Machine Learning for Chemical Reactivity: The Importance of Failed Experiments
ANGEWANDTE CHEMIE-INTERNATIONAL EDITION(2022)
摘要
Assessing the outcomes of chemical reactions in a quantitative fashion has been a cornerstone across all synthetic disciplines. Classically approached through empirical optimization, data-driven modelling bears an enormous potential to streamline this process. However, such predictive models require significant quantities of high-quality data, the availability of which is limited: Main reasons for this include experimental errors and, importantly, human biases regarding experiment selection and result reporting. In a series of case studies, we investigate the impact of these biases for drawing general conclusions from chemical reaction data, revealing the utmost importance of "negative" examples. Eventually, case studies into data expansion approaches showcase directions to circumvent these limitations-and demonstrate perspectives towards a long-term data quality enhancement in chemistry.
更多查看译文
关键词
Cross-Coupling, Data Bias, Machine Learning, Reaction Data, Yield Prediction
AI 理解论文
溯源树
样例
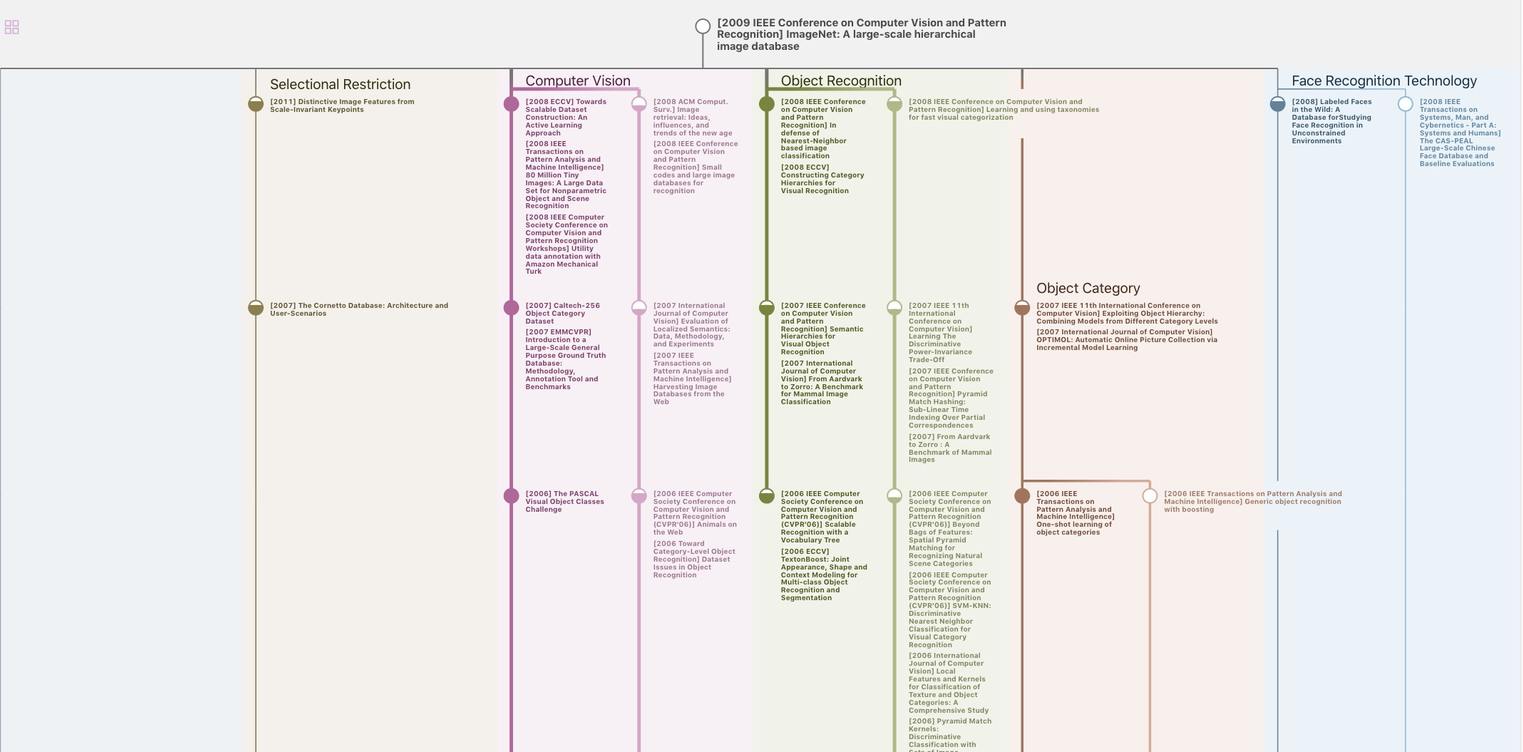
生成溯源树,研究论文发展脉络
Chat Paper
正在生成论文摘要