Deep graph clustering via mutual information maximization and mixture model
Knowledge and Information Systems(2024)
摘要
Attributed graph clustering or community detection which learns to cluster the nodes of a graph is a challenging task in graph analysis. Recently contrastive learning has shown significant results in various unsupervised graph learning tasks. In spite of the success of graph contrastive learning methods in self-supervised graph learning, using them for graph clustering is not well explored. In this paper, we introduce a contrastive learning framework for learning clustering-friendly node embedding. We propose Gaussian mixture information maximization which utilizes a mutual information maximization approach for node embedding. Meanwhile, in order to have a clustering-friendly embedding space, it imposes a mixture of Gaussians distribution on this space. The parameters of the contrastive node embedding model and the mixture distribution are optimized jointly in a unified framework. Experiments show that our clustering-directed embedding space can enhance clustering performance in comparison with the case where community structure of the graph is ignored during node representation learning. The results on real-world datasets demonstrate the effectiveness of our method in community detection.
更多查看译文
关键词
Graph neural network,Graph representation learning,Graph clustering,Contrastive learning
AI 理解论文
溯源树
样例
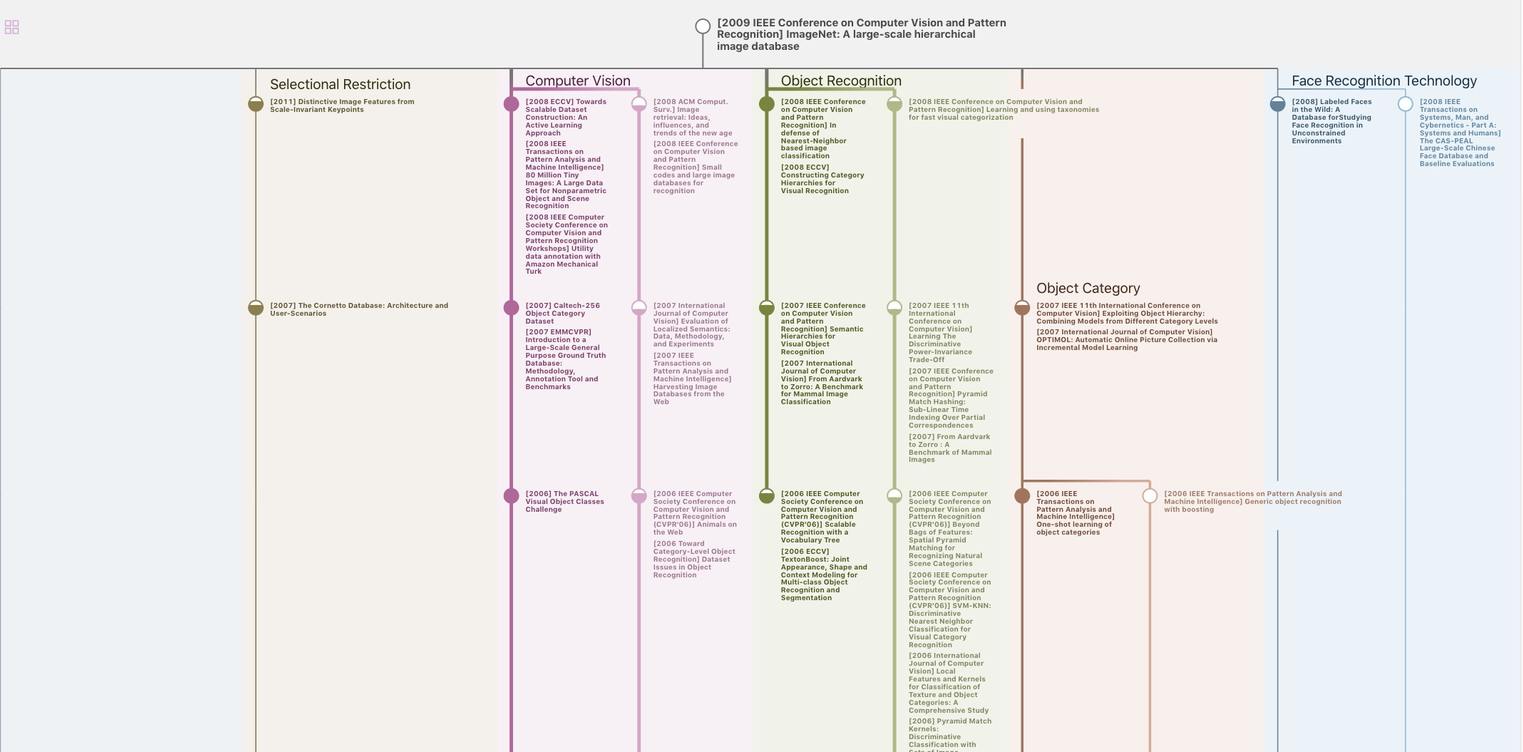
生成溯源树,研究论文发展脉络
Chat Paper
正在生成论文摘要