Enhancing Knowledge Bases with Quantity Facts
International World Wide Web Conference(2022)
摘要
ABSTRACT Machine knowledge about the world’s entities should include quantity properties, such as heights of buildings, running times of athletes, energy efficiency of car models, energy production of power plants, and more. State-of-the-art knowledge bases (KBs), such as Wikidata, cover many relevant entities but often miss the corresponding quantities. Prior work on extracting quantity facts from web contents focused on high precision for top-ranked outputs, but did not tackle the KB coverage issue. This paper presents a recall-oriented approach which aims to close this gap in knowledge-base coverage. Our method is based on iterative learning for extracting quantity facts, with two novel contributions to boost recall for KB augmentation without sacrificing the quality standards of the knowledge base. The first contribution is a query expansion technique to capture a larger pool of fact candidates. The second contribution is a novel technique for harnessing observations on value distributions for self-consistency. Experiments with extractions from more than 13 million web documents demonstrate the benefits of our method.
更多查看译文
关键词
Knowledge Bases, Information Extraction, Quantity Facts
AI 理解论文
溯源树
样例
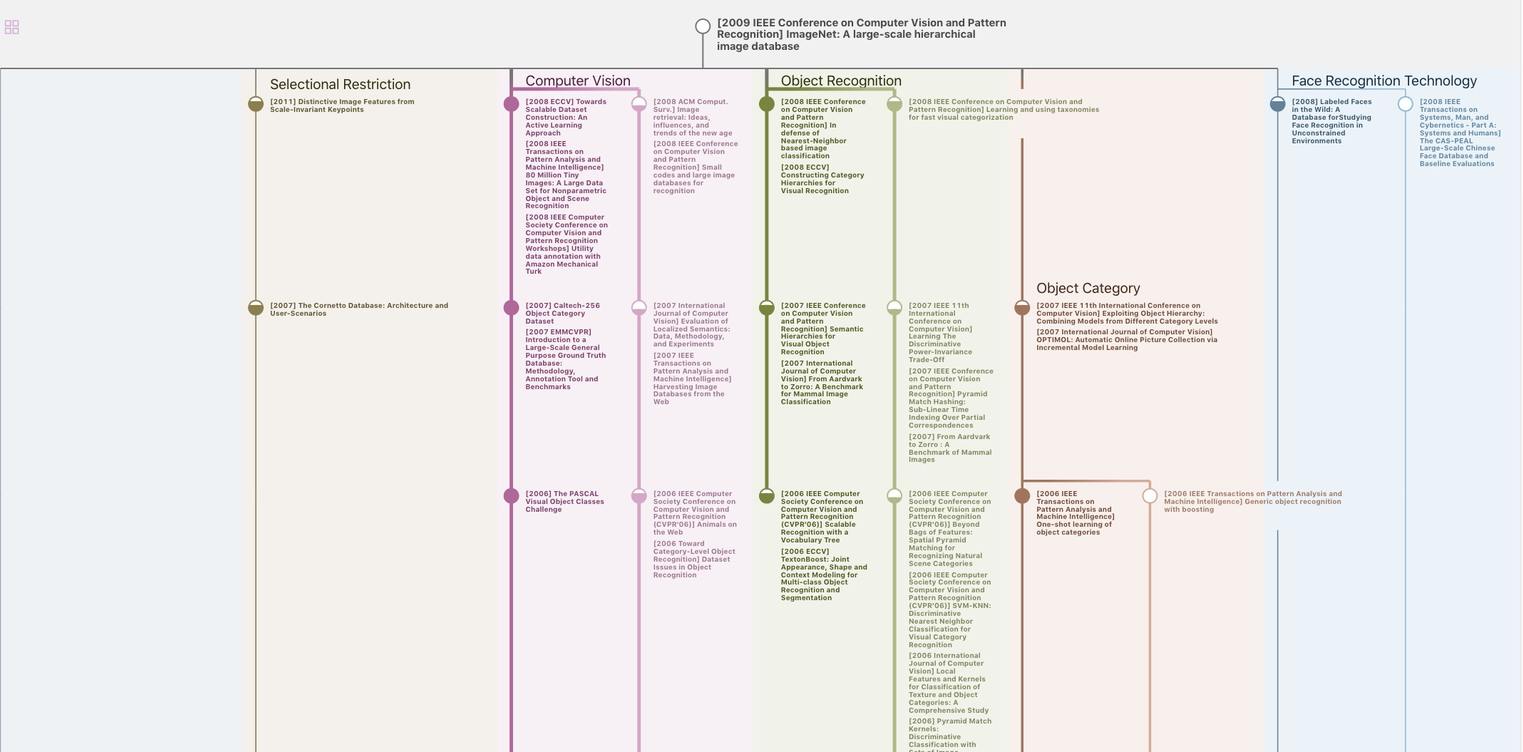
生成溯源树,研究论文发展脉络
Chat Paper
正在生成论文摘要