A Deep Reinforcement Learning-based Sliding Mode Control Design for Partially-known Nonlinear Systems
2022 EUROPEAN CONTROL CONFERENCE (ECC)(2022)
摘要
Presence of model uncertainties creates challenges for model-based control design, and complexity of the control design is further exacerbated when coping with nonlinear systems. This paper presents a sliding mode control (SMC) design approach for nonlinear systems with partially known dynamics by blending data-driven and model-based approaches. First, an SMC is designed for the available (nominal) model of the nonlinear system. The closed-loop state trajectory of the available model is used to build the desired trajectory for the partially known nonlinear system states. Next, a deep policy gradient method is used to cope with unknown parts of the system dynamics and adjust the sliding mode control output to achieve a desired state trajectory. The performance (and viability) of the proposed design approach is finally examined through numerical examples.
更多查看译文
关键词
sliding mode control design,nonlinear,learning-based,partially-known
AI 理解论文
溯源树
样例
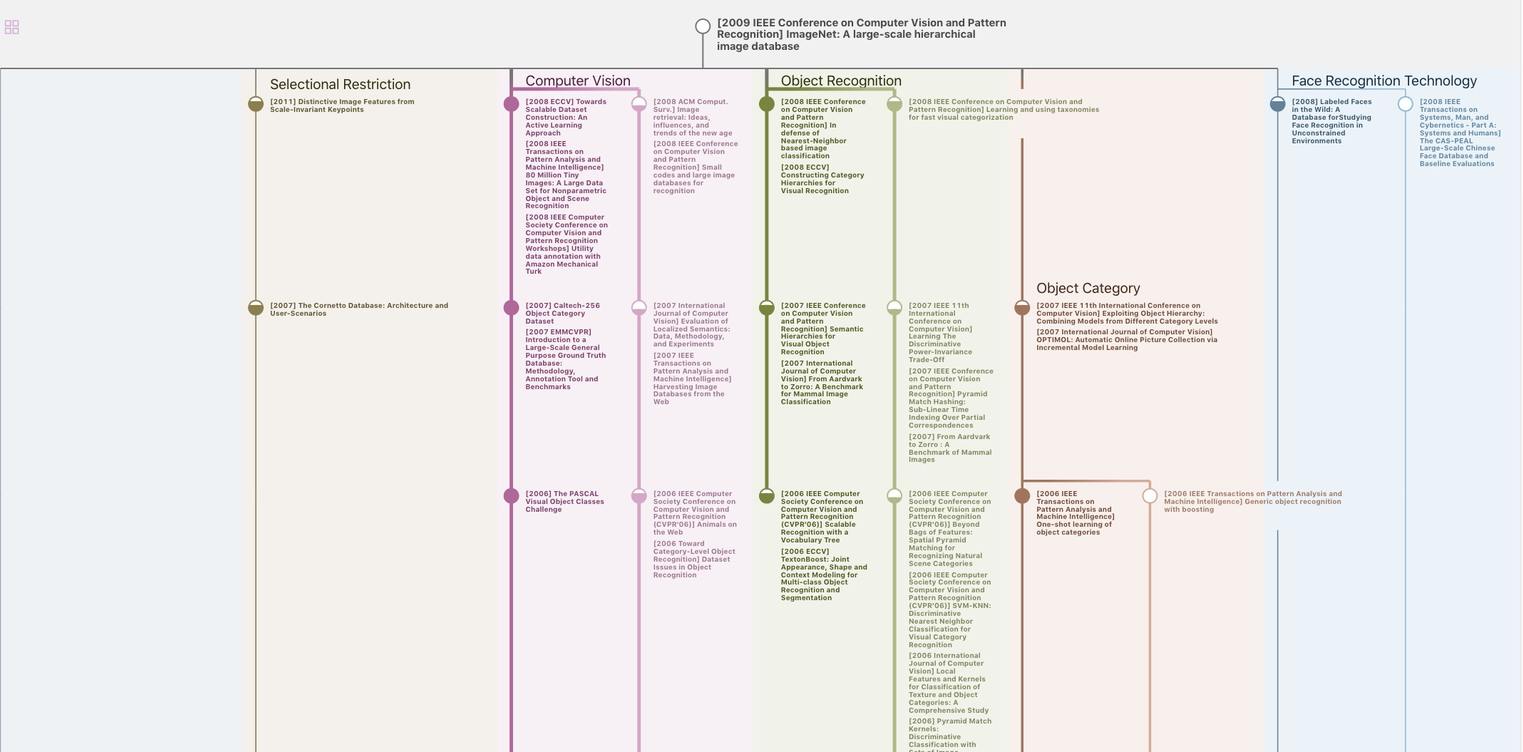
生成溯源树,研究论文发展脉络
Chat Paper
正在生成论文摘要