Conventional regression analysis and machine learning in prediction of anastomotic leakage and pulmonary complications after esophagogastric cancer surgery
JOURNAL OF SURGICAL ONCOLOGY(2022)
摘要
Background and Objectives With the current advanced data-driven approach to health care, machine learning is gaining more interest. The current study investigates the added value of machine learning to linear regression in predicting anastomotic leakage and pulmonary complications after upper gastrointestinal cancer surgery. Methods All patients in the Dutch Upper Gastrointestinal Cancer Audit undergoing curatively intended esophageal or gastric cancer surgeries from 2011 to 2017 were included. Anastomotic leakage was defined as any clinically or radiologically proven anastomotic leakage. Pulmonary complications entailed: pneumonia, pleural effusion, respiratory failure, pneumothorax, and/or acute respiratory distress syndrome. Different machine learning models were tested. Nomograms were constructed using Least Absolute Shrinkage and Selection Operator. Results Between 2011 and 2017, 4228 patients underwent surgical resection for esophageal cancer, of which 18% developed anastomotic leakage and 30% a pulmonary complication. Of the 2199 patients with surgical resection for gastric cancer, 7% developed anastomotic leakage and 15% a pulmonary complication. In all cases, linear regression had the highest predictive value with the area under the curves varying between 61.9 and 68.0, but the difference with machine learning models did not reach statistical significance. Conclusion Machine learning models can predict postoperative complications in upper gastrointestinal cancer surgery, but they do not outperform the current gold standard, linear regression
更多查看译文
关键词
cancer, complications, machine learning, mortality, risk factors, upper gastrointestinal surgery
AI 理解论文
溯源树
样例
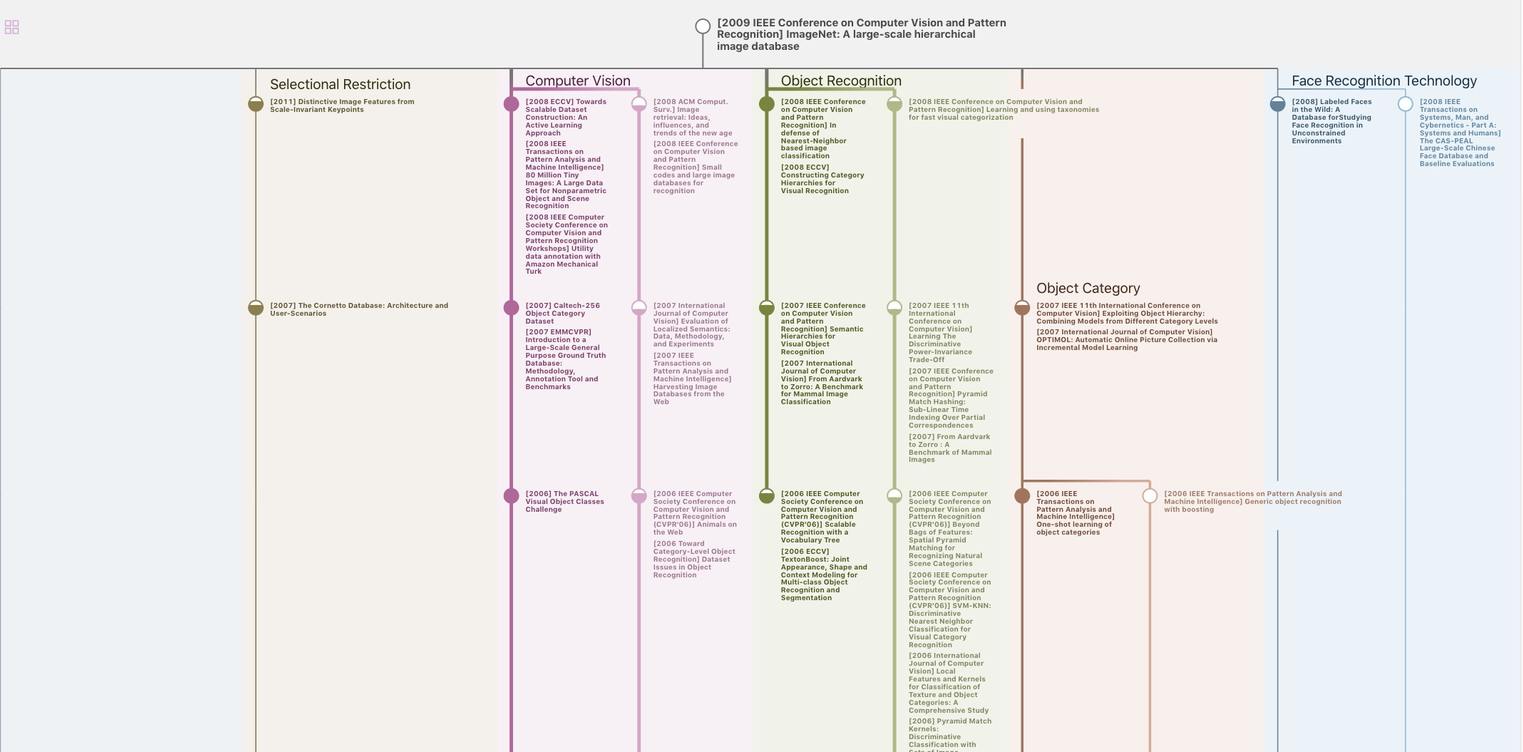
生成溯源树,研究论文发展脉络
Chat Paper
正在生成论文摘要