Tailored Uncertainty Estimation for Deep Learning Systems
arxiv(2022)
摘要
Uncertainty estimation bears the potential to make deep learning (DL) systems more reliable. Standard techniques for uncertainty estimation, however, come along with specific combinations of strengths and weaknesses, e.g., with respect to estimation quality, generalization abilities and computational complexity. To actually harness the potential of uncertainty quantification, estimators are required whose properties closely match the requirements of a given use case. In this work, we propose a framework that, firstly, structures and shapes these requirements, secondly, guides the selection of a suitable uncertainty estimation method and, thirdly, provides strategies to validate this choice and to uncover structural weaknesses. By contributing tailored uncertainty estimation in this sense, our framework helps to foster trustworthy DL systems. Moreover, it anticipates prospective machine learning regulations that require, e.g., in the EU, evidences for the technical appropriateness of machine learning systems. Our framework provides such evidences for system components modeling uncertainty.
更多查看译文
关键词
deep learning,uncertainty estimation
AI 理解论文
溯源树
样例
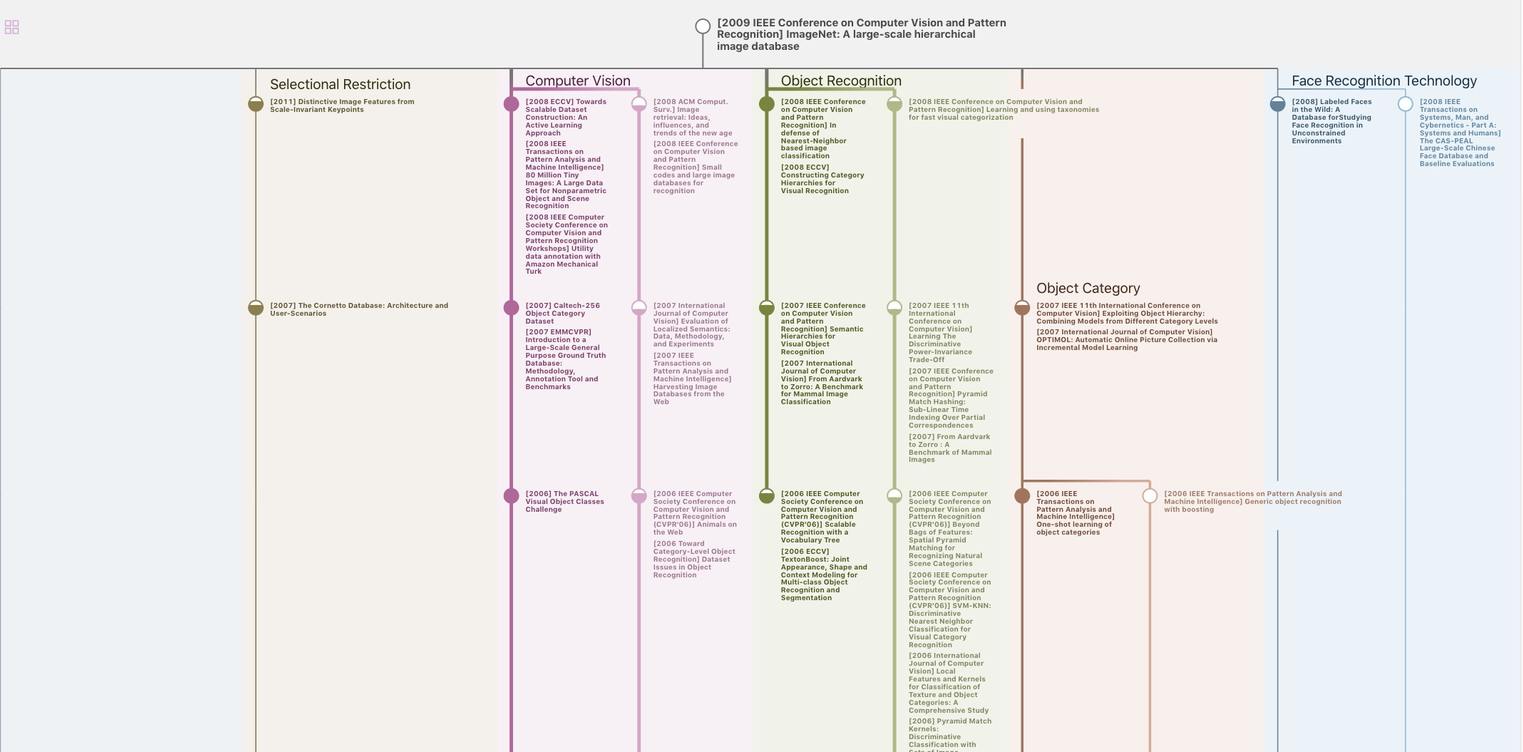
生成溯源树,研究论文发展脉络
Chat Paper
正在生成论文摘要