Robustness of Neural Architectures for Audio Event Detection
arxiv(2022)
摘要
Traditionally, in Audio Recognition pipeline, noise is suppressed by the "frontend", relying on preprocessing techniques such as speech enhancement. However, it is not guaranteed that noise will not cascade into downstream pipelines. To understand the actual influence of noise on the entire audio pipeline, in this paper, we directly investigate the impact of noise on a different types of neural models without the preprocessing step. We measure the recognition performances of 4 different neural network models on the task of environment sound classification under the 3 types of noises: \emph{occlusion} (to emulate intermittent noise), \emph{Gaussian} noise (models continuous noise), and \emph{adversarial perturbations} (worst case scenario). Our intuition is that the different ways in which these models process their input (i.e. CNNs have strong locality inductive biases, which Transformers do not have) should lead to observable differences in performance and/ or robustness, an understanding of which will enable further improvements. We perform extensive experiments on AudioSet which is the largest weakly-labeled sound event dataset available. We also seek to explain the behaviors of different models through output distribution change and weight visualization.
更多查看译文
关键词
audio event detection,neural architectures
AI 理解论文
溯源树
样例
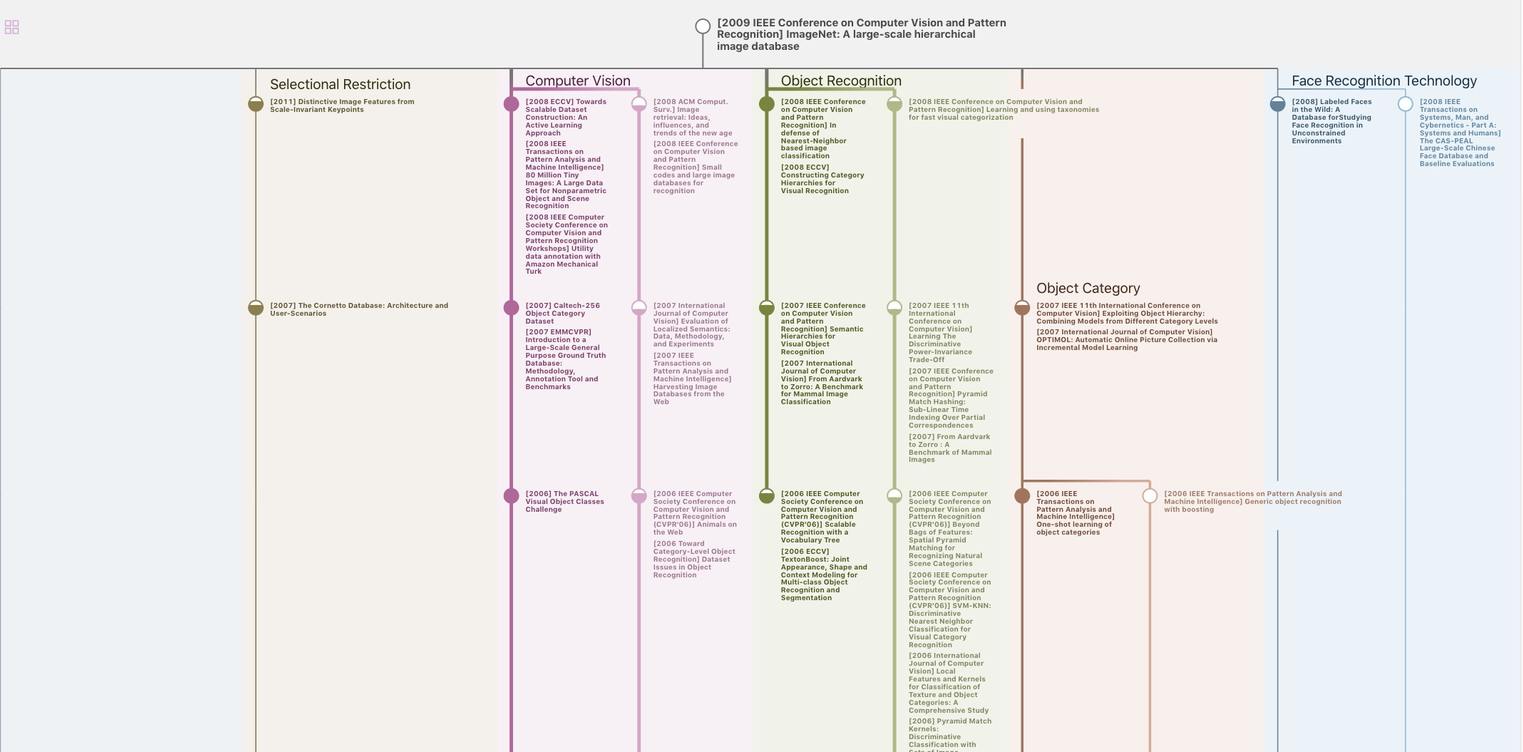
生成溯源树,研究论文发展脉络
Chat Paper
正在生成论文摘要