A Multi-Task Learning Approach for Delayed Feedback Modeling.
International Workshop on Multimodal Human Understanding for the Web and Social Media(2022)
摘要
Conversion rate (CVR) prediction is one of the most essential tasks for digital display advertising. In industrial recommender systems, online learning is particularly favored for its capability to capture the dynamic change of data distribution, which often leads to significantly improvement of conversion rates. However, the gap between a click behavior and the corresponding conversion ranges from a few minutes to days; therefore, fresh data may not have accurate label information when they are ingested by the training algorithm, which is called the delayed feedback problem of CVR prediction. To solve this problem, previous works label the delayed positive samples as negative and correct them at their conversion time, then they optimize the expectation of actual conversion distribution via important sampling under the observed distribution. However, these methods approximate the actual feature distribution as the observed feature distribution, which may introduce additional bias to the delayed feedback modeling. In this paper, we prove the observed conversion rate is the product of the actual conversion rate and the observed non-delayed positive rate. Then we propose Multi-Task Delayed Feedback Model (MTDFM), which consists of two sub-networks: actual CVR network and NDPR (non-delayed positive rate) network. We train the actual CVR network by simultaneously optimizing the observed conversion rate and non-delayed positive rate. The proposed method does not require the observed feature distribution to remain the same as the actual distribution. Finally, experimental results on both public and industrial datasets demonstrate that the proposed method outperforms the previous state-of-the-art methods consistently.
更多查看译文
AI 理解论文
溯源树
样例
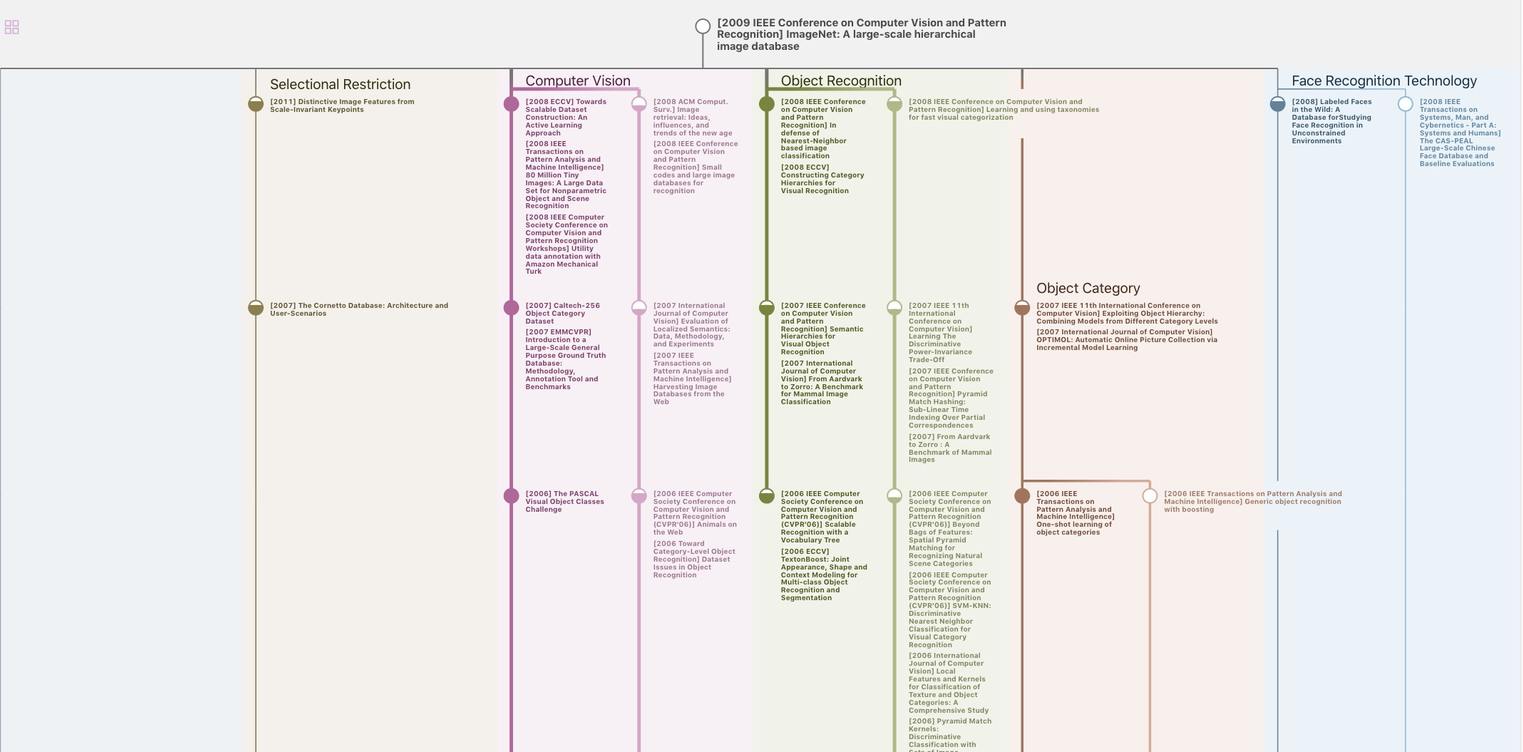
生成溯源树,研究论文发展脉络
Chat Paper
正在生成论文摘要