Intrusion detection in wireless sensor network using enhanced empirical based component analysis
FUTURE GENERATION COMPUTER SYSTEMS-THE INTERNATIONAL JOURNAL OF ESCIENCE(2022)
摘要
Nowadays, several kinds of attacks exist in cyberspace, and hence comprehensive research has been implemented to overcome these drawbacks. One such method to provide security in WSN (Wireless sensor network) is Intrusion Detection System. However, the determination of unknown attacks remains a major challenge in the intrusion detection system. Hence, the usage of deep learning methodologies remains to be an active area in cyber security. However, prevailing a deep learning algorithm possesses limitations such as comparatively low accuracy and heavily depends on the manual selection of the features. These problems have been analyzed, and corresponding to the problems, the present study proposed an enhanced empirical-based component analysis to select relevant features. This proposed feature selection model integrates the advantages of both empirical mode decomposition and principal component analysis to retain most of the relevant features. The classification of the attack node with the selected features was performed with LSTM (Long Short Term Memory). The proposed framework validated datasets, namely NSL-KDD, CICIDS 2017, UNSW NB 2015, and KDD99 datasets, compared with the state of art methods. The comparative analysis with the prevailing methods proved the effectiveness of the presented system in terms of performance metrics such as accuracy, F1 score, Recall, FPR, FAR, etc. (c) 2022 Elsevier B.V. All rights reserved.
更多查看译文
关键词
Intrusion detection,Wireless sensor networks,LSTM,Empirical mode decomposition,Component analysis
AI 理解论文
溯源树
样例
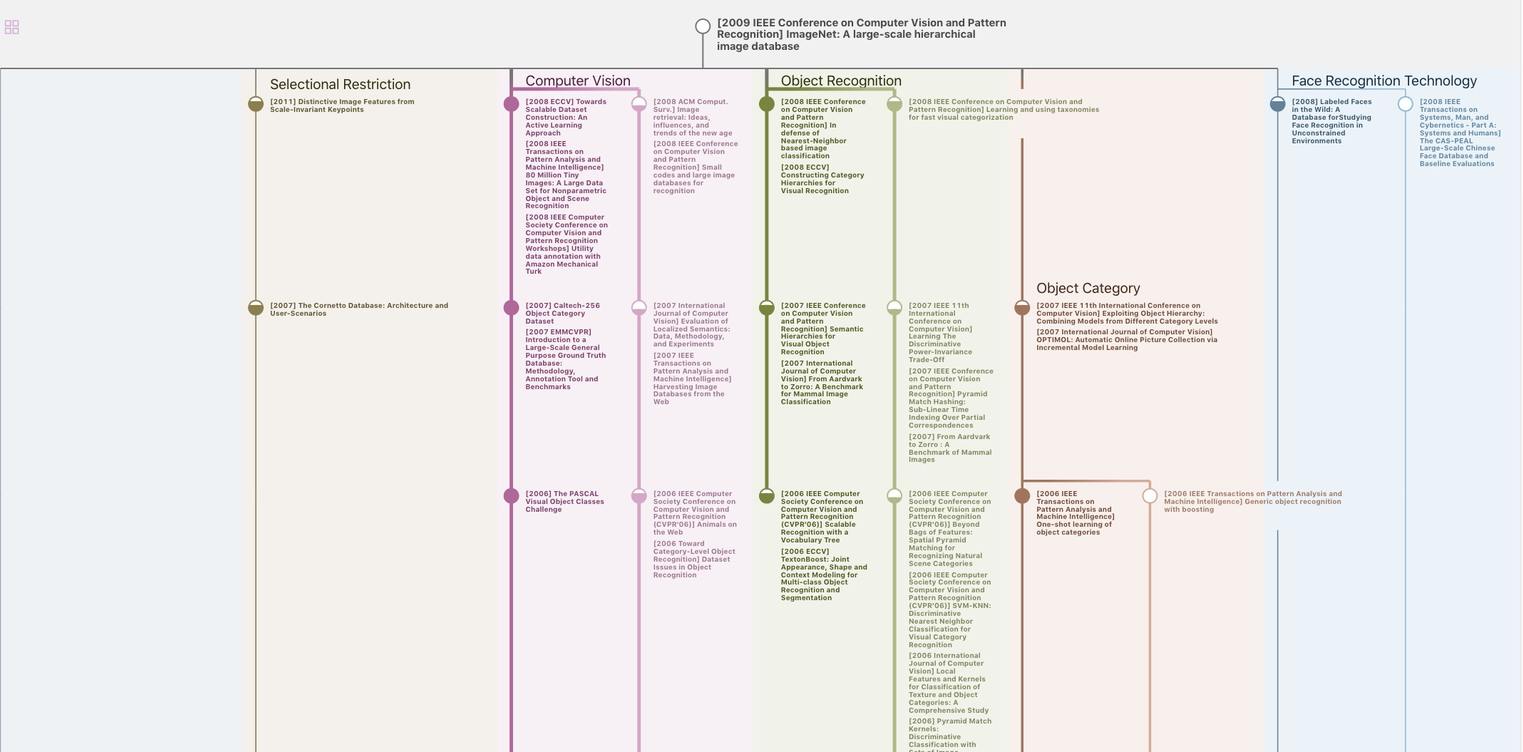
生成溯源树,研究论文发展脉络
Chat Paper
正在生成论文摘要