Bayesian Monitoring of COVID-19 in Sweden
arxiv(2023)
摘要
In an effort to provide regional decision support for the public healthcare, we design a data-driven compartment-based model of COVID-19 in Sweden. From national hospital statistics we derive parameter priors, and we develop linear filtering techniques to drive the simulations given data in the form of daily healthcare demands. We additionally propose a posterior marginal estimator which provides for an improved temporal resolution of the reproduction number estimate as well as supports robustness checks via a parametric bootstrap procedure. From our computational approach we obtain a Bayesian model of predictive value which provides important insight into the progression of the disease, including estimates of the effective reproduction number, the infection fatality rate, and the regional-level immunity. We successfully validate our posterior model against several different sources, including outputs from extensive screening programs. Since our required data in comparison is easy and non-sensitive to collect, we argue that our approach is particularly promising as a tool to support monitoring and decisions within public health.
更多查看译文
关键词
monitoring,sweden
AI 理解论文
溯源树
样例
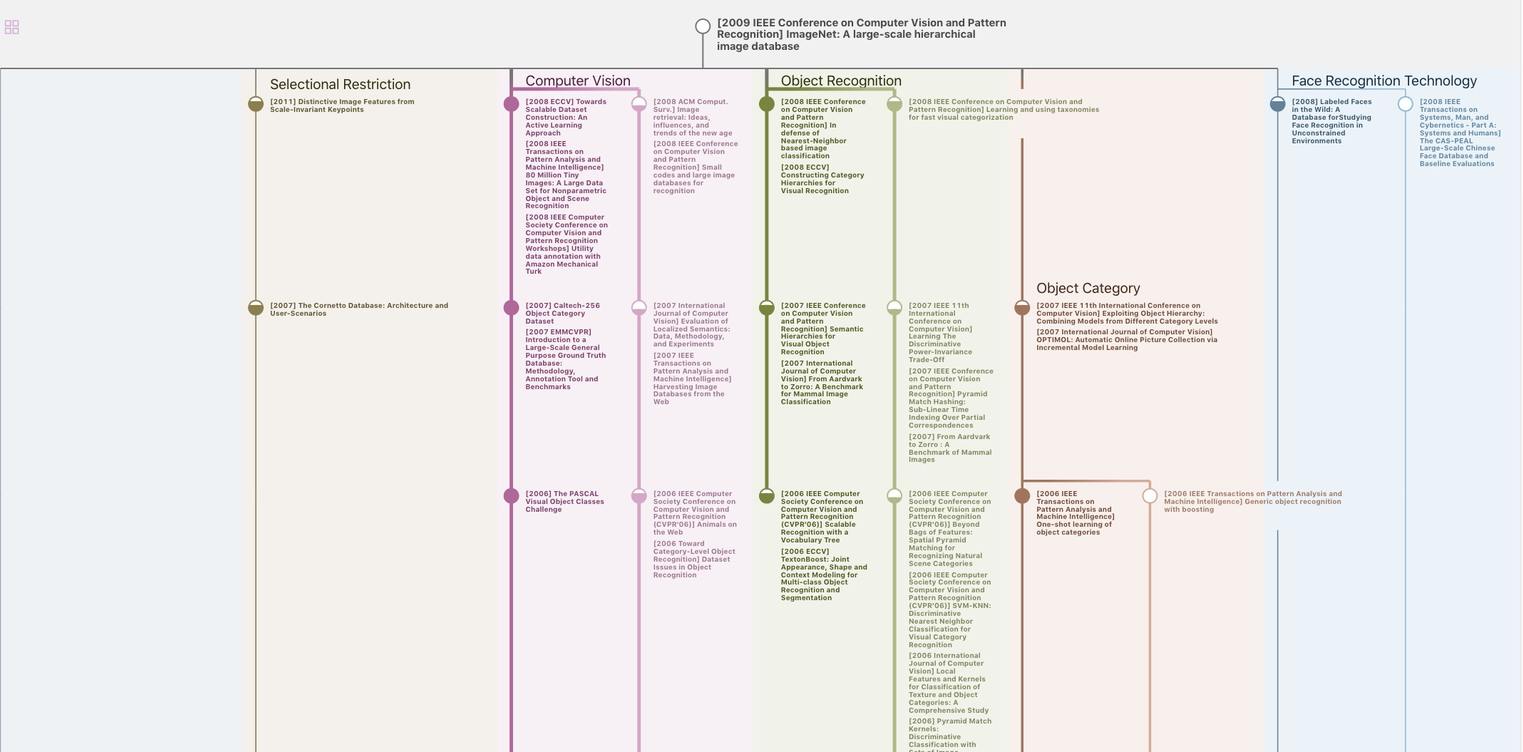
生成溯源树,研究论文发展脉络
Chat Paper
正在生成论文摘要