Self-Supervised Learning for Invariant Representations From Multi-Spectral and SAR Images
IEEE JOURNAL OF SELECTED TOPICS IN APPLIED EARTH OBSERVATIONS AND REMOTE SENSING(2022)
摘要
Self-supervised learning (SSL) has become the new state of the art in several domain classification and segmentation tasks. One popular category of SSL are distillation networks, such as Bootstrap Your Own Latent (BYOL). This work proposes RS-BYOL, which builds on BYOL in the remote sensing (RS) domain where data are nontrivially different from natural RGB images. Since multispectral (MS) and synthetic aperture radar (SAR) sensors provide varied spectral and spatial resolution information, we utilize them as an implicit augmentation to learn invariant feature embeddings. In order to learn RS-based invariant features with SSL, we trained RS-BYOL in two ways, i.e., single channel feature learning and three channel feature learning. This work explores the usefulness of single channel feature learning from random 10 MS bands of 10-20 m resolution and VV-VH of SAR bands compared to the common notion of using three or more bands. In our linear probing evaluation, these single channel features reached a 0.92 F1 score on the EuroSAT classification task and 59.6 mIoU on the IEEE Data Fusion Contest segmentation task for certain single bands. We also compare our results with ImageNet weights and show that the RS-based SSL model outperforms the supervised ImageNet-based model. We further explore the usefulness of multimodal data compared to single modality data, and it is shown that utilizing MS and SAR data allows better invariant representations to be learnt than utilizing only MS data.
更多查看译文
关键词
Synthetic aperture radar, Task analysis, Optical sensors, Optical imaging, Representation learning, Remote sensing, Satellites, Optical-synthetic aperture radar (SAR) fusion, satellite images, self-supervised learning (SSL), unsupervised learning
AI 理解论文
溯源树
样例
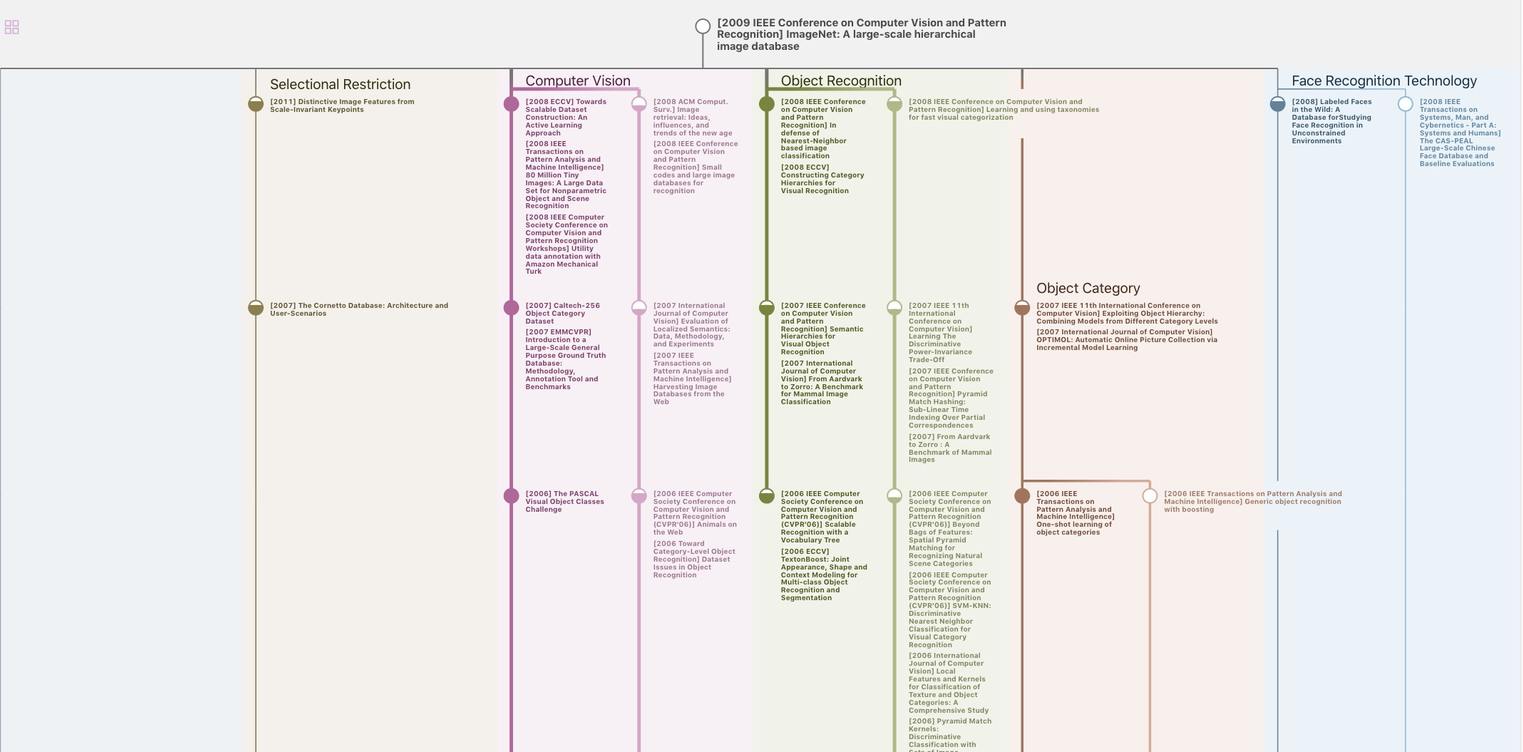
生成溯源树,研究论文发展脉络
Chat Paper
正在生成论文摘要