Does a PESQNet (Loss) Require a Clean Reference Input? The Original PESQ Does, But ACR Listening Tests Don’t
2022 International Workshop on Acoustic Signal Enhancement (IWAENC)(2022)
摘要
Perceptual evaluation of speech quality (PESQ) requires a clean speech reference as input, but predicts the results from (reference-free) absolute category rating (ACR) tests. In this work, we train a fully convolutional recurrent neural network (FCRN) as deep noise suppression (DNS) model, with either a non-intrusive or an intrusive PESQNet, where only the latter has access to a clean speech reference. The PESQNet is used as a mediator providing a perceptual loss during the DNS training to maximize the PESQ score of the enhanced speech signal. For the intrusive PESQNet, we investigate two topologies, called early-fusion (EF) and middle-fusion (MF) PESQNet, and compare to the non-intrusive PESQNet to evaluate and to quantify the benefits of employing a clean speech reference input during DNS training. Detailed analyses show that the DNS model trained with the MF-intrusive PESQNet outperforms the Interspeech 2021 DNS Challenge baseline and the one trained with an MSE loss by 0.23 and 0.12 PESQ points, respectively. Furthermore, we can show that only marginal benefits are obtained compared to the DNS trained with the non-intrusive PESQNet. Therefore, as ACR listening tests, the PESQNet does not necessarily require a clean speech reference input, opening the possibility of using real data for DNS training.
更多查看译文
关键词
Deep noise suppression,intrusive / non-intrusive PESQ estimation,convolutional recurrent neural network
AI 理解论文
溯源树
样例
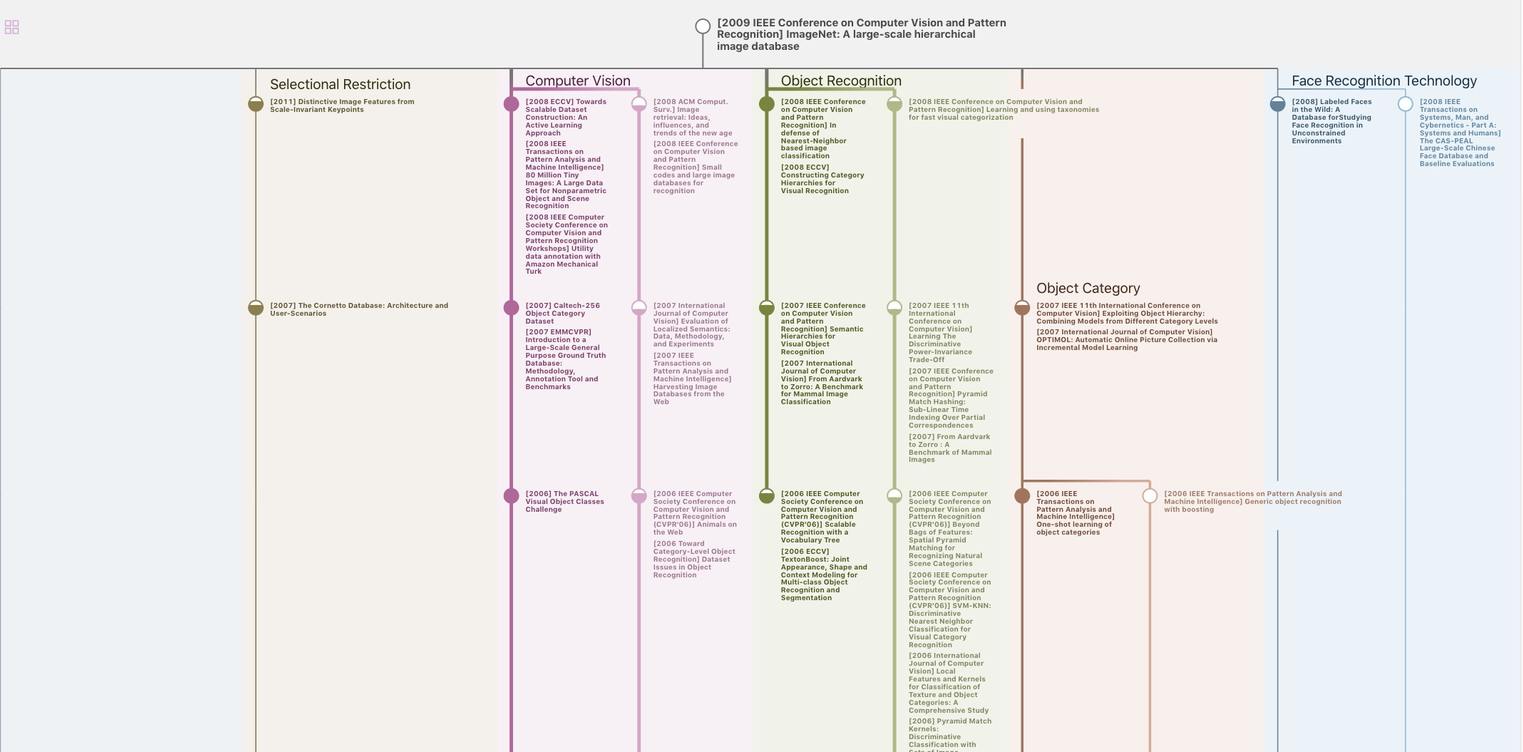
生成溯源树,研究论文发展脉络
Chat Paper
正在生成论文摘要