A Deep Learning Model for Inferring Elevated Pulmonary Capillary Wedge Pressures From the 12-Lead Electrocardiogram
JACC: Advances(2022)
摘要
Noninvasive measurement of patient hemodynamics remains an important goal in cardiovascular medicine. In this study, we developed a deep learning model that can infer elevated mean pulmonary capillary wedge pressure (mPCWP) from the 12-lead electrocardiogram. The model, Right Heart Catheterization Network (RHCNet), also identifies those predictions that are likely to correspond to misleading results, thereby helping clinical providers gauge when model predictions are untrustworthy. Our method may form the foundation for an effective tool for ruling out elevated left-sided filling pressures in selected patients. RHCNet is generally available at https://github.com/daphneschles/RHCnet and may noninvasively screen for an elevated mPCWP when invasive hemodynamic monitoring cannot be routinely performed.
更多查看译文
关键词
deep learning,ECG,pulmonary artery occlusion pressure,pulmonary artery wedge pressure,pulmonary capillary wedge pressure
AI 理解论文
溯源树
样例
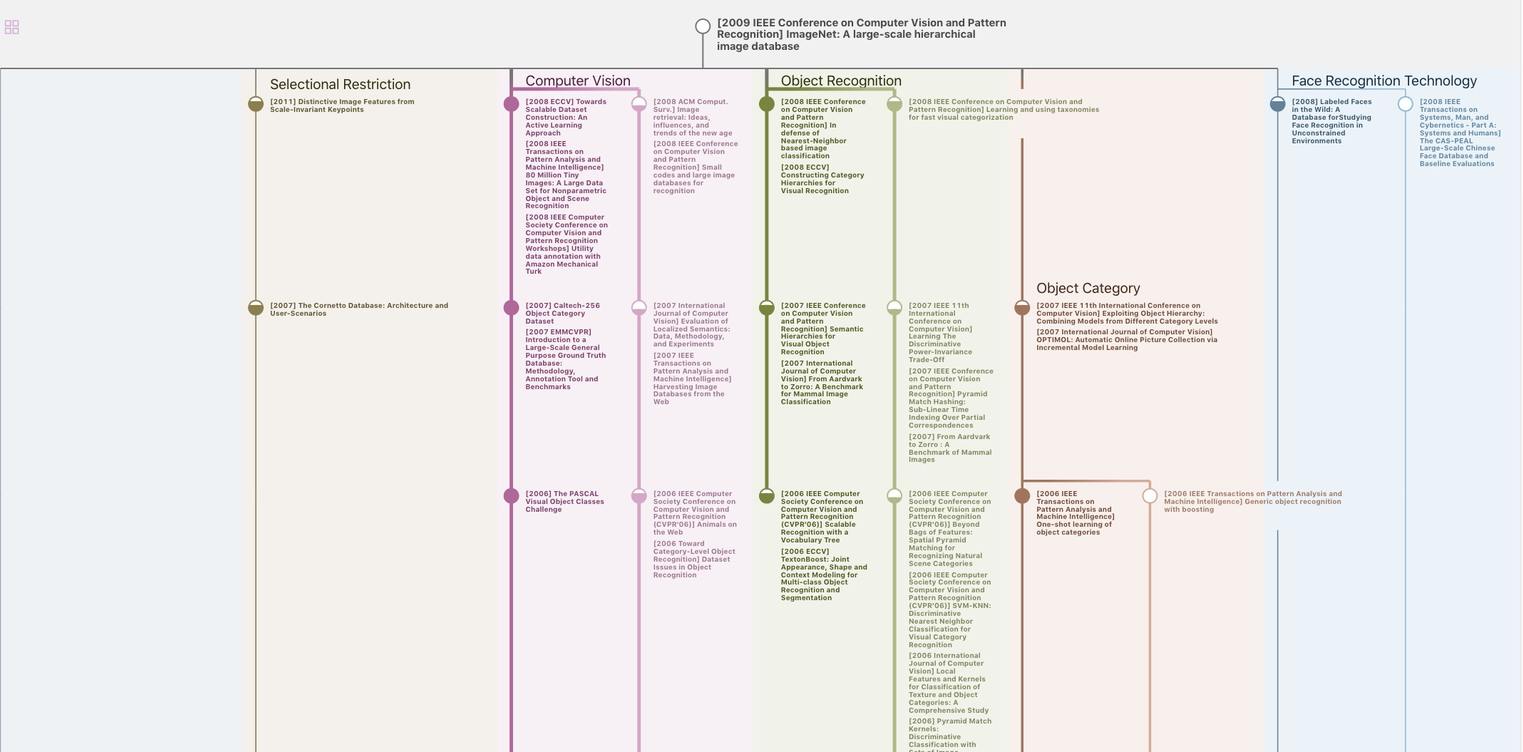
生成溯源树,研究论文发展脉络
Chat Paper
正在生成论文摘要