A Continual Deepfake Detection Benchmark: Dataset, Methods, and Essentials
WACV(2023)
摘要
There have been emerging a number of benchmarks and techniques for the detection of deepfakes. However, very few works study the detection of incrementally appearing deepfakes in the real-world scenarios. To simulate the wild scenes, this paper suggests a continual deepfake detection benchmark (CDDB) over a new collection of deepfakes from both known and unknown generative models. The suggested CDDB designs multiple evaluations on the detection over easy, hard, and long sequence of deepfake tasks, with a set of appropriate measures. In addition, we exploit multiple approaches to adapt multiclass incremental learning methods, commonly used in the continual visual recognition, to the continual deepfake detection problem. We evaluate existing methods, including their adapted ones, on the proposed CDDB. Within the proposed benchmark, we explore some commonly known essentials of standard continual learning. Our study provides new insights on these essentials in the context of continual deepfake detection. The suggested CDDB is clearly more challenging than the existing benchmarks, which thus offers a suitable evaluation avenue to the future research. Both data and code are available at https://github.com/Coral79/CDDB.
更多查看译文
关键词
continual deepfake detection benchmark
AI 理解论文
溯源树
样例
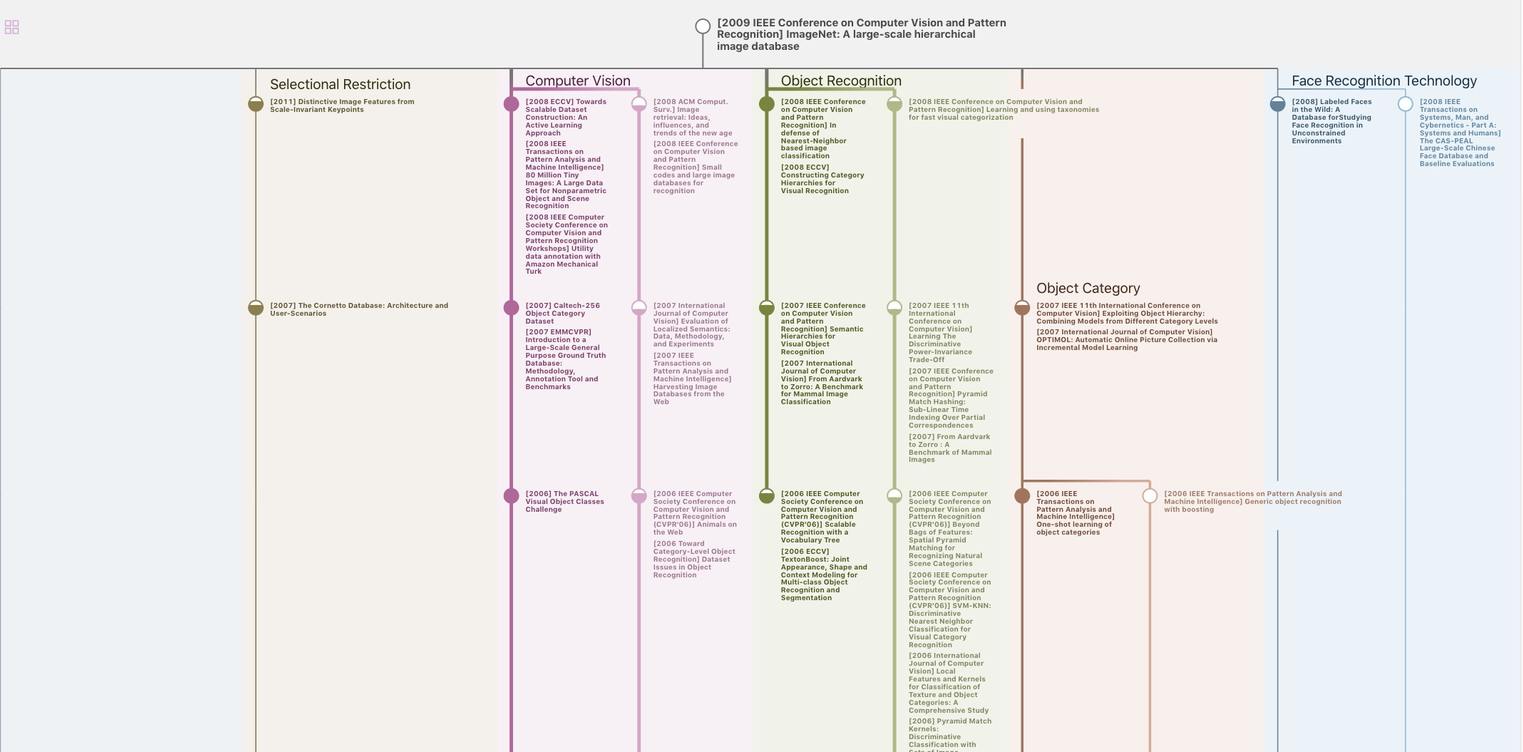
生成溯源树,研究论文发展脉络
Chat Paper
正在生成论文摘要