Data-Driven Adaptive Simultaneous Machine Translation
arxiv(2022)
摘要
In simultaneous translation (SimulMT), the most widely used strategy is the wait-k policy thanks to its simplicity and effectiveness in balancing translation quality and latency. However, wait-k suffers from two major limitations: (a) it is a fixed policy that can not adaptively adjust latency given context, and (b) its training is much slower than full-sentence translation. To alleviate these issues, we propose a novel and efficient training scheme for adaptive SimulMT by augmenting the training corpus with adaptive prefix-to-prefix pairs, while the training complexity remains the same as that of training full-sentence translation models. Experiments on two language pairs show that our method outperforms all strong baselines in terms of translation quality and latency.
更多查看译文
关键词
translation,data-driven
AI 理解论文
溯源树
样例
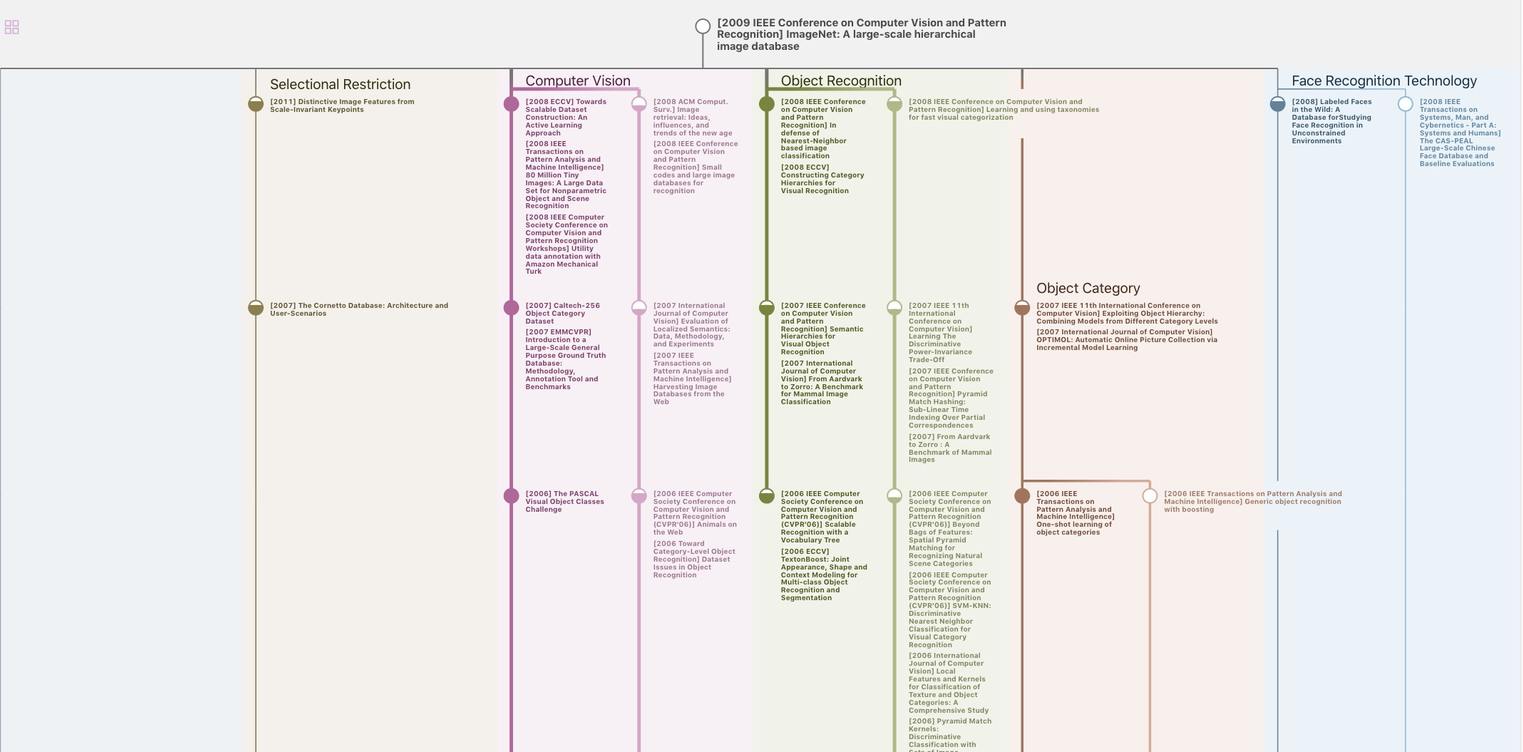
生成溯源树,研究论文发展脉络
Chat Paper
正在生成论文摘要