Preoperative Brain Tumor Imaging: Models and Software for Segmentation and Standardized Reporting
FRONTIERS IN NEUROLOGY(2022)
摘要
For patients suffering from brain tumor, prognosis estimation and treatment decisions are made by a multidisciplinary team based on a set of preoperative MR scans. Currently, the lack of standardized and automatic methods for tumor detection and generation of clinical reports, incorporating a wide range of tumor characteristics, represents a major hurdle. In this study, we investigate the most occurring brain tumor types: glioblastomas, lower grade gliomas, meningiomas, and metastases, through four cohorts of up to 4,000 patients. Tumor segmentation models were trained using the AGU-Net architecture with different preprocessing steps and protocols. Segmentation performances were assessed in-depth using a wide-range of voxel and patient-wise metrics covering volume, distance, and probabilistic aspects. Finally, two software solutions have been developed, enabling an easy use of the trained models and standardized generation of clinical reports: Raidionics and Raidionics-Slicer. Segmentation performances were quite homogeneous across the four different brain tumor types, with an average true positive Dice ranging between 80 and 90%, patient-wise recall between 88 and 98%, and patient-wise precision around 95%. In conjunction to Dice, the identified most relevant other metrics were the relative absolute volume difference, the variation of information, and the Hausdorff, Mahalanobis, and object average symmetric surface distances. With our Raidionics software, running on a desktop computer with CPU support, tumor segmentation can be performed in 16-54 s depending on the dimensions of the MRI volume. For the generation of a standardized clinical report, including the tumor segmentation and features computation, 5-15 min are necessary. All trained models have been made open-access together with the source code for both software solutions and validation metrics computation. In the future, a method to convert results from a set of metrics into a final single score would be highly desirable for easier ranking across trained models. In addition, an automatic classification of the brain tumor type would be necessary to replace manual user input. Finally, the inclusion of post-operative segmentation in both software solutions will be key for generating complete post-operative standardized clinical reports.
更多查看译文
关键词
metastasis, meningioma, glioma, RADS, MRI, deep learning, 3D segmentation, open-source software
AI 理解论文
溯源树
样例
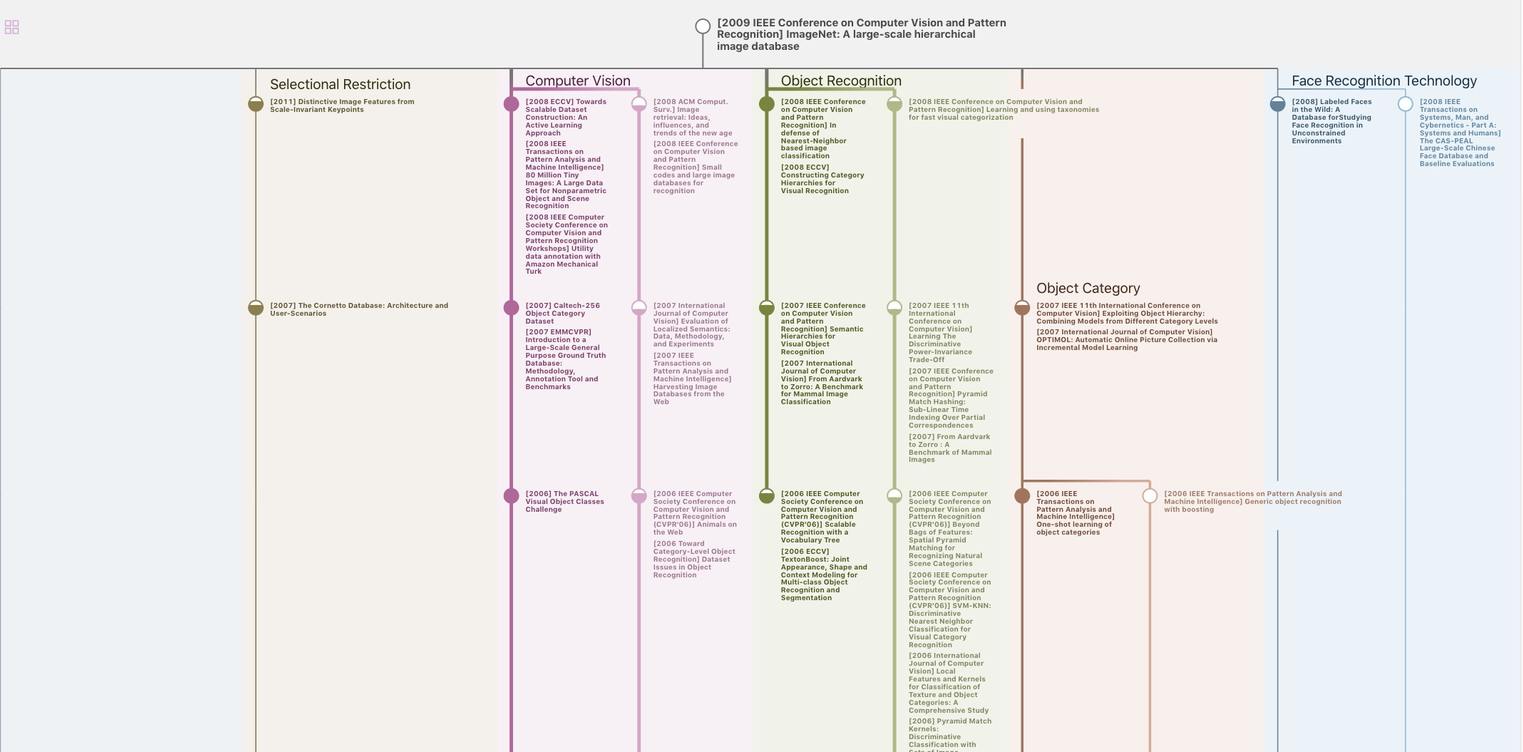
生成溯源树,研究论文发展脉络
Chat Paper
正在生成论文摘要