Exposing Deepfake Face Forgeries With Guided Residuals
IEEE TRANSACTIONS ON MULTIMEDIA(2023)
摘要
For Deepfake detection, residual-based features can preserve tampering traces and suppress irrelevant image content. However, inappropriate residual prediction brings side effects on detection accuracy. Meanwhile, residual-domain features are easily affected by some image operations such as lossy compression. Most existing works exploit either spatial-domain or residual-domain features, which are fed into the backbone network for feature learning. Actually, both types of features are mutually correlated. In this work, we propose an adaptive fusion based guided residuals network (AdapGRnet), which fuses spatial-domain and residual-domain features in a mutually reinforcing way, for Deepfake detection. Specifically, we present a fine-grained manipulation trace extractor (MTE), which is a key module of AdapGRnet. Compared with the prediction-based residuals, MTE can avoid the potential bias caused by inappropriate prediction. Moreover, an attention fusion mechanism (AFM) is designed to selectively emphasize feature channel maps and adaptively allocate the weights for two streams. Experimental results show that AdapGRnet achieves better detection accuracies than the state-of-the-art works on four public fake face datasets including HFF, FaceForensics++, DFDC and CelebDF. Especially, AdapGRnet achieves an accuracy up to 96.52% on the HFF-JP60 dataset, which improves about 5.50%. That is, AdapGRnet achieves better robustness than the existing works.
更多查看译文
关键词
Faces,Feature extraction,Deepfakes,Forgery,Robustness,Image forensics,Biology,Deepfake detection,image forensics,guided residuals,attention fusion mechanism
AI 理解论文
溯源树
样例
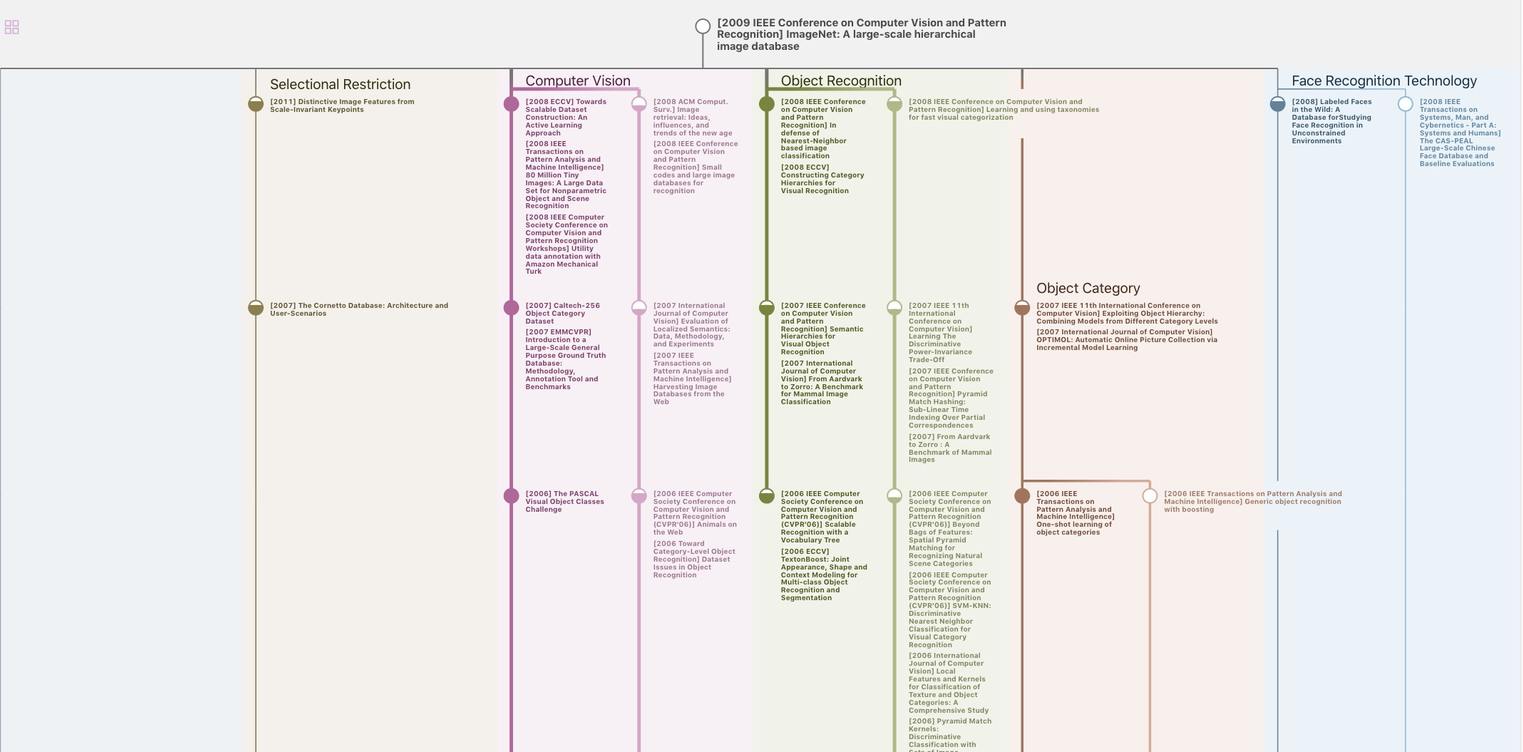
生成溯源树,研究论文发展脉络
Chat Paper
正在生成论文摘要