Contrastive Learning for Improving ASR Robustness in Spoken Language Understanding
Conference of the International Speech Communication Association (INTERSPEECH)(2022)
摘要
Spoken language understanding (SLU) is an essential task for machines to understand human speech for better interactions. However, errors from the automatic speech recognizer (ASR) usually hurt the understanding performance. In reality, ASR systems may not be easy to adjust for the target scenarios. Therefore, this paper focuses on learning utterance representations that are robust to ASR errors using a contrastive objective, and further strengthens the generalization ability by combining supervised contrastive learning and self-distillation in model fine-tuning. Experiments on three benchmark datasets demonstrate the effectiveness of our proposed approach.
更多查看译文
关键词
asr robustness,contrastive learning,language
AI 理解论文
溯源树
样例
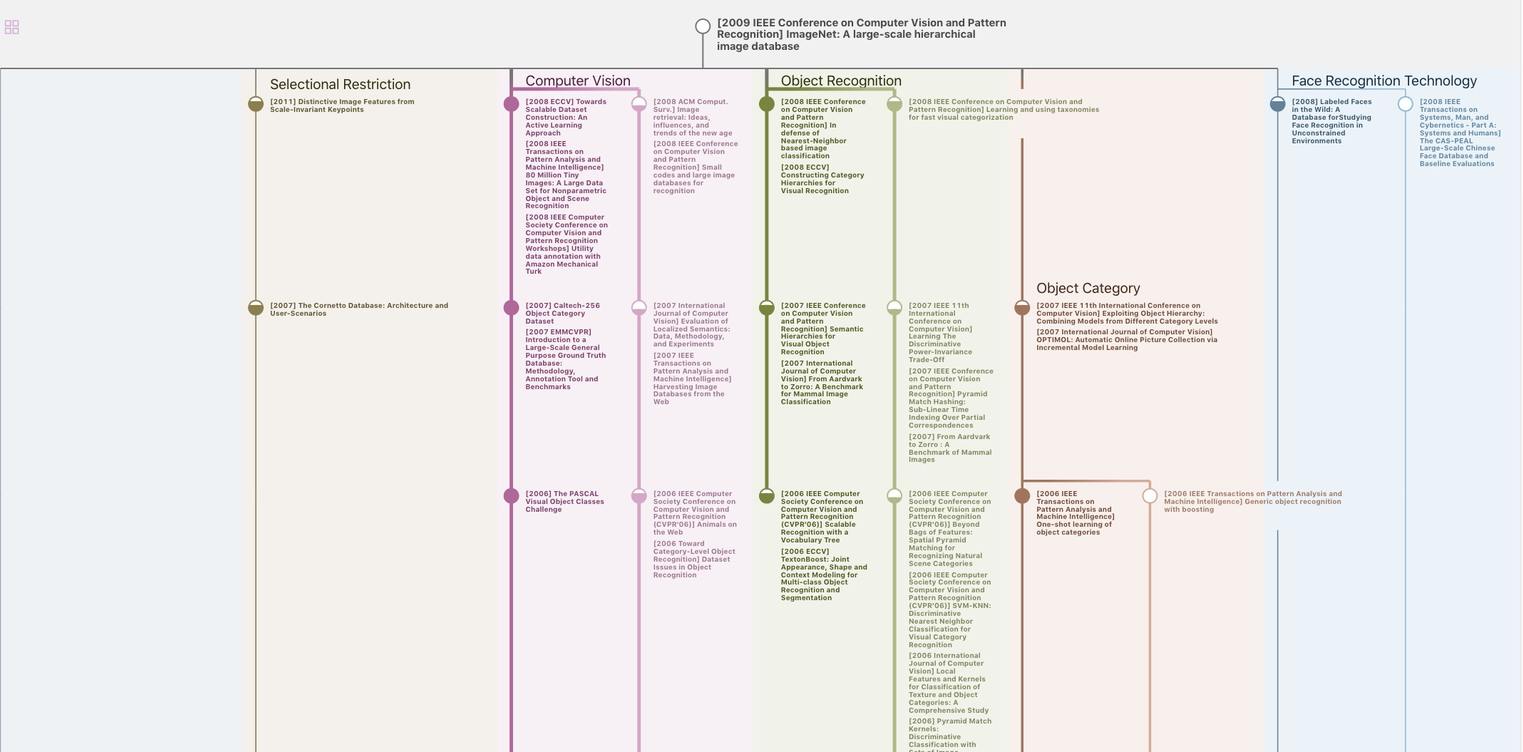
生成溯源树,研究论文发展脉络
Chat Paper
正在生成论文摘要