NR-CNN: Nested-Residual Guided CNN In-loop Filtering for Video Coding
ACM TRANSACTIONS ON MULTIMEDIA COMPUTING COMMUNICATIONS AND APPLICATIONS(2022)
摘要
Recently, deep learning for video coding, such as deep predictive coding, deep transform coding, and deep in-loop filtering, has been an emerging research area. The coding gain of hybrid coding framework could be extensively promoted by the data-driven models. However, previous deep coding tools especially deep in-loop filtering mainly consider the performance improvement while pay less attention to the reliability, usability, and adaptivity of the networks. In this article, a nested-residual guided convolutional neural network (NR-CNN) structure with cascaded global shortcut and configurable residual blocks is proposed for in-loop filtering. By taking advantage of the correlation between different color components, we further extend the NR-CNN by utilizing luminance as textural and structural guidance for chrominance filtering, which significantly improves the filtering performance. To fully exploit the proposed network into codec integration, we subsequently introduce an efficient and adaptive framework consisting of an adaptive granularity optimization and a parallel inference pipeline for deep learning based filtering. The former contributes to the coding performance improvement through an adaptive decision-making based on rate-distortion analysis at various granularities. The latter reduces the running time of network inference. The extensive experimental results show the superiority of the proposed method, achieving 8.2%, 14.9%, and 13.2% BD-rate savings on average under random access (RA) configuration. Meanwhile, the proposed method also obtains better subjective quality.
更多查看译文
关键词
In-loop filter,deep learning based video coding,rate and distortion optimization,video coding
AI 理解论文
溯源树
样例
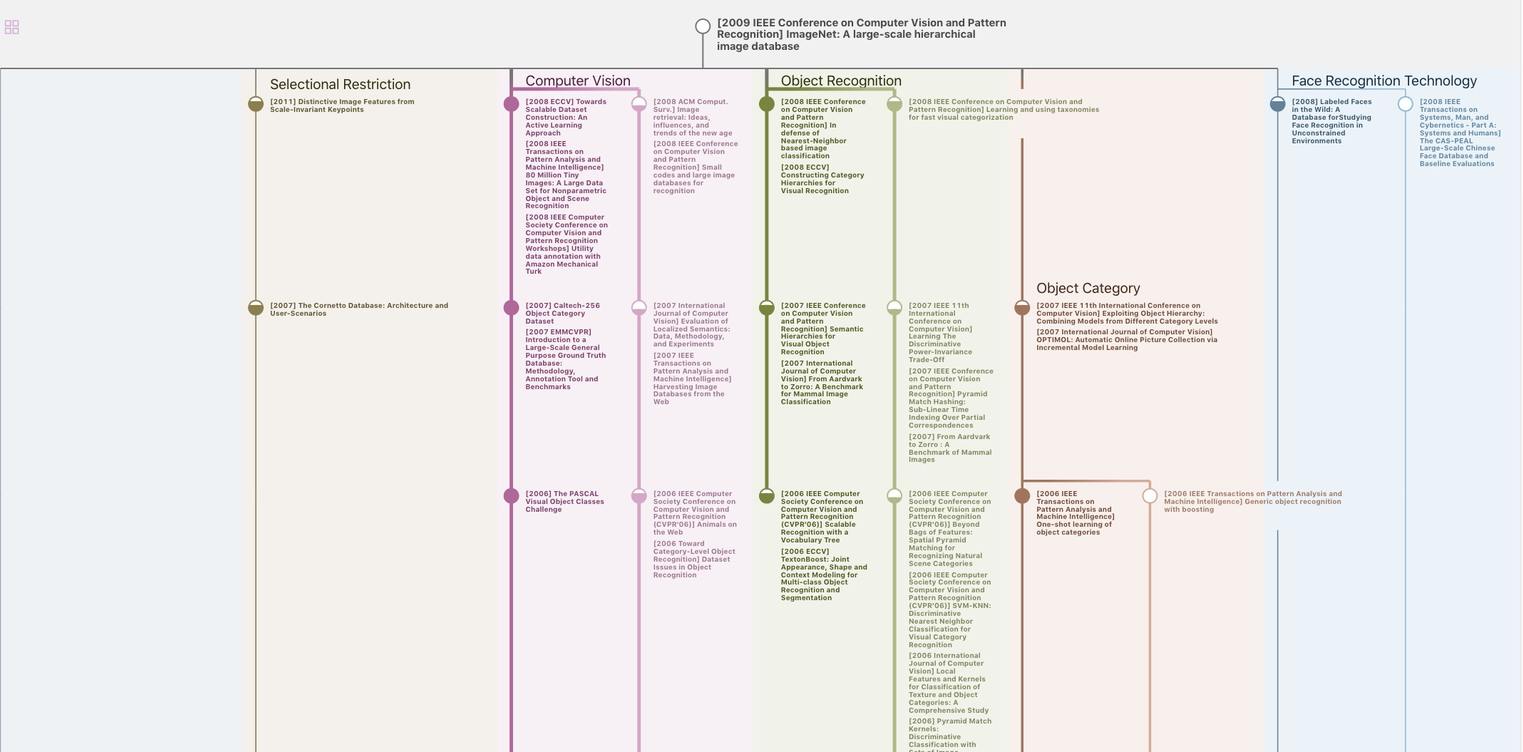
生成溯源树,研究论文发展脉络
Chat Paper
正在生成论文摘要