Correntropy-Based Low-Rank Matrix Factorization With Constraint Graph Learning for Image Clustering.
IEEE transactions on neural networks and learning systems(2022)
摘要
This article proposes a novel low-rank matrix factorization model for semisupervised image clustering. In order to alleviate the negative effect of outliers, the maximum correntropy criterion (MCC) is incorporated as a metric to build the model. To utilize the label information to improve the clustering results, a constraint graph learning framework is proposed to adaptively learn the local structure of the data by considering the label information. Furthermore, an iterative algorithm based on Fenchel conjugate (FC) and block coordinate update (BCU) is proposed to solve the model. The convergence properties of the proposed algorithm are analyzed, which shows that the algorithm exhibits both objective sequential convergence and iterate sequential convergence. Experiments are conducted on six real-world image datasets, and the proposed algorithm is compared with eight state-of-the-art methods. The results show that the proposed method can achieve better performance in most situations in terms of clustering accuracy and mutual information.
更多查看译文
关键词
Data models,Laplace equations,Convergence,Clustering algorithms,Adaptation models,Principal component analysis,Image reconstruction,Low-rank factorization,machine learning,maximum correntropy criterion (MCC),semisupervised learning (SSL)
AI 理解论文
溯源树
样例
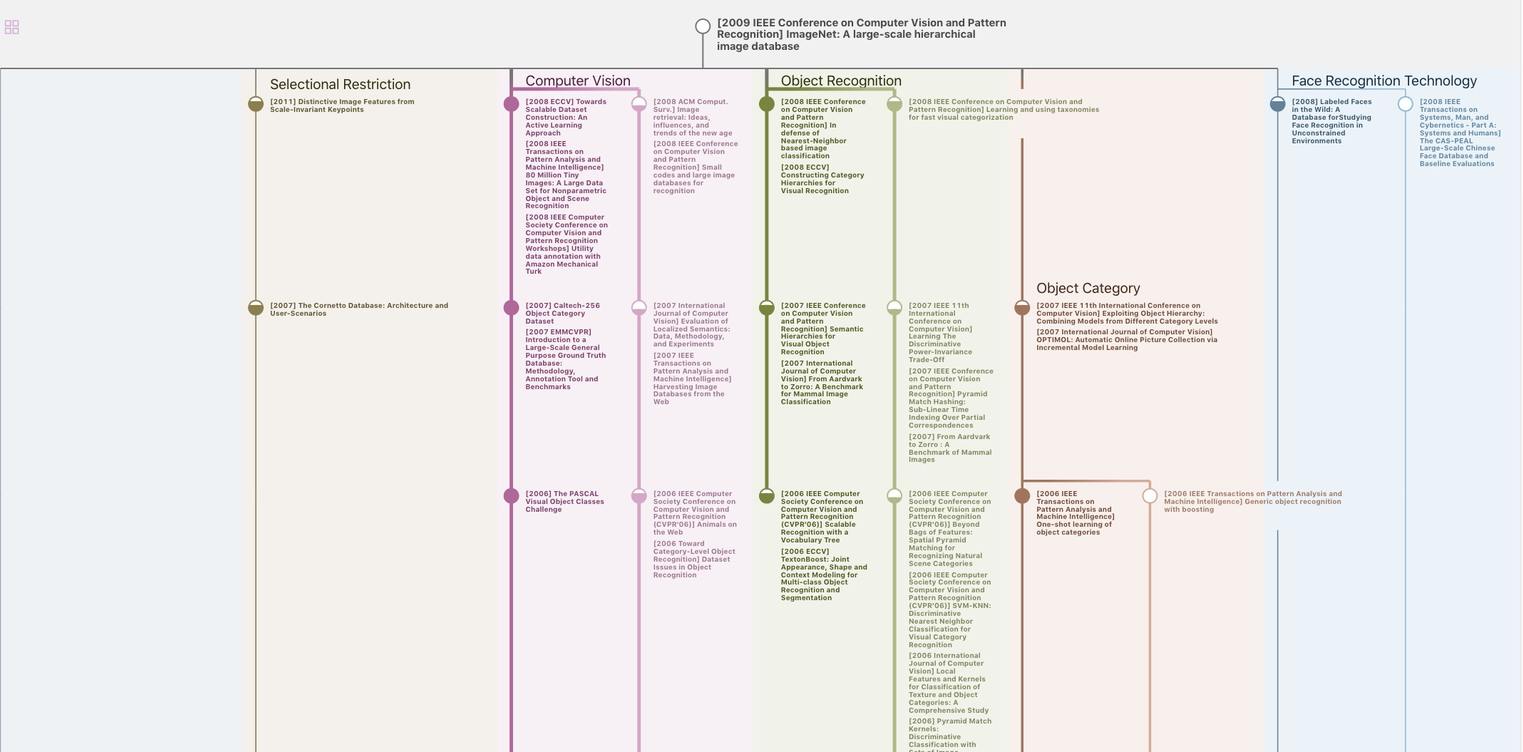
生成溯源树,研究论文发展脉络
Chat Paper
正在生成论文摘要