On the generalization capabilities of FSL methods through domain adaptation: a case study in endoscopic kidney stone image classification
arxiv(2022)
摘要
Deep learning has shown great promise in diverse areas of computer vision, such as image classification, object detection and semantic segmentation, among many others. However, as it has been repeatedly demonstrated, deep learning methods trained on a dataset do not generalize well to datasets from other domains or even to similar datasets, due to data distribution shifts. In this work, we propose the use of a meta-learning based few-shot learning approach to alleviate these problems. In order to demonstrate its efficacy, we use two datasets of kidney stones samples acquired with different endoscopes and different acquisition conditions. The results show how such methods are indeed capable of handling domain-shifts by attaining an accuracy of 74.38% and 88.52% in the 5-way 5-shot and 5-way 20-shot settings respectively. Instead, in the same dataset, traditional Deep Learning (DL) methods attain only an accuracy of 45%.
更多查看译文
关键词
domain adaptation,fsl methods,classification,generalization capabilities
AI 理解论文
溯源树
样例
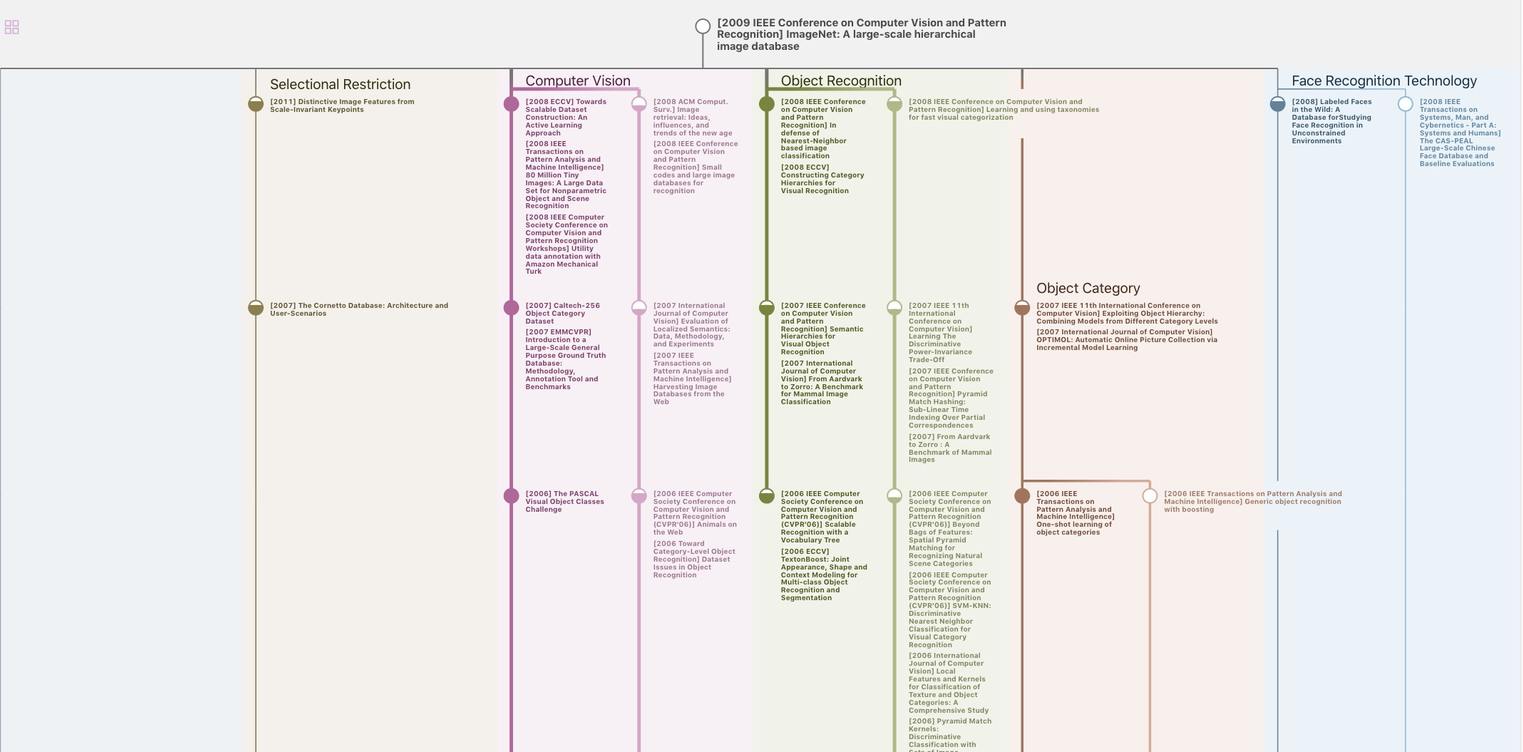
生成溯源树,研究论文发展脉络
Chat Paper
正在生成论文摘要