RADNet: A Deep Neural Network Model for Robust Perception in Moving Autonomous Systems
arxiv(2022)
摘要
Interactive autonomous applications require robustness of the perception engine to artifacts in unconstrained videos. In this paper, we examine the effect of camera motion on the task of action detection. We develop a novel ranking method to rank videos based on the degree of global camera motion. For the high ranking camera videos we show that the accuracy of action detection is decreased. We propose an action detection pipeline that is robust to the camera motion effect and verify it empirically. Specifically, we do actor feature alignment across frames and couple global scene features with local actor-specific features. We do feature alignment using a novel formulation of the Spatio-temporal Sampling Network (STSN) but with multi-scale offset prediction and refinement using a pyramid structure. We also propose a novel input dependent weighted averaging strategy for fusing local and global features. We show the applicability of our network on our dataset of moving camera videos with high camera motion (MOVE dataset) with a 4.1% increase in frame mAP and 17% increase in video mAP.
更多查看译文
关键词
robust perception,deep neural network model,neural network,moving
AI 理解论文
溯源树
样例
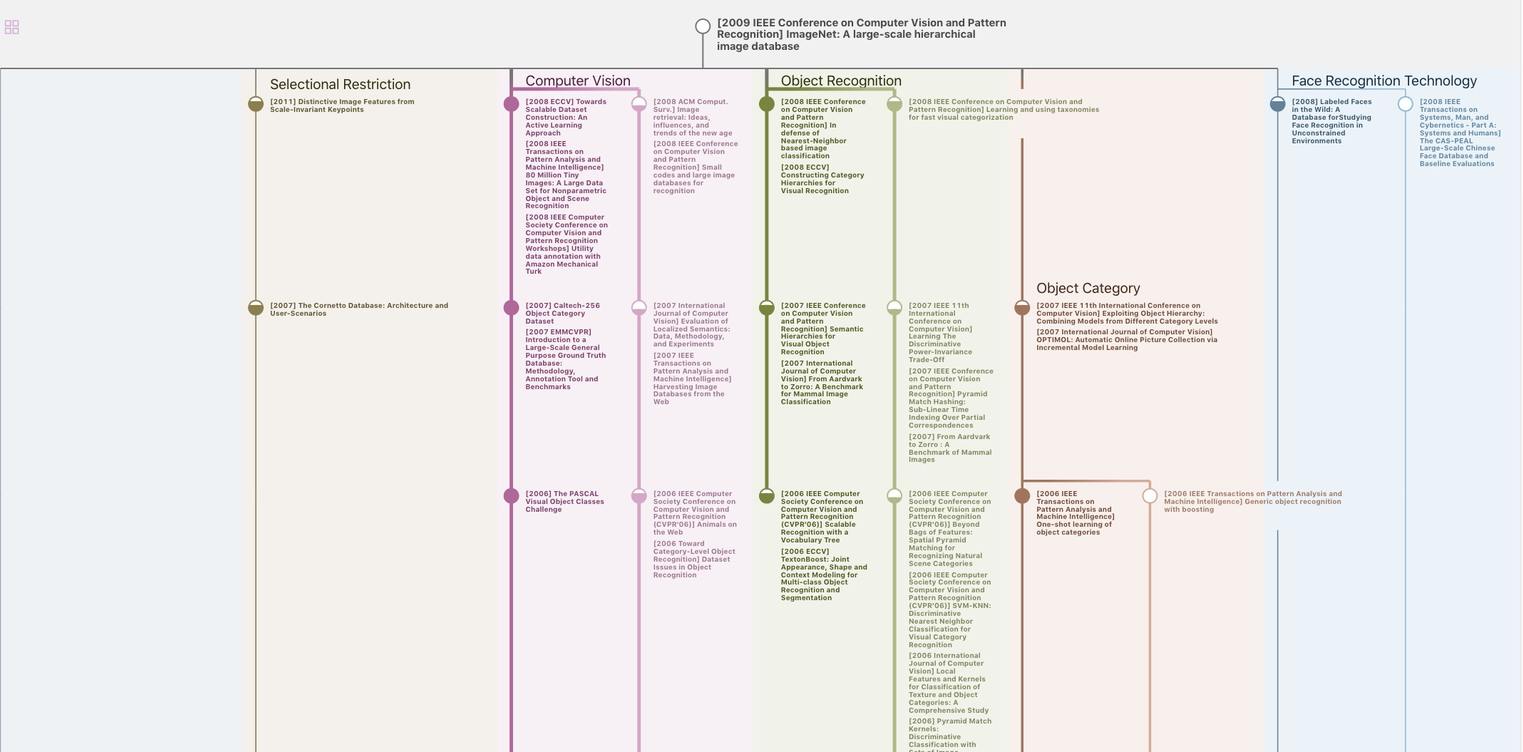
生成溯源树,研究论文发展脉络
Chat Paper
正在生成论文摘要