Evaluating Prediction Models of Sleep Apnea From Smartphone-Recorded Sleep Breathing Sounds
JAMA OTOLARYNGOLOGY-HEAD & NECK SURGERY(2022)
摘要
IMPORTANCE Breathing sounds during sleep are an important characteristic feature of obstructive sleep apnea (OSA) and have been regarded as a potential biomarker. Breathing sounds during sleep can be easily recorded using a microphone, which is found in most smartphone devices. Therefore, it may be easy to implement an evaluation tool for prescreening purposes. OBJECTIVE To evaluate OSA prediction models using smartphone-recorded sounds and identify optimal settings with regard to noise processing and sound feature selection. DESIGN, SETTING, AND PARTICIPANTS A cross-sectional study was performed among patients who visited the sleep center of Seoul National University Bundang Hospital for snoring or sleep apnea from August 2015 to August 2019, Audio recordings during sleep were performed using a smartphone during routine, full-night, in-laboratory polysomnography. Using a random forest algorithm, binary classifications were separately conducted for 3 different threshold criteria accordingto an apnea hypopnea index (AHI) threshold of 5, 15, or 30 events/h. Four regression models were created according to noise reduction and feature selection from the input sound to predict actual AHI: (1) noise reduction without feature selection, (2) noise reduction with feature selection, (3) neither noise reduction nor feature selection, and (4) feature selection without noise reduction. Clinical and polysomnographic parameters that may have been associated with errors were assessed. Data were analyzed from September 2019 to September 2020. MAIN OUTCOMES AND MEASURES Accuracy of OSA prediction models. RESULTS A total of 423 patients (mean [SD] age, 48.1 [12.8] years; 356 [84.1%) male) were analyzed. Data were split into training (n = 256 [60.5%)) and test data sets (n = 167 [39.5%]). Accuracies were 88.2%, 82.3%, and 81.7%, and the areas under curve were 0.90, 0.89, and 0.90 for an AHI threshold of 5. 15, and 30 events/h, respectively. In the regression analysis. using recorded sounds that had not been denoised and had only selected attributes resulted in the highest correlation coefficient (r = 0.78; 95% Cl. 0.69-0.88). The AHI (beta = 0.33; 95% CI, 0.24-0.42) and sleep efficiency (beta = -0.20; 95% CI, -0.35 to -0.05) were found to be associated with estimation error. CONCLUSIONS AND RELEVANCE In this cross-sectional study, recorded sleep breathing sounds using a smartphone were used to create reasonably accurate OSA prediction models. Future research should focus on real-life recordings using various smartphone devices.
更多查看译文
AI 理解论文
溯源树
样例
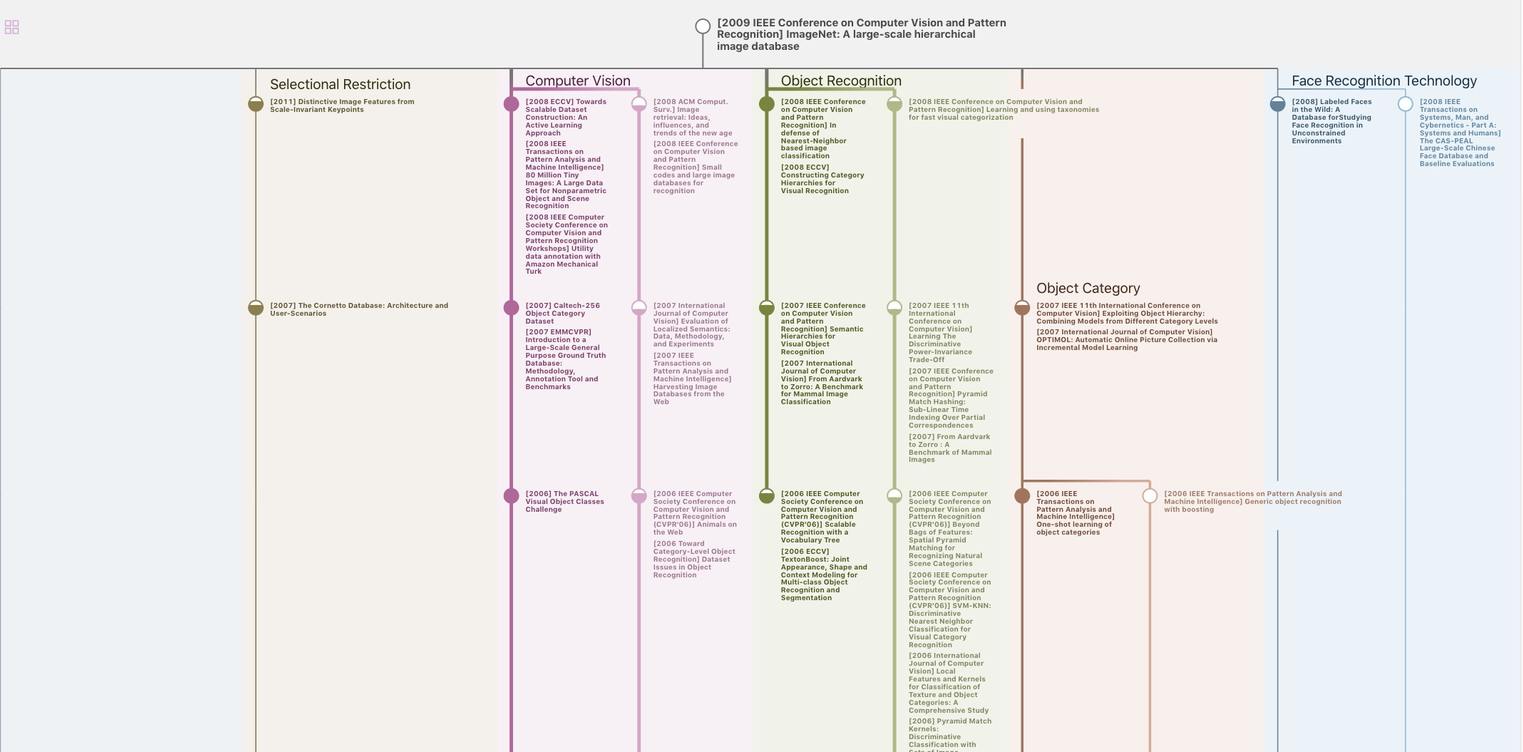
生成溯源树,研究论文发展脉络
Chat Paper
正在生成论文摘要