Uncertainty Minimization for Personalized Federated Semi-Supervised Learning
IEEE Transactions on Network Science and Engineering(2023)
摘要
Since federated learning (FL) has been introduced as a decentralized learning technique with privacy preservation, statistical heterogeneity of distributed data stays the main obstacle to achieve robust performance and stable convergence in FL applications. Model personalization methods have been studied to overcome this problem. However, existing approaches are mainly under the prerequisite of fully labeled data, which is unrealistic in practice due to the requirement of expertise. The primary issue caused by partial-labeled condition is that, clients with deficient labeled data can suffer from unfair performance gain because they lack adequate insights of local distribution to customize the global model. To tackle this problem, 1) we propose a novel personalized semi-supervised learning paradigm which allows partial-labeled or unlabeled clients to seek labeling assistance from data-related clients (helper agents), thus to enhance their perception of local data; 2) based on this paradigm, we design an uncertainty-based data-relation metric to ensure that selected helpers can provide trustworthy pseudo labels instead of misleading the local training; 3) to mitigate the network overload introduced by helper searching, we further develop a helper selection protocol to achieve efficient communication with acceptable performance sacrifice. Experiments show that our proposed method can obtain superior performance and more stable convergence than other related works with partially labeled data, especially in highly heterogeneous setting.
更多查看译文
关键词
Data heterogeneity,federated learning,semi-supervised learning,uncertainty estimation
AI 理解论文
溯源树
样例
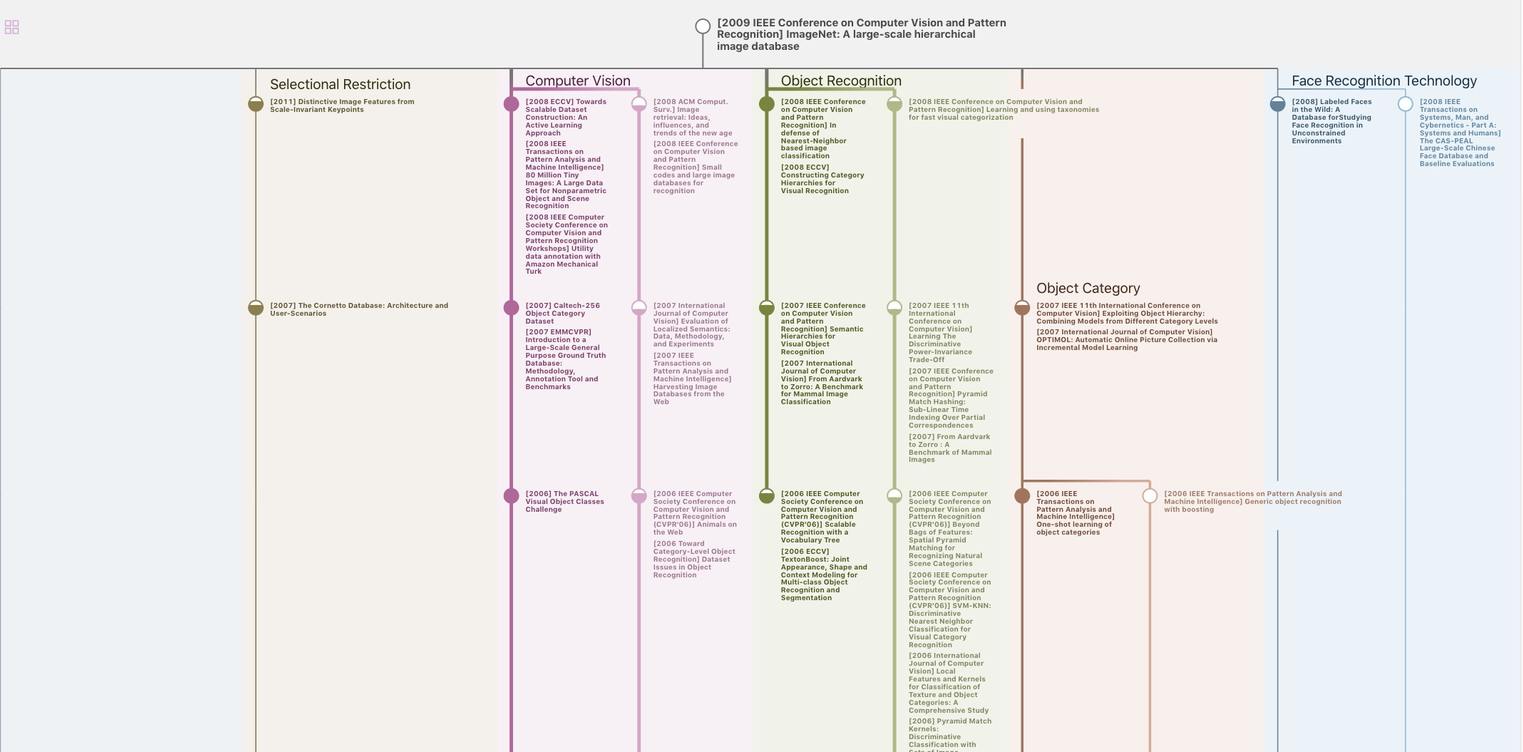
生成溯源树,研究论文发展脉络
Chat Paper
正在生成论文摘要