Optimal subsampling for functional quantile regression
Statistical Papers(2023)
摘要
Subsampling is an efficient method to deal with massive data. In this paper, we investigate the optimal subsampling for linear quantile regression when the covariates are functions. The asymptotic distribution of the subsampling estimator is first derived. Then, we obtain the optimal subsampling probabilities based on the A-optimality criterion. Furthermore, the modified subsampling probabilities without estimating the densities of the response variables given the covariates are also proposed, which are easier to implement in practise. Numerical experiments on synthetic and real data show that the proposed methods always outperform the one with uniform sampling and can approximate the results based on full data well with less computational efforts.
更多查看译文
关键词
Functional quantile regression,A-optimality,Asymptotic distribution,Optimal subsampling,Massive data
AI 理解论文
溯源树
样例
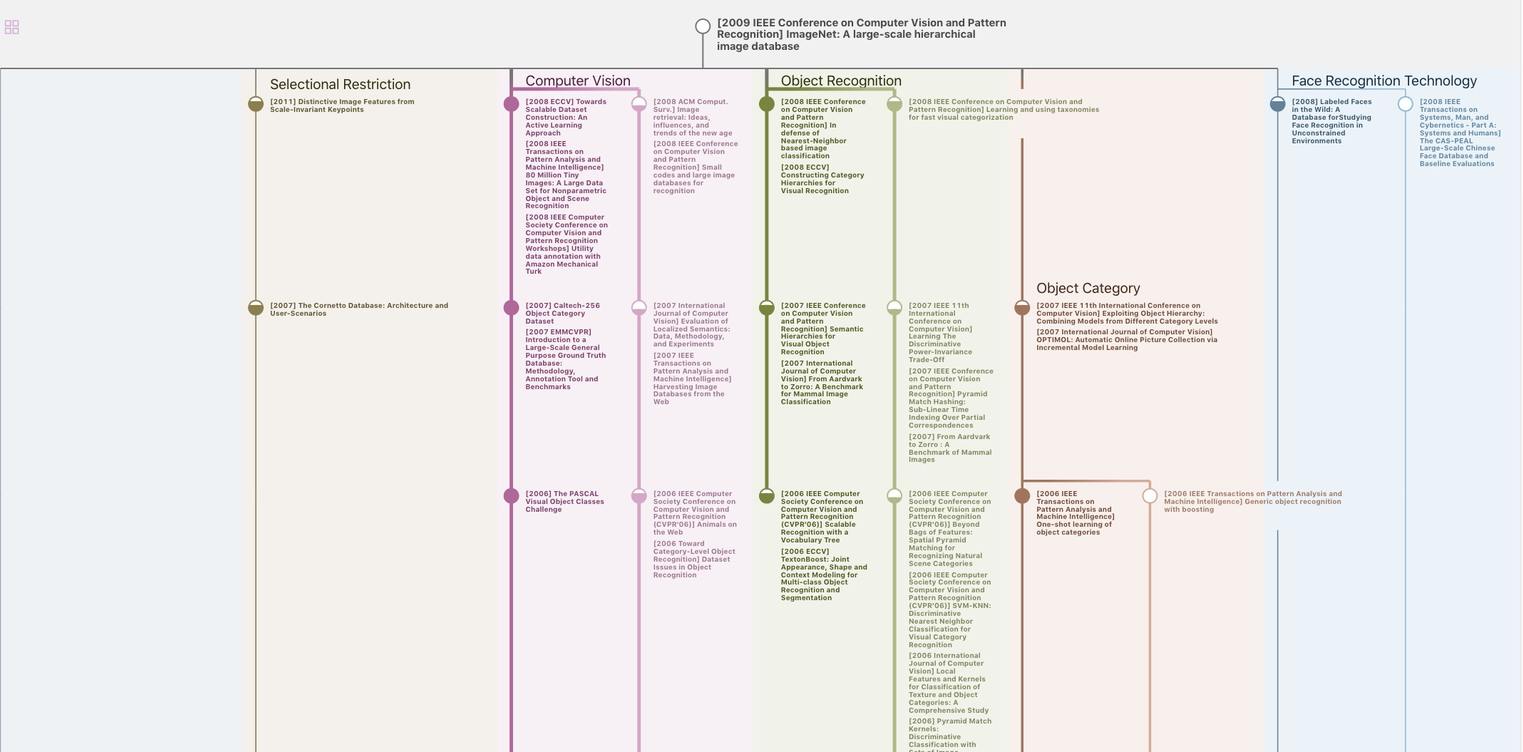
生成溯源树,研究论文发展脉络
Chat Paper
正在生成论文摘要