To Know by the Company Words Keep and What Else Lies in the Vicinity
arxiv(2022)
摘要
The development of state-of-the-art (SOTA) Natural Language Processing (NLP) systems has steadily been establishing new techniques to absorb the statistics of linguistic data. These techniques often trace well-known constructs from traditional theories, and we study these connections to close gaps around key NLP methods as a means to orient future work. For this, we introduce an analytic model of the statistics learned by seminal algorithms (including GloVe and Word2Vec), and derive insights for systems that use these algorithms and the statistics of co-occurrence, in general. In this work, we derive -- to the best of our knowledge -- the first known solution to Word2Vec's softmax-optimized, skip-gram algorithm. This result presents exciting potential for future development as a direct solution to a deep learning (DL) language model's (LM's) matrix factorization. However, we use the solution to demonstrate a seemingly-universal existence of a property that word vectors exhibit and which allows for the prophylactic discernment of biases in data -- prior to their absorption by DL models. To qualify our work, we conduct an analysis of independence, i.e., on the density of statistical dependencies in co-occurrence models, which in turn renders insights on the distributional hypothesis' partial fulfillment by co-occurrence statistics.
更多查看译文
关键词
company words,vicinity
AI 理解论文
溯源树
样例
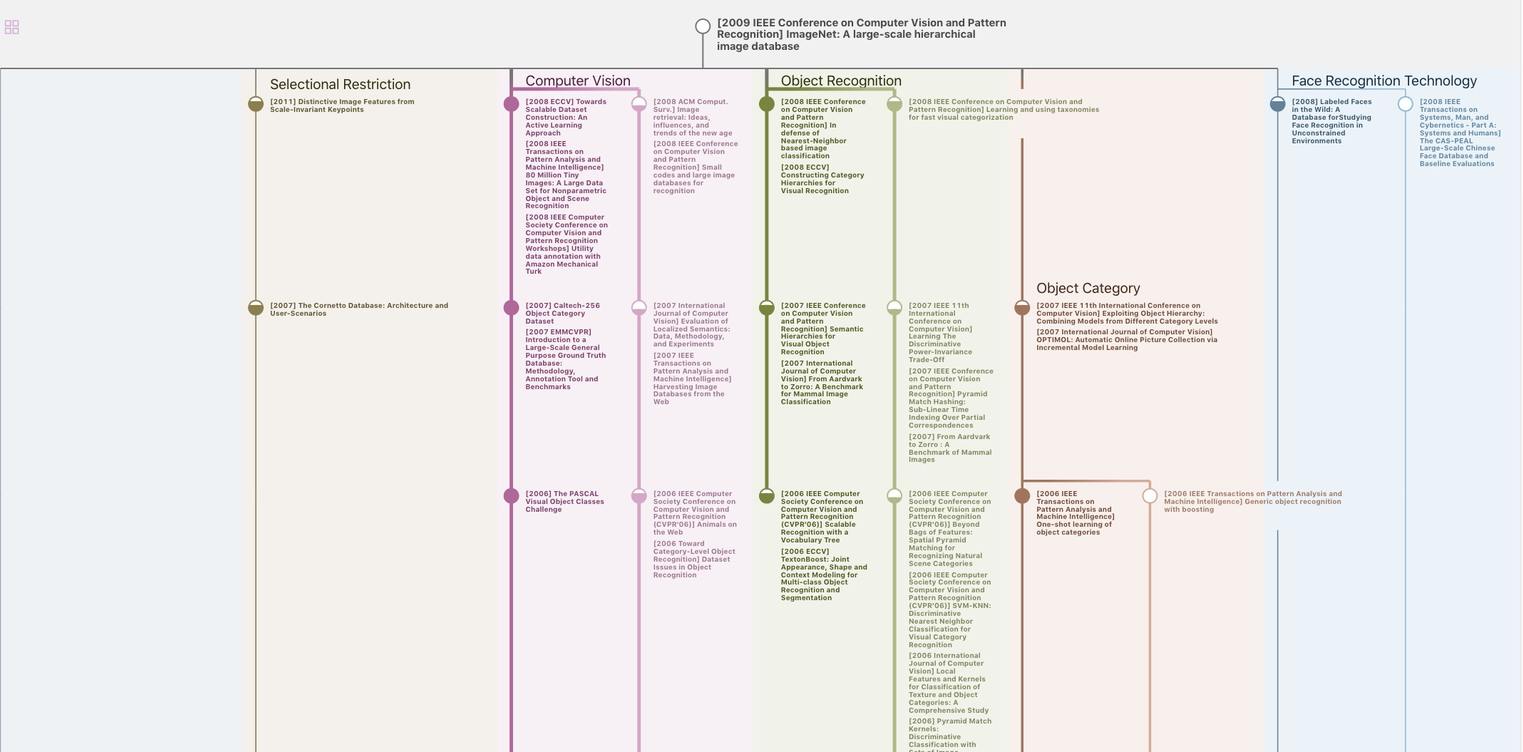
生成溯源树,研究论文发展脉络
Chat Paper
正在生成论文摘要