FedMix: Mixed Supervised Federated Learning for Medical Image Segmentation
arxiv(2023)
摘要
The purpose of federated learning is to enable multiple clients to jointly train a machine learning model without sharing data. However, the existing methods for training an image segmentation model have been based on an unrealistic assumption that the training set for each local client is annotated in a similar fashion and thus follows the same image supervision level. To relax this assumption, in this work, we propose a label-agnostic unified federated learning framework, named FedMix, for medical image segmentation based on mixed image labels. In FedMix, each client updates the federated model by integrating and effectively making use of all available labeled data ranging from strong pixel-level labels, weak bounding box labels, to weakest image-level class labels. Based on these local models, we further propose an adaptive weight assignment procedure across local clients, where each client learns an aggregation weight during the global model update. Compared to the existing methods, FedMix not only breaks through the constraint of a single level of image supervision but also can dynamically adjust the aggregation weight of each local client, achieving rich yet discriminative feature representations. Experimental results on multiple publicly-available datasets validate that the proposed FedMix outperforms the state-of-the-art methods by a large margin. In addition, we demonstrate through experiments that FedMix is extendable to multi-class medical image segmentation and much more feasible in clinical scenarios. The code is available at: https://github.com/Jwicaksana/FedMix.
更多查看译文
关键词
Federated learning,mixed supervision,medical image segmentation,pseudo labeling,adaptive weight aggregation
AI 理解论文
溯源树
样例
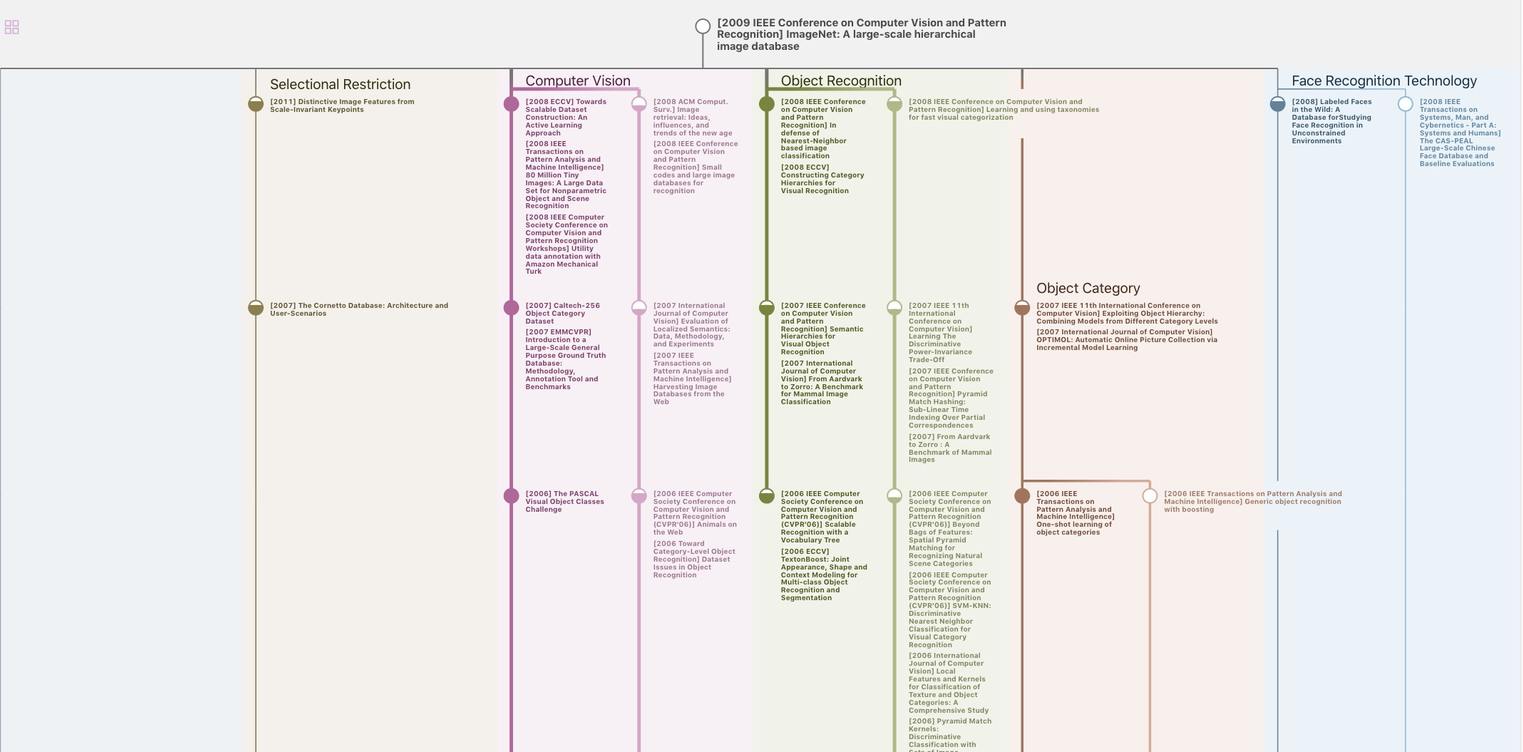
生成溯源树,研究论文发展脉络
Chat Paper
正在生成论文摘要