Learning intraoperative organ manipulation with context-based reinforcement learning
International Journal of Computer Assisted Radiology and Surgery(2022)
摘要
Purpose Automation of sub-tasks during robotic surgery is challenging due to the high variability of the surgical scenes intra- and inter-patients. For example, the pick and place task can be executed different times during the same operation and for distinct purposes. Hence, designing automation solutions that can generalise a skill over different contexts becomes hard. All the experiments are conducted using the Pneumatic Attachable Flexible (PAF) rail, a novel surgical tool designed for robotic-assisted intraoperative organ manipulation. Methods We build upon previous open-source surgical Reinforcement Learning (RL) training environment to develop a new RL framework for manipulation skills, rlman . In rlman , contextual RL agents are trained to solve different aspects of the pick and place task using the PAF rail system. rlman is implemented to support both low- and high-dimensional state information to solve surgical sub-tasks in a simulation environment. Results We use rlman to train state of the art RL agents to solve four different surgical sub-tasks involving manipulation skills using the PAF rail. We compare the results with state-of-the-art benchmarks found in the literature. We evaluate the ability of the agent to be able to generalise over different aspects of the targeted surgical environment. Conclusion We have shown that the rlman framework can support the training of different RL algorithms for solving surgical sub-task, analysing the importance of context information for generalisation capabilities. We are aiming to deploy the trained policy on the real da Vinci using the dVRK and show that the generalisation of the trained policy can be transferred to the real world.
更多查看译文
关键词
Computer-assisted intervention, Robotic surgery, Reinforcement learning, Surgical automation
AI 理解论文
溯源树
样例
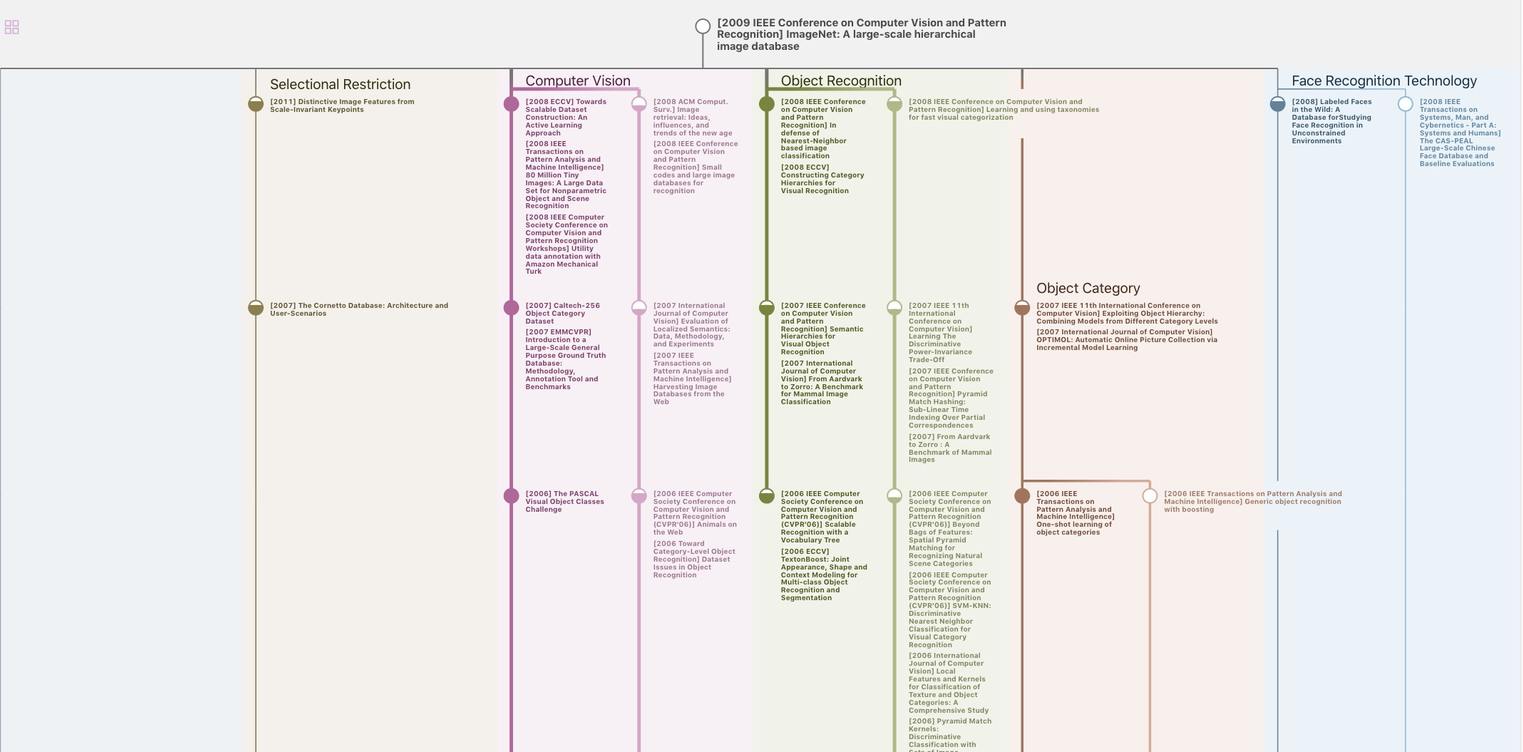
生成溯源树,研究论文发展脉络
Chat Paper
正在生成论文摘要