Inferring ongoing cancer evolution from single tumour biopsies using synthetic supervised learning
PLOS COMPUTATIONAL BIOLOGY(2022)
摘要
Variant allele frequencies (VAF) encode ongoing evolution and subclonal selection in growing tumours. However, existing methods that utilize VAF information for cancer evolutionary inference are compressive, slow, or incorrectly specify the underlying cancer evolutionary dynamics. Here, we provide a proof-of-principle synthetic supervised learning method, TumE, that integrates simulated models of cancer evolution with Bayesian neural networks, to infer ongoing selection in bulk-sequenced single tumour biopsies. Analyses in synthetic and patient tumours show that TumE significantly improves both accuracy and inference time per sample when detecting positive selection, deconvoluting selected subclonal populations, and estimating subclone frequency. Importantly, we show how transfer learning can leverage stored knowledge within TumE models for related evolutionary inference tasks-substantially reducing data and computational time for further model development and providing a library of recyclable deep learning models for the cancer evolution community. This extensible framework provides a foundation and future directions for harnessing progressive computational methods for the benefit of cancer genomics and, in turn, the cancer patient.
更多查看译文
关键词
ongoing cancer evolution,synthetic supervised learning,single tumour biopsies
AI 理解论文
溯源树
样例
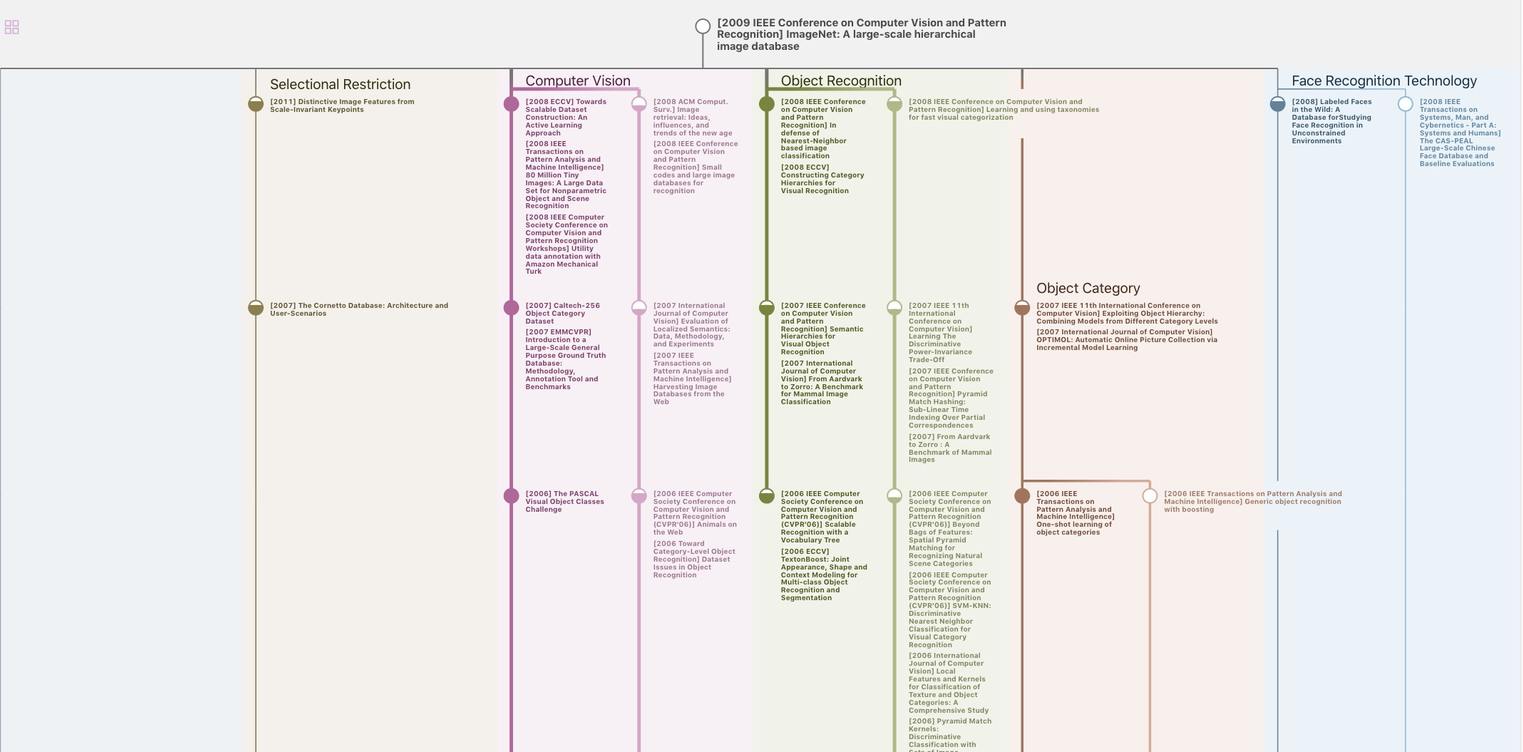
生成溯源树,研究论文发展脉络
Chat Paper
正在生成论文摘要