WeatherBench Probability: A benchmark dataset for probabilistic medium-range weather forecasting along with deep learning baseline models
arxiv(2022)
摘要
WeatherBench is a benchmark dataset for medium-range weather forecasting of geopotential, temperature and precipitation, consisting of preprocessed data, predefined evaluation metrics and a number of baseline models. WeatherBench Probability extends this to probabilistic forecasting by adding a set of established probabilistic verification metrics (continuous ranked probability score, spread-skill ratio and rank histograms) and a state-of-the-art operational baseline using the ECWMF IFS ensemble forecast. In addition, we test three different probabilistic machine learning methods -- Monte Carlo dropout, parametric prediction and categorical prediction, in which the probability distribution is discretized. We find that plain Monte Carlo dropout severely underestimates uncertainty. The parametric and categorical models both produce fairly reliable forecasts of similar quality. The parametric models have fewer degrees of freedom while the categorical model is more flexible when it comes to predicting non-Gaussian distributions. None of the models are able to match the skill of the operational IFS model. We hope that this benchmark will enable other researchers to evaluate their probabilistic approaches.
更多查看译文
关键词
weatherbench,deep learning,benchmark dataset,forecasting,medium-range
AI 理解论文
溯源树
样例
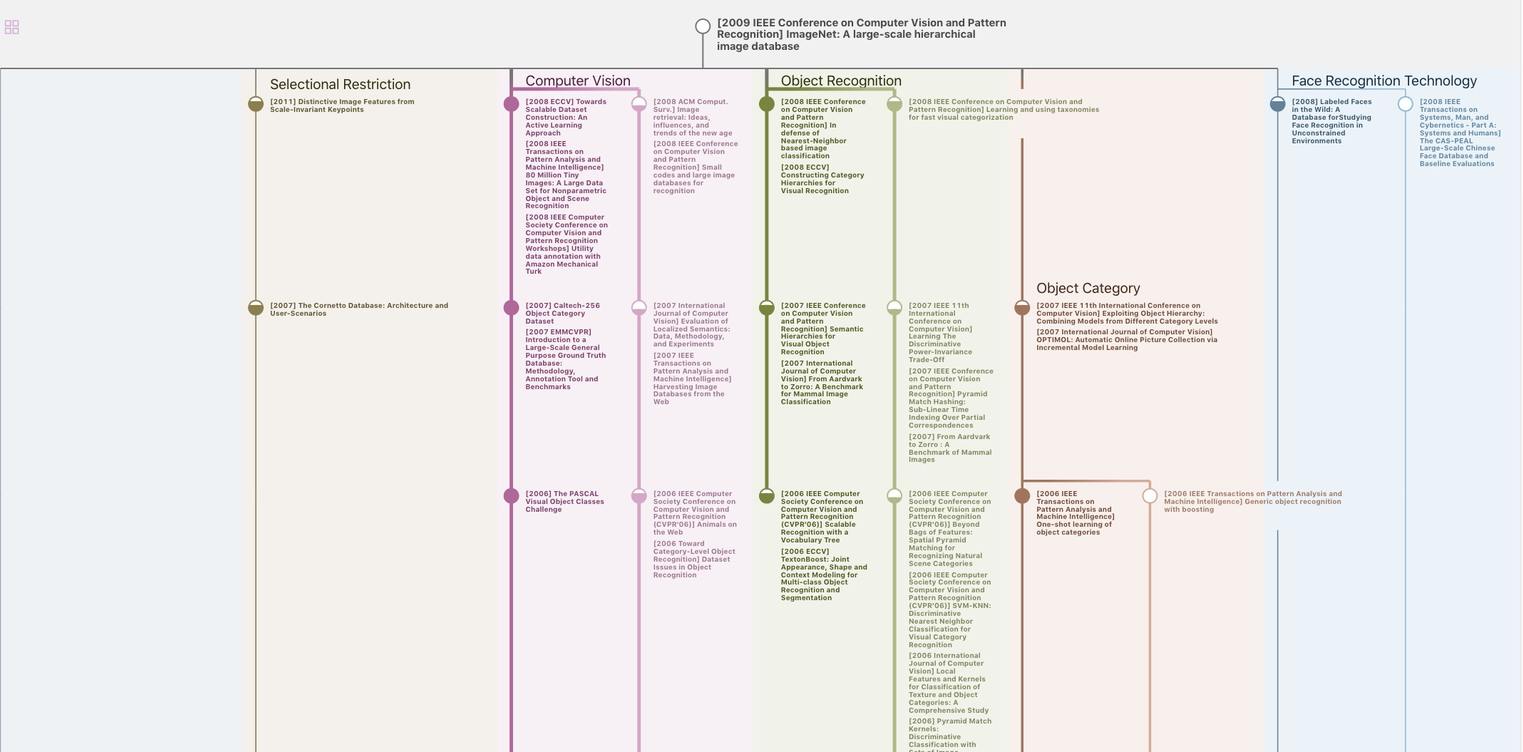
生成溯源树,研究论文发展脉络
Chat Paper
正在生成论文摘要