Deep Learning-based Channel Estimation for Wideband Hybrid MmWave Massive MIMO
arxiv(2022)
摘要
Hybrid analog-digital (HAD) architecture is widely adopted in practical millimeter wave (mmWave) massive multiple-input multiple-output (MIMO) systems to reduce hardware cost and energy consumption. However, channel estimation in the context of HAD is challenging due to only limited radio frequency (RF) chains at transceivers. Although various compressive sensing (CS) algorithms have been developed to solve this problem by exploiting inherent channel sparsity and sparsity structures, practical effects, such as power leakage and beam squint, can still make the real channel features deviate from the assumed models and result in performance degradation. Also, the high complexity of CS algorithms caused by a large number of iterations hinders their applications in practice. To tackle these issues, we develop a deep learning (DL)-based channel estimation approach where the sparse Bayesian learning (SBL) algorithm is unfolded into a deep neural network (DNN). In each SBL layer, Gaussian variance parameters of the sparse angular domain channel are updated by a tailored DNN, which is able to effectively capture complicated channel sparsity structures in various domains. Besides, the measurement matrix is jointly optimized for performance improvement. Then, the proposed approach is extended to the multi-block case where channel correlation in time is further exploited to adaptively predict the measurement matrix and facilitate the update of Gaussian variance parameters. Based on simulation results, the proposed approaches significantly outperform existing approaches but with reduced complexity.
更多查看译文
关键词
Millimeter wave, massive MIMO, hybrid analog-digital, wideband channel estimation, measurement matrix, power leakage, beam squint, sparse Bayesian learning, model-driven deep learning
AI 理解论文
溯源树
样例
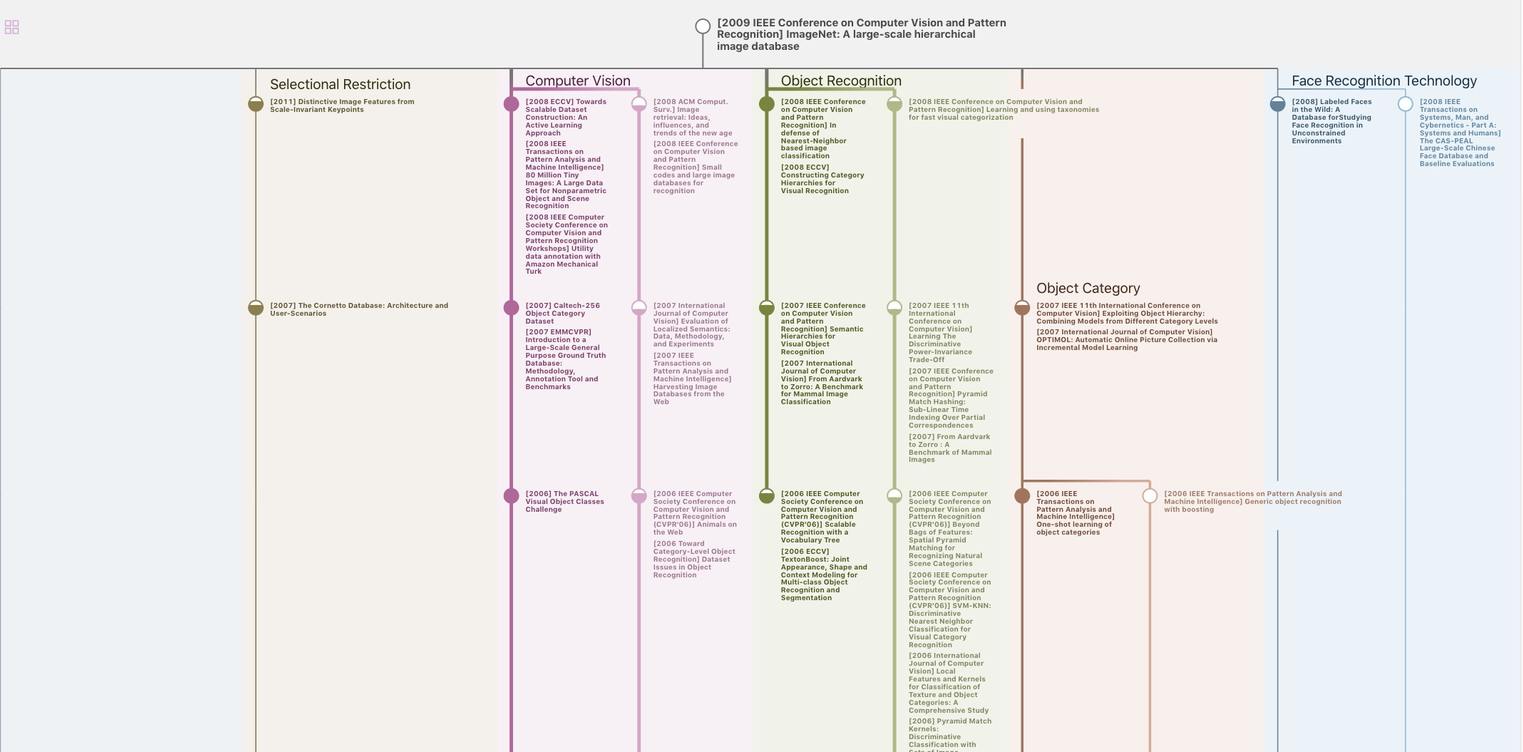
生成溯源树,研究论文发展脉络
Chat Paper
正在生成论文摘要