Wild Patterns Reloaded: A Survey of Machine Learning Security against Training Data Poisoning
arxiv(2023)
摘要
The success of machine learning is fueled by the increasing availability of computing power and large training datasets. The training data is used to learn new models or update existing ones, assuming that it is sufficiently representative of the data that will be encountered at test time. This assumption is challenged by the threat of poisoning, an attack that manipulates the training data to compromise the model's performance at test time. Although poisoning has been acknowledged as a relevant threat in industry applications, and a variety of different attacks and defenses have been proposed so far, a complete systematization and critical review of the field is still missing. In this survey, we provide a comprehensive systematization of poisoning attacks and defenses in machine learning, reviewing more than 100 papers published in the field in the past 15 years. We start by categorizing the current threat models and attacks and then organize existing defenses accordingly. While we focus mostly on computer-vision applications, we argue that our systematization also encompasses state-of-the-art attacks and defenses for other data modalities. Finally, we discuss existing resources for research in poisoning and shed light on the current limitations and open research questions in this research field.
更多查看译文
关键词
Poisoning attacks,backdoor attacks,machine learning,computer vision,computer security
AI 理解论文
溯源树
样例
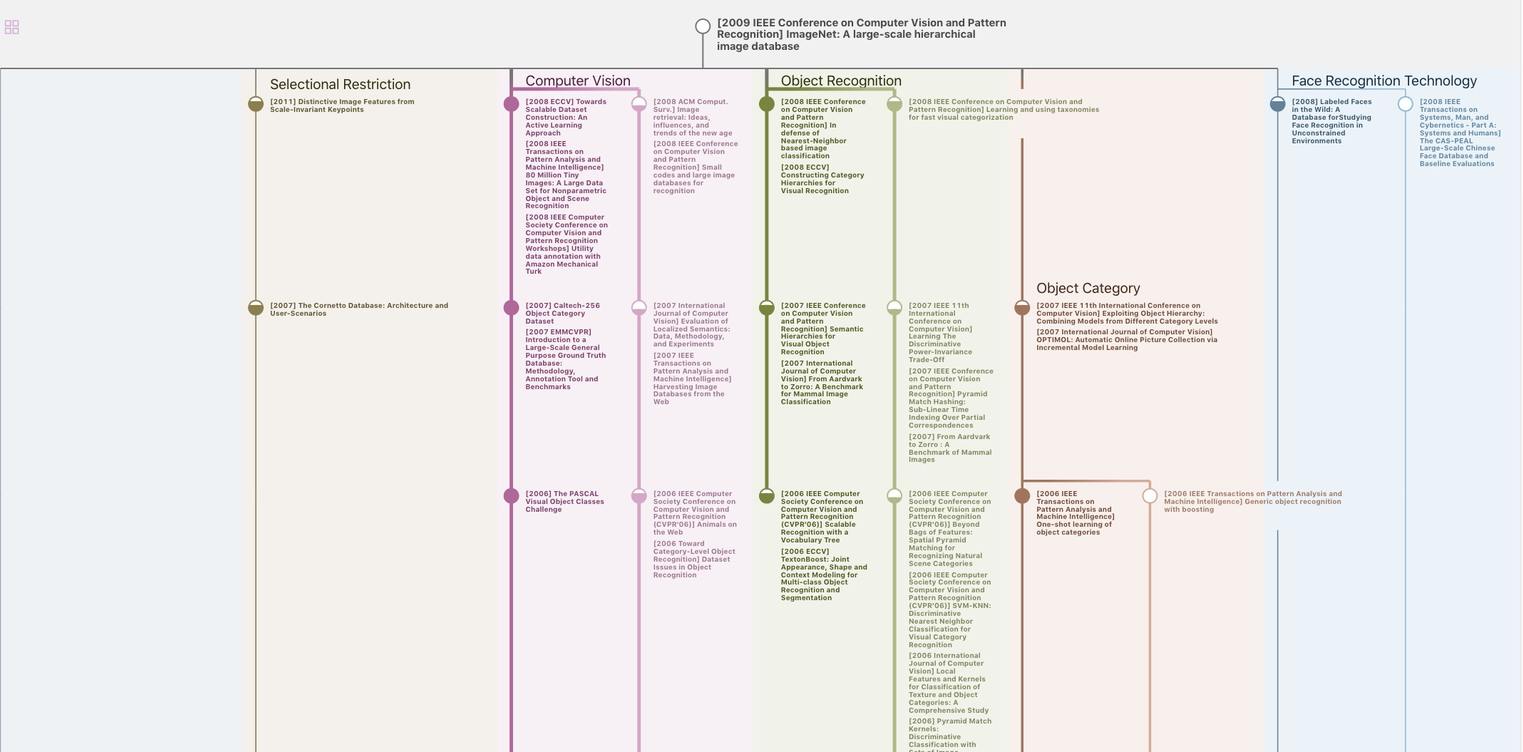
生成溯源树,研究论文发展脉络
Chat Paper
正在生成论文摘要