Mahalanobis balancing: A multivariate perspective on approximate covariate balancing
SCANDINAVIAN JOURNAL OF STATISTICS(2024)
摘要
In the past decade, various exact balancing-based weighting methods were introduced to the causal inference literature. It eliminates covariate imbalance by imposing balancing constraints in a certain optimization problem, which can nevertheless be infeasible when there is bad overlap between the covariate distributions in the treated and control groups or when the covariates are high dimensional. Recently, approximate balancing was proposed as an alternative balancing framework. It resolves the feasibility issue by using inequality moment constraints instead. However, it can be difficult to select the threshold parameters. Moreover, moment constraints may not fully capture the discrepancy of covariate distributions. In this paper, we propose Mahalanobis balancing to approximately balance covariate distributions from a multivariate perspective. We use a quadratic constraint to control overall imbalance with a single threshold parameter, which can be tuned by a simple selection procedure. We show that the dual problem of Mahalanobis balancing is an & ell;2$$ {\ell}_2 $$ norm-based regularized regression problem, and establish interesting connection to propensity score models. We derive asymptotic properties, discuss the high-dimensional scenario, and make extensive numerical comparisons with existing balancing methods.
更多查看译文
关键词
causal inference,Mahalanobis distance,multivariate imbalance,overlap,propensity score
AI 理解论文
溯源树
样例
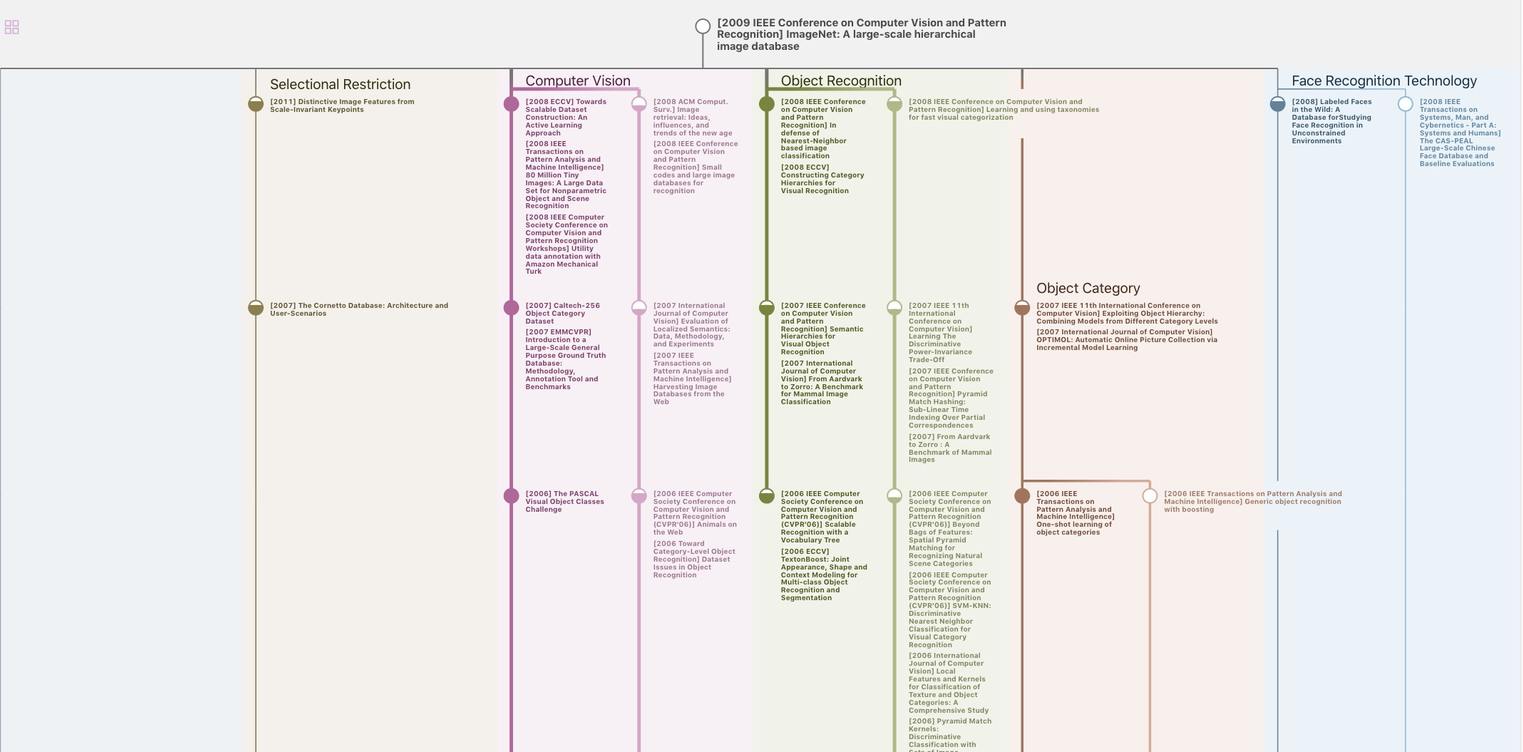
生成溯源树,研究论文发展脉络
Chat Paper
正在生成论文摘要