Contrastive Hierarchical Clustering
MACHINE LEARNING AND KNOWLEDGE DISCOVERY IN DATABASES: RESEARCH TRACK, ECML PKDD 2023, PT I(2023)
摘要
Deep clustering has been dominated by flat models, which split a dataset into a predefined number of groups. Although recent methods achieve an extremely high similarity with the ground truth on popular benchmarks, the information contained in the flat partition is limited. In this paper, we introduce CoHiClust, a Contrastive Hierarchical Clustering model based on deep neural networks, which can be applied to typical image data. By employing a self-supervised learning approach, CoHiClust distills the base network into a binary tree without access to any labeled data. The hierarchical clustering structure can be used to analyze the relationship between clusters, as well as to measure the similarity between data points. Experiments demonstrate that CoHiClust generates a reasonable structure of clusters, which is consistent with our intuition and image semantics. Moreover, it obtains superior clustering accuracy on most of the image datasets compared to the state-of-the-art flat clustering models. Our implementation is available at https:// github.com/MichalZnalezniak/Contrastive-Hierarchical-Clustering.
更多查看译文
关键词
Hierarchical clustering,Contrastive learning,Deep embedding clustering
AI 理解论文
溯源树
样例
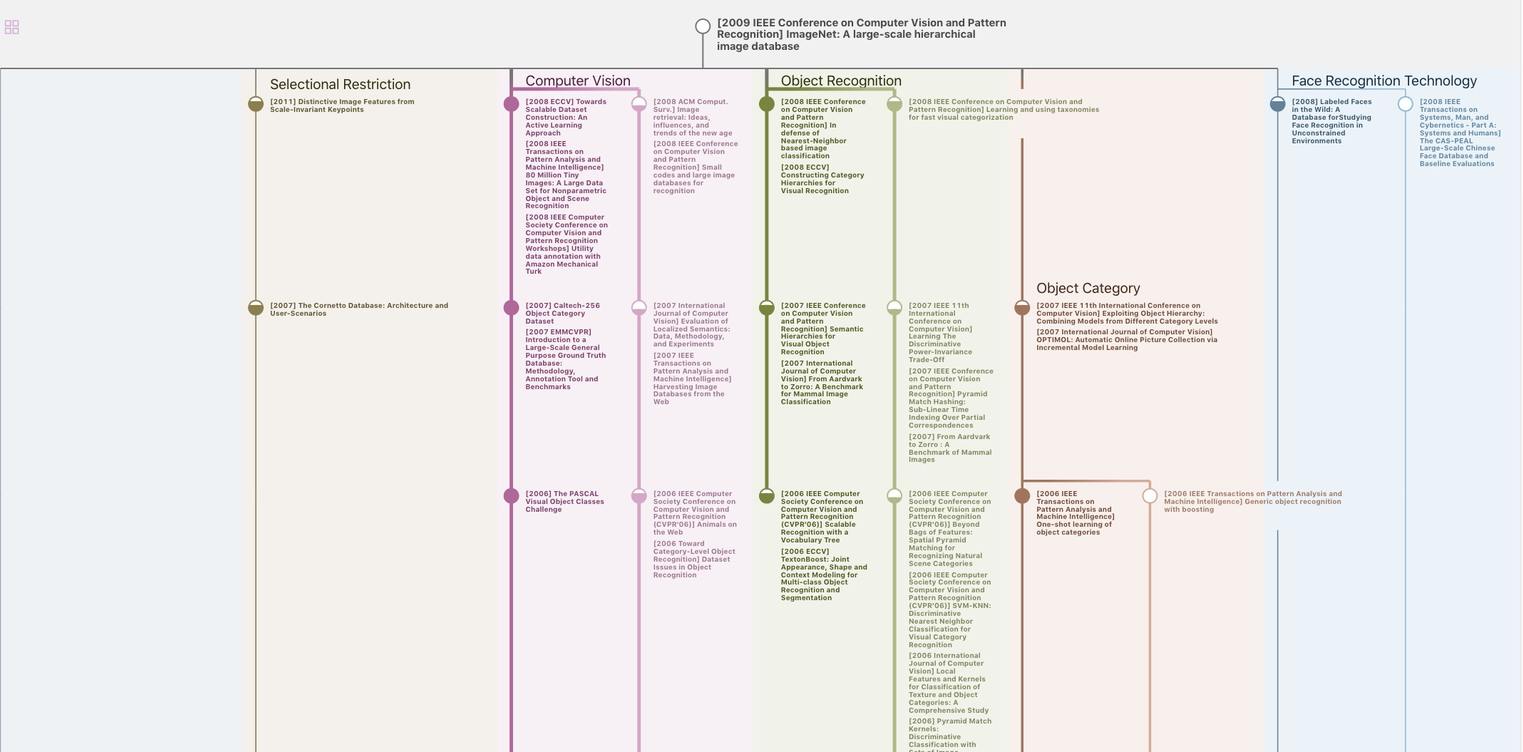
生成溯源树,研究论文发展脉络
Chat Paper
正在生成论文摘要