Data-Consistent non-Cartesian deep subspace learning for efficient dynamic MR image reconstruction.
Proceedings. IEEE International Symposium on Biomedical Imaging(2022)
摘要
Non-Cartesian sampling with subspace-constrained image reconstruction is a popular approach to dynamic MRI, but slow iterative reconstruction limits its clinical application. Data-consistent (DC) deep learning can accelerate reconstruction with good image quality, but has not been formulated for non-Cartesian subspace imaging. In this study, we propose a DC non-Cartesian deep subspace learning framework for fast, accurate dynamic MR image reconstruction. Four novel DC formulations are developed and evaluated: two gradient decent approaches, a directly solved approach, and a conjugate gradient approach. We applied a U-Net model with and without DC layers to reconstruct T1-weighted images for cardiac MR Multitasking (an advanced multidimensional imaging method), comparing our results to the iteratively reconstructed reference. Experimental results show that the proposed framework significantly improves reconstruction accuracy over the U-Net model without DC, while significantly accelerating the reconstruction over conventional iterative reconstruction.
更多查看译文
关键词
Deep learning,Dynamic MRI,MRI reconstruction,Non-Cartesian,Subspace
AI 理解论文
溯源树
样例
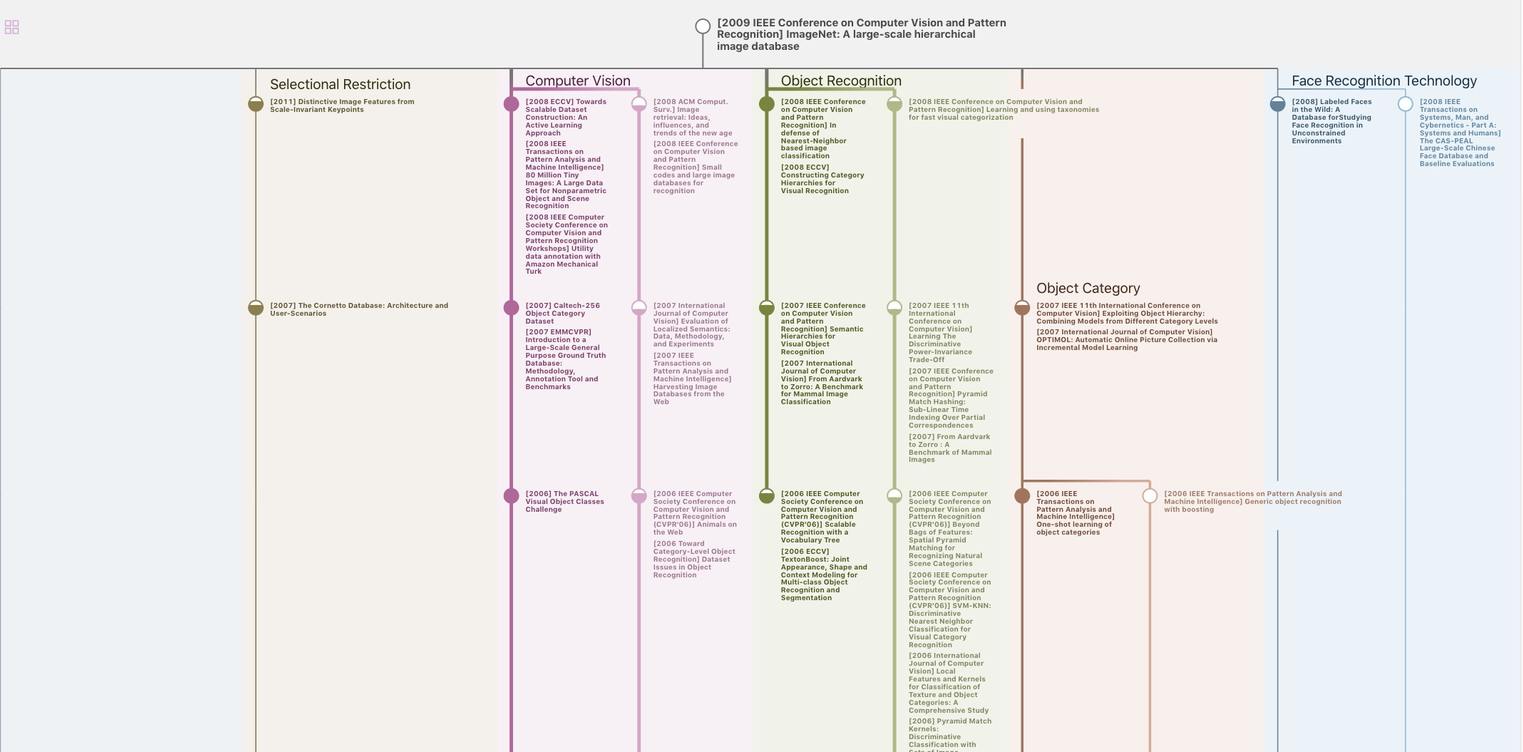
生成溯源树,研究论文发展脉络
Chat Paper
正在生成论文摘要