Automatic Velocity Picking Using a Multi-Information Fusion Deep Semantic Segmentation Network
IEEE TRANSACTIONS ON GEOSCIENCE AND REMOTE SENSING(2022)
摘要
Velocity picking, a critical step in seismic data processing, has been studied for decades. Although manual picking can produce accurate normal moveout (NMO) velocities from the velocity spectra of prestack gathers, it is time-consuming and becomes infeasible with the emergence of a large amount of seismic data. Numerous automatic velocity picking methods have thus been developed. In recent years, deep learning (DL) methods have produced good results on the seismic data with medium and high signal-to-noise ratios (SNRs). Unfortunately, it still lacks a picking method to automatically generate accurate velocities in situations of low SNR. In this article, we propose a multiinformation fusion network (MIFN) to estimate stacking velocity from the fusion information of velocity spectra and stack gather segments (SGSs). In particular, we transform the velocity picking problem into a semantic segmentation problem based on the velocity spectrum images. Meanwhile, the information provided by SGS is used as a prior in the network to assist segmentation. The experimental results on two field datasets show that the picking results of MIFN are stable and accurate for the scenarios with medium and high SNR, and it also performs well in low SNR scenarios. Code is made publicly available at https://github.com/newbee-ML/MIFN-Velocity-Picking
更多查看译文
关键词
MOS devices, Signal to noise ratio, Semantics, Image segmentation, Stacking, Feature extraction, Estimation, Deep learning (DL), stack velocity picking, velocity spectrum
AI 理解论文
溯源树
样例
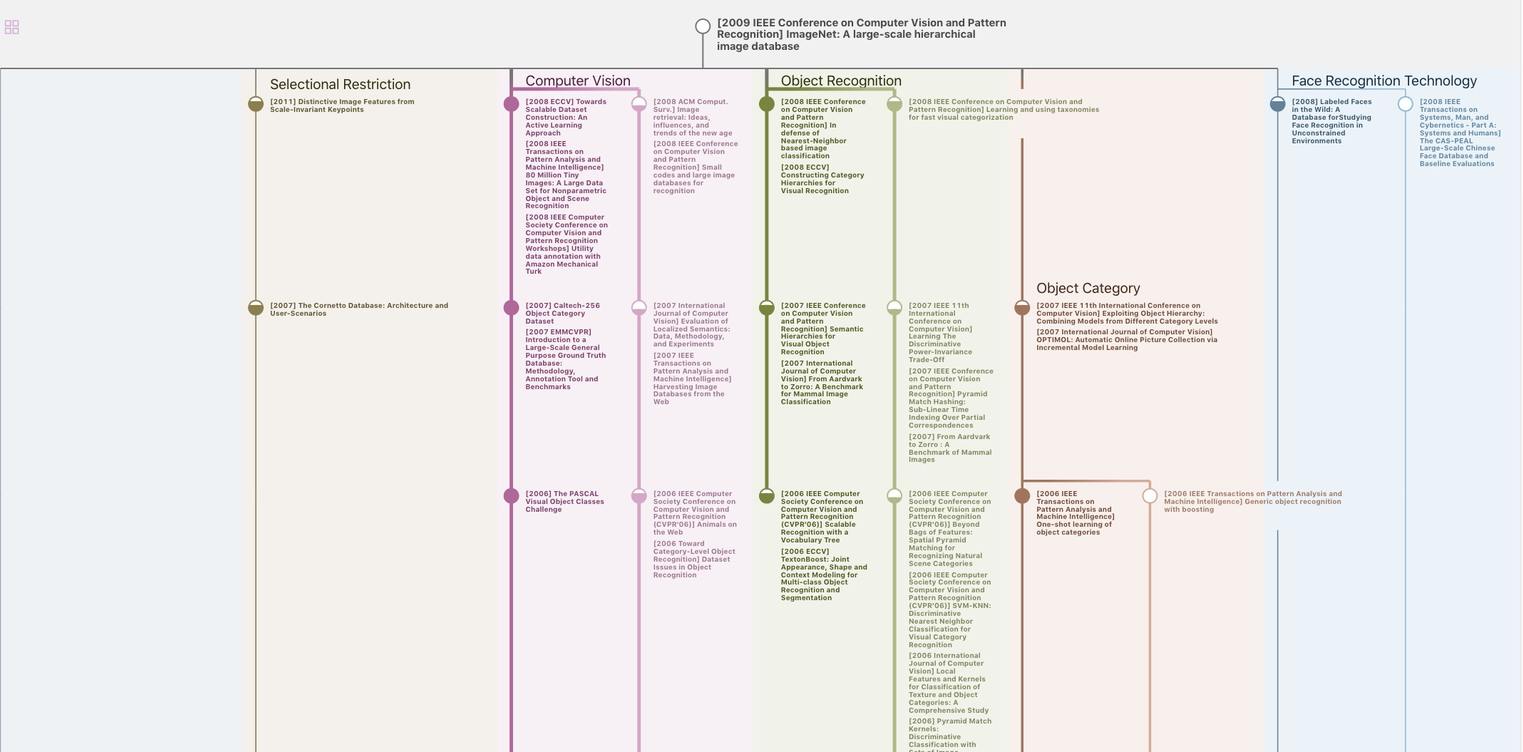
生成溯源树,研究论文发展脉络
Chat Paper
正在生成论文摘要