Efficient VVC Intra Prediction Based on Deep Feature Fusion and Probability Estimation
IEEE TRANSACTIONS ON MULTIMEDIA(2023)
摘要
The ever-growing multimedia traffic has underscored the importance of effective multimedia codecs. Among them, the up-to-date lossy video coding standard, Versatile Video Coding (VVC), has been attracting attentions of video coding community. However, the gain of VVC is achieved at the cost of significant encoding complexity, which brings the need to realize fast encoder with comparable Rate Distortion (RD) performance. In this paper, we propose to optimize the VVC complexity at intra-frame prediction, with a two-stage framework of deep feature fusion and probability estimation. At the first stage, we employ the deep convolutional network to extract the spatial-temporal neighboring coding features. Then we fuse all reference features obtained by different convolutional kernels to determine an optimal intra coding depth. At the second stage, we employ a probability-based model and the spatial-temporal coherence to select the candidate partition modes within the optimal coding depth. Finally, these selected depths and partitions are executed whilst unnecessary computations are excluded. Experimental results on standard database demonstrate the superiority of proposed method, especially for High Definition (HD) and Ultra-HD (UHD) video sequences.
更多查看译文
关键词
Encoding,Feature extraction,Computational modeling,Complexity theory,Video coding,Kernel,Convolutional neural networks,Intra coding,rate-distortion (RD),versatile video coding (VVC),video coding
AI 理解论文
溯源树
样例
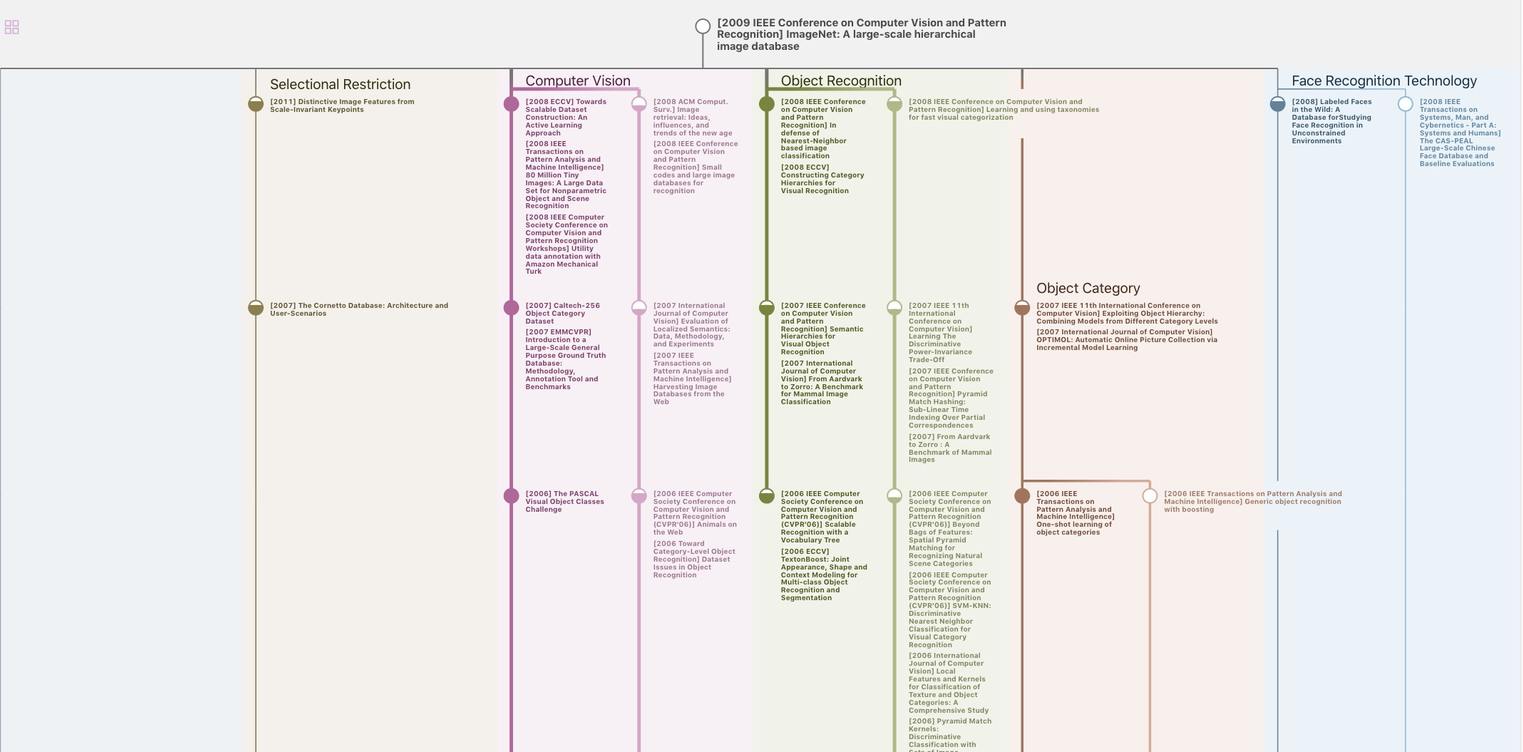
生成溯源树,研究论文发展脉络
Chat Paper
正在生成论文摘要