected features, FRFS using a multi-criteria fitness function which is named as MFRFS is investigated. Experiments on 15 datasets show that FRFS can obtain higher classification performance with smaller number of features compared with the feature selection method without feature ranking. MFRFS further reduces the number of features while maintaining the classification performance compared with FRFS. Comparisons with five benchmark techniques show that MFRFS can achieve better classification performance.
更多查看译文
关键词
feature selection,feature ranking,genetic programming,multi-criteria
AI 理解论文
溯源树
样例
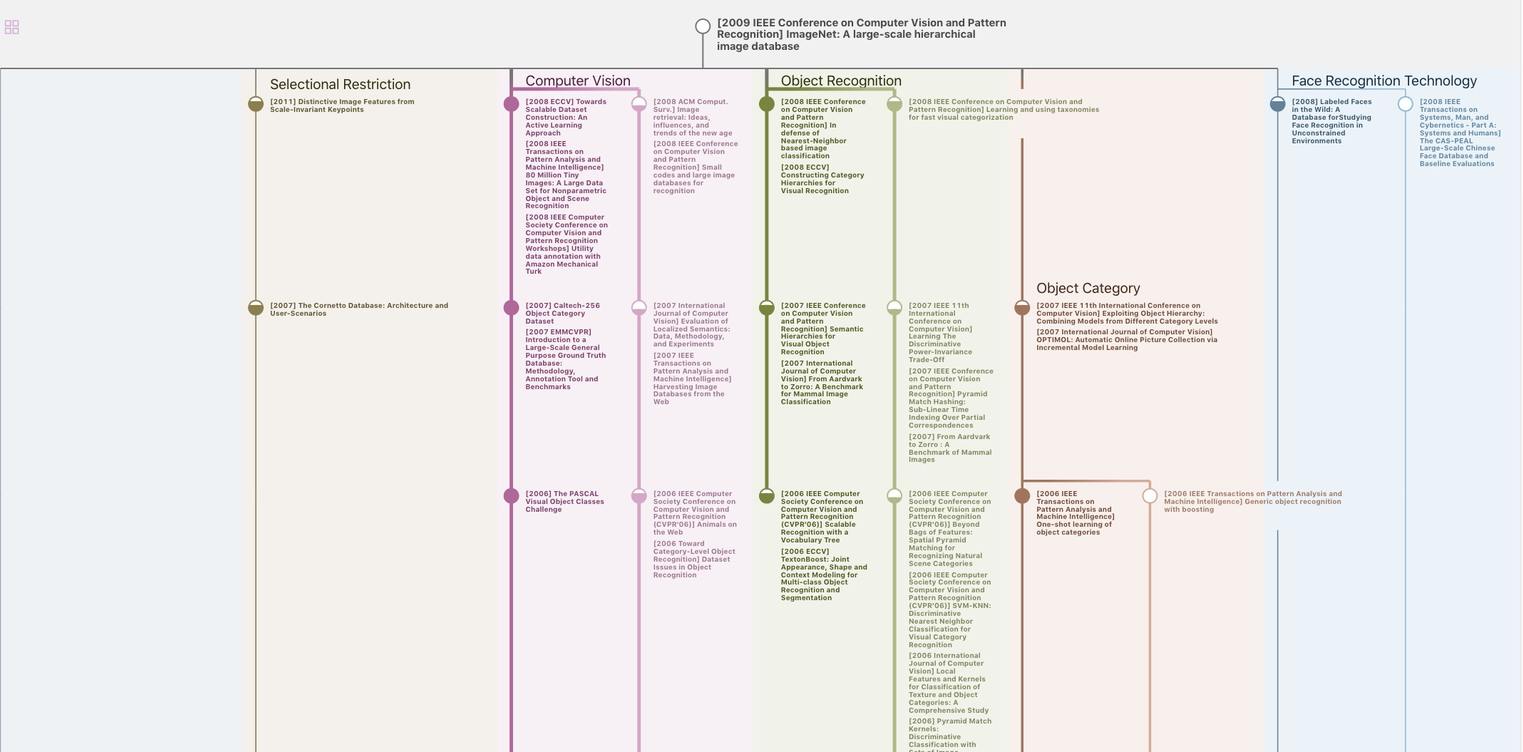
生成溯源树,研究论文发展脉络
Chat Paper
正在生成论文摘要