FP-GNN: a versatile deep learning architecture for enhanced molecular property prediction.
Briefings Bioinform.(2022)
摘要
Accurate prediction of molecular properties, such as physicochemical and bioactive properties, as well as ADME/T (absorption, distribution, metabolism, excretion and toxicity) properties, remains a fundamental challenge for molecular design, especially for drug design and discovery. In this study, we advanced a novel deep learning architecture, termed FP-GNN (fingerprints and graph neural networks), which combined and simultaneously learned information from molecular graphs and fingerprints for molecular property prediction. To evaluate the FP-GNN model, we conducted experiments on 13 public datasets, an unbiased LIT-PCBA dataset and 14 phenotypic screening datasets for breast cell lines. Extensive evaluation results showed that compared to advanced deep learning and conventional machine learning algorithms, the FP-GNN algorithm achieved state-of-the-art performance on these datasets. In addition, we analyzed the influence of different molecular fingerprints, and the effects of molecular graphs and molecular fingerprints on the performance of the FP-GNN model. Analysis of the anti-noise ability and interpretation ability also indicated that FP-GNN was competitive in real-world situations. Collectively, FP-GNN algorithm can assist chemists, biologists and pharmacists in predicting and discovering better molecules with desired functions or properties.
更多查看译文
关键词
artificial intelligence,drug design and discovery,graph attention networks,machine learning,molecular representation
AI 理解论文
溯源树
样例
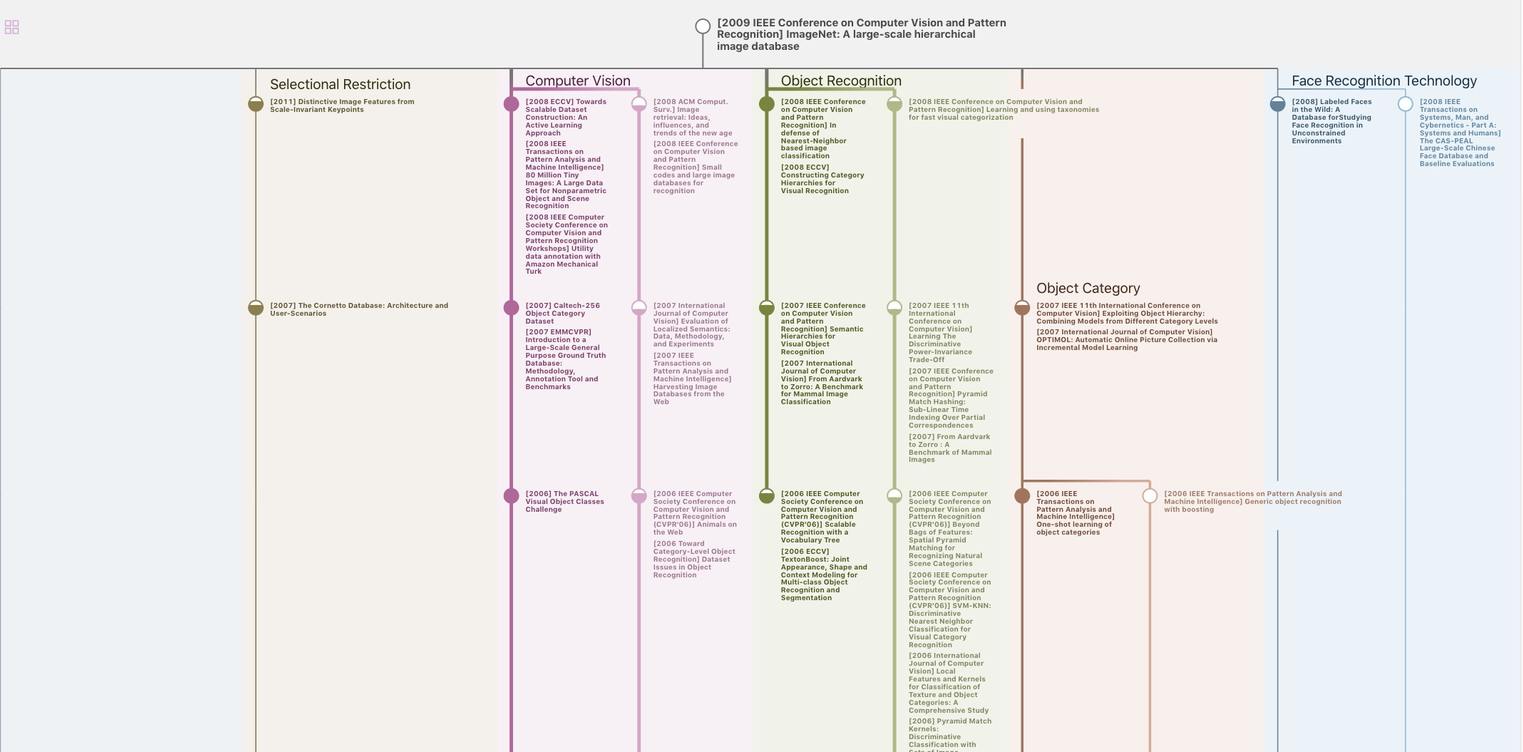
生成溯源树,研究论文发展脉络
Chat Paper
正在生成论文摘要