NaturalSpeech: End-to-End Text-to-Speech Synthesis with Human-Level Quality.
arxiv(2024)
摘要
Text-to-speech (TTS) has made rapid progress in both academia and industry in recent years. Some questions naturally arise that whether a TTS system can achieve human-level quality, how to define/judge that quality, and how to achieve it. In this paper, we answer these questions by first defining the human-level quality based on the statistical significance of subjective measure and introducing appropriate guidelines to judge it, and then developing a TTS system called NaturalSpeech that achieves human-level quality on benchmark datasets. Specifically, we leverage a variational auto-encoder (VAE) for end-to-end text-to-waveform generation, with several key modules to enhance the capacity of the prior from text and reduce the complexity of the posterior from speech, including phoneme pre-training, differentiable duration modeling, bidirectional prior/posterior modeling, and a memory mechanism in VAE. Experimental evaluations on the popular LJSpeech dataset show that our proposed NaturalSpeech achieves -0.01 CMOS (comparative mean opinion score) to human recordings at the sentence level, with Wilcoxon signed rank test at p-level p >> 0.05, which demonstrates no statistically significant difference from human recordings for the first time.
更多查看译文
关键词
Text-to-Speech,Speech Synthesis,Human-Level Quality,Variational Auto-Encoder,End-to-End
AI 理解论文
溯源树
样例
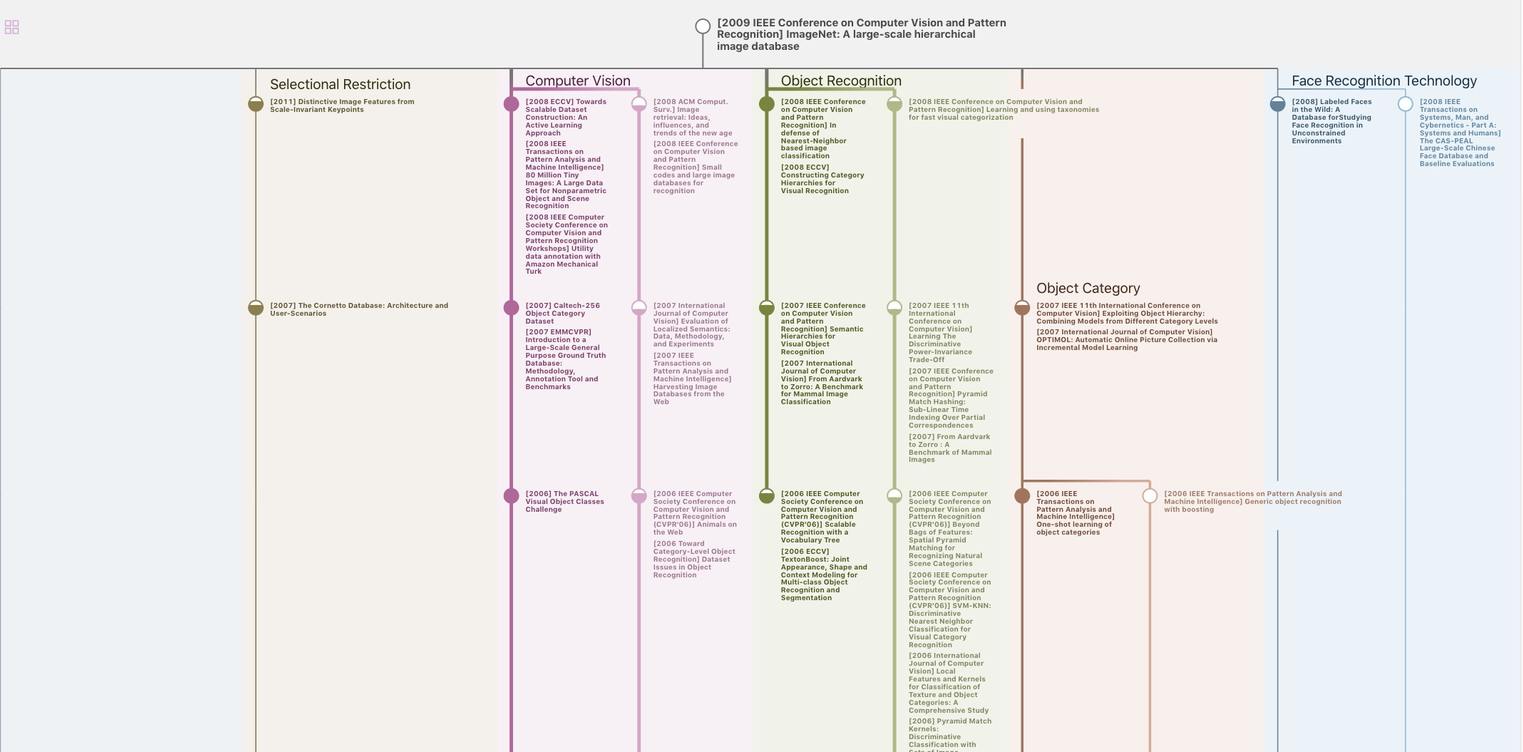
生成溯源树,研究论文发展脉络
Chat Paper
正在生成论文摘要