Sparse-Group Log-Sum Penalized Graphical Model Learning For Time Series.
IEEE International Conference on Acoustics, Speech, and Signal Processing (ICASSP)(2022)
摘要
We consider the problem of inferring the conditional independence graph (CIG) of a high-dimensional stationary multivariate Gaussian time series. A sparse-group lasso based frequency-domain formulation of the problem has been considered in the literature where the objective is to estimate the sparse inverse power spectral density (PSD) of the data. The CIG is then inferred from the estimated inverse PSD. In this paper we investigate use of a sparse-group log-sum penalty (LSP) instead of sparse-group lasso penalty. An alternating direction method of multipliers (ADMM) approach for iterative optimization of the non-convex problem is presented. We provide sufficient conditions for local convergence in the Frobenius norm of the inverse PSD estimators to the true value. This results also yields a rate of convergence. We illustrate our approach using numerical examples utilizing both synthetic and real data.
更多查看译文
关键词
Sparse graph learning,graph estimation,time series,undirected graph,inverse spectral density estimation
AI 理解论文
溯源树
样例
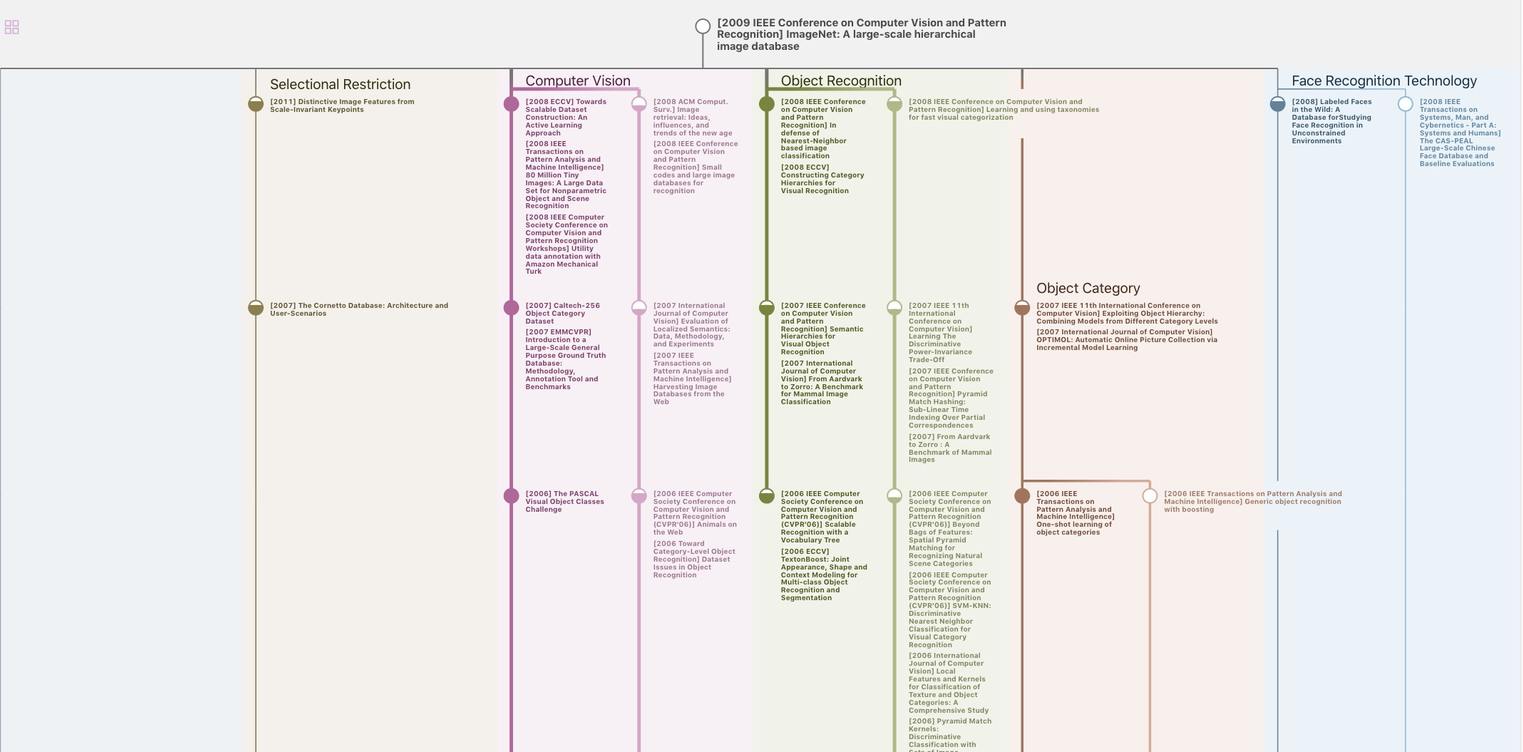
生成溯源树,研究论文发展脉络
Chat Paper
正在生成论文摘要