FedRN: Exploiting k-Reliable Neighbors Towards Robust Federated Learning
Conference on Information and Knowledge Management(2022)
摘要
ABSTRACTRobustness is becoming another important challenge of federated learning in that the data collection process in each client is naturally accompanied by noisy labels. However, it is far more complex and challenging owing to varying levels of data heterogeneity and noise over clients, which exacerbates the client-to-client performance discrepancy. In this work, we propose a robust federated learning method called FedRN, which exploits k-reliable neighbors with high data expertise or similarity. Our method helps mitigate the gap between low- and high-performance clients by training only with a selected set of clean examples, identified by a collaborative model that is built based on the reliability score over clients. We demonstrate the superiority of FedRN via extensive evaluations on three real-world or synthetic benchmark datasets. Compared with existing robust methods, the results show that FedRN significantly improves the test accuracy in the presence of noisy labels.
更多查看译文
关键词
Federated Learning, Robust Learning, Label Noise
AI 理解论文
溯源树
样例
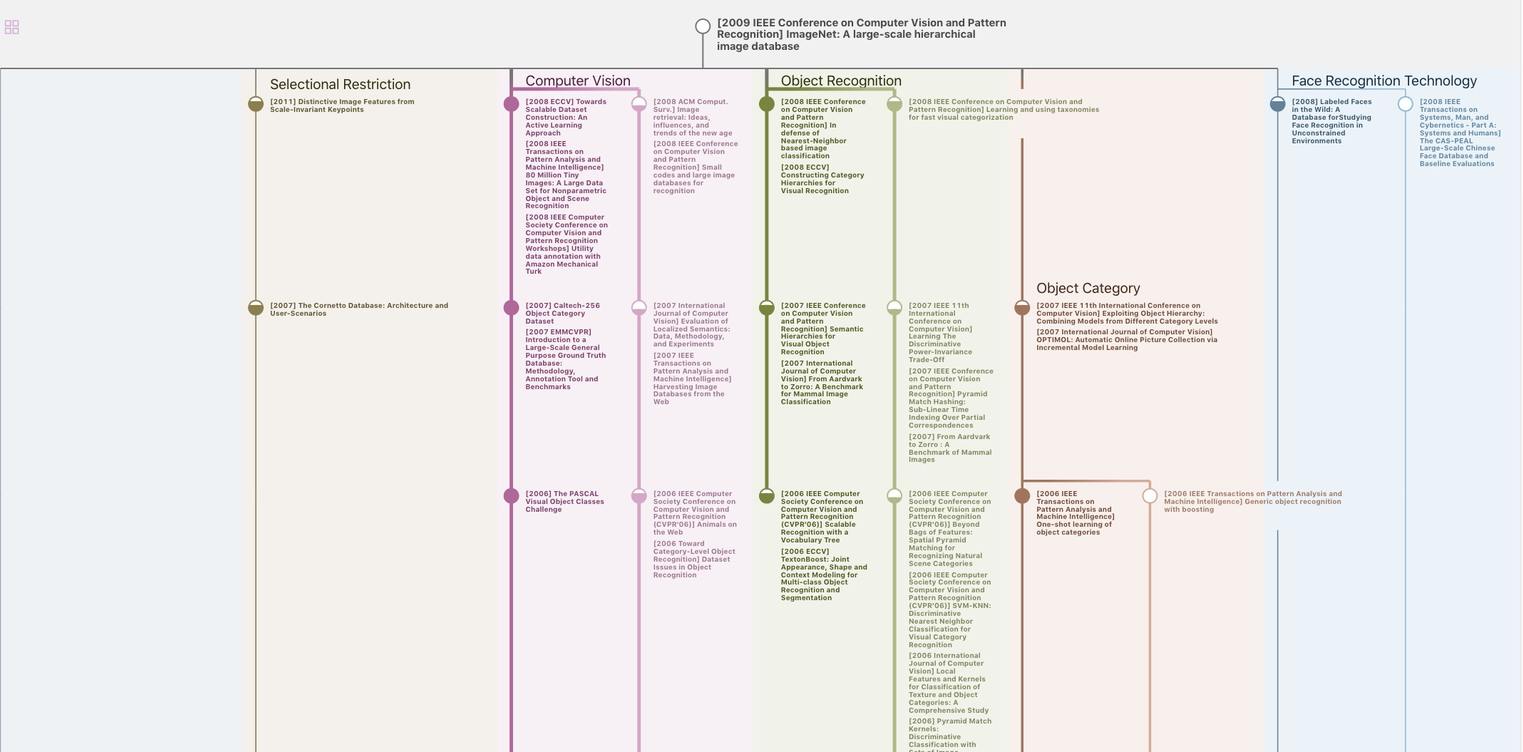
生成溯源树,研究论文发展脉络
Chat Paper
正在生成论文摘要