Learning high-DOF reaching-and-grasping via dynamic representation of gripper-object interaction
ACM Transactions on Graphics(2022)
摘要
We approach the problem of high-DOF reaching-and-grasping via learning joint planning of grasp and motion with deep reinforcement learning. To resolve the sample efficiency issue in learning the high-dimensional and complex control of dexterous grasping, we propose an effective representation of grasping state characterizing the spatial interaction between the gripper and the target object. To represent gripper-object interaction, we adopt Interaction Bisector Surface (IBS) which is the Voronoi diagram between two close by 3D geometric objects and has been successfully applied in characterizing spatial relations between 3D objects. We found that IBS is surprisingly effective as a state representation since it well informs the finegrained control of each finger with spatial relation against the target object. This novel grasp representation, together with several technical contributions including a fast IBS approximation, a novel vector-based reward and an effective training strategy, facilitate learning a strong control model of high-DOF grasping with good sample efficiency, dynamic adaptability, and cross-category generality. Experiments show that it generates high-quality dexterous grasp for complex shapes with smooth grasping motions. Code and data for this paper are at https://github.com/qijinshe/IBS-Grasping.
更多查看译文
关键词
dynamic representation and planning, vector-based reward, replay buffer, imperfect demonstration
AI 理解论文
溯源树
样例
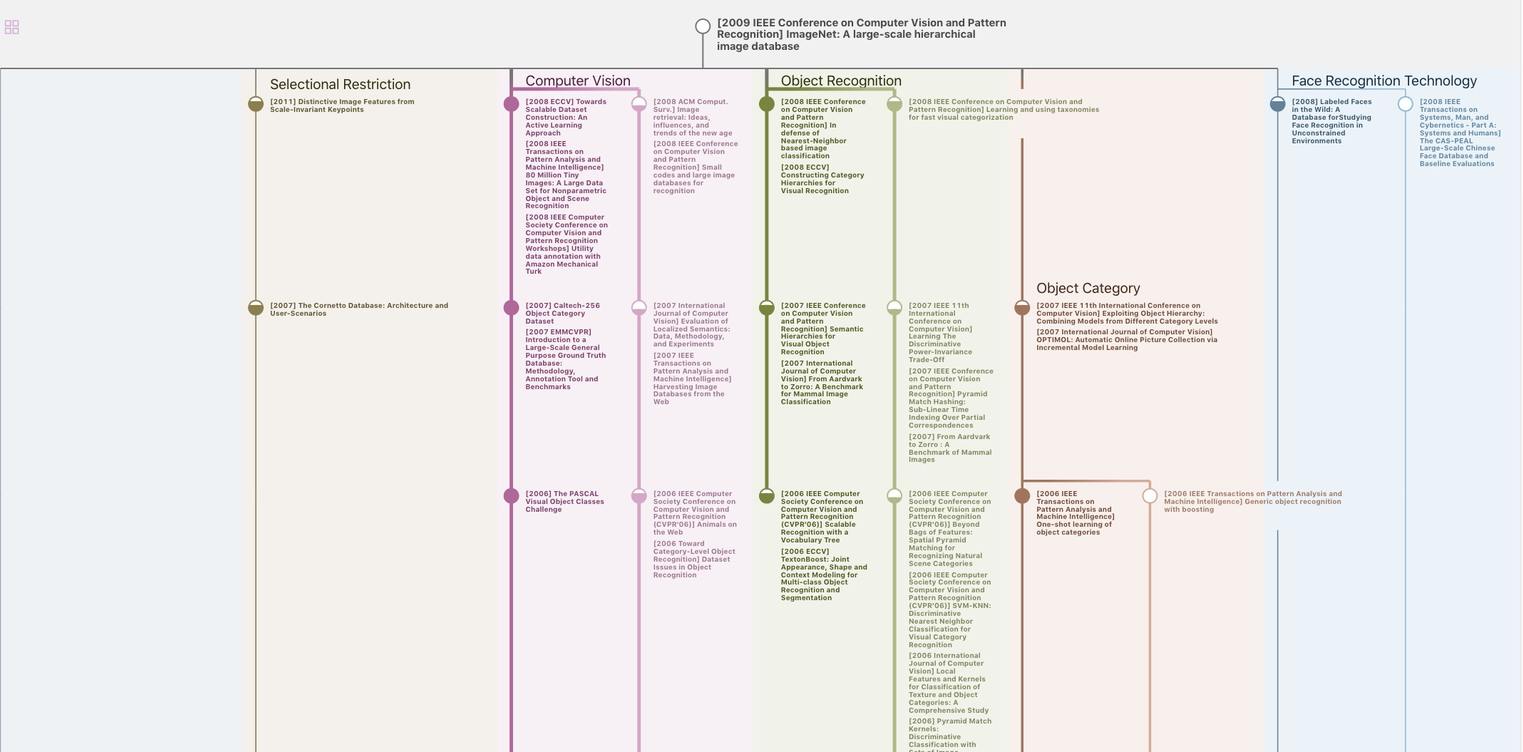
生成溯源树,研究论文发展脉络
Chat Paper
正在生成论文摘要